Summary: Dreams that appear to be simultaneously realistic and bizarre help our brains learn and extract generic concepts from previous experiences, a new study reports.
Source: Human Brain Project
A new study by researchers from the University of Bern, Switzerland suggests that dreams—especially those that simultaneously appear realistic, but, upon a closer look, bizarre—help our brain learn and extract generic concepts from previous experiences.
The study, carried out within the Human Brain Project and published in eLife, offers a new theory on the significance of dreams using machine learning-inspired methodology and brain simulation.
The importance of sleep and dreams for learning and memory has long been recognized—the impact that a single restless night can have on our cognition is well known. “What we lack is a theory that ties this together with consolidation of experiences, generalization of concepts and creativity,” explains Nicolas Deperrois, lead author of the study.
During sleep, we commonly experience two types of sleep phases, alternating one after the other: non-REM sleep, when the brain “replays” the sensory stimulus experienced while awake, and REM sleep, when spontaneous bursts of intense brain activity produce vivid dreams.
The researchers used simulations of the brain cortex to model how different sleep phases affect learning. To introduce an element of unusualness in the artificial dreams, they took inspiration from a machine learning technique called Generative Adversarial Networks (GANs).
In GANs, two neural networks compete with each other to generate new data from the same dataset, in this case a series of simple pictures of objects and animals. This operation produces new artificial images which can look superficially realistic to a human observer.
The researchers then simulated the cortex during three distinct states: wakefulness, non-REM sleep, and REM sleep. During wakefulness, the model is exposed to pictures of boats, cars, dogs and other objects. In non-REM sleep, the model replays the sensory inputs with some occlusions.
REM sleep creates new sensory inputs through the GANs, generating twisted but realistic versions and combinations of boats, cars, dogs etc.
To test the performance of the model, a simple classifier evaluates how easily the identity of the object (boat, dog, car etc.) can be read from the cortical representations.
“Non-REM and REM dreams become more realistic as our model learns,” explains Jakob Jordan, senior author and leader of the research team.
“While non-REM dreams resemble waking experiences quite closely, REM dreams tend to creatively combine these experiences.”
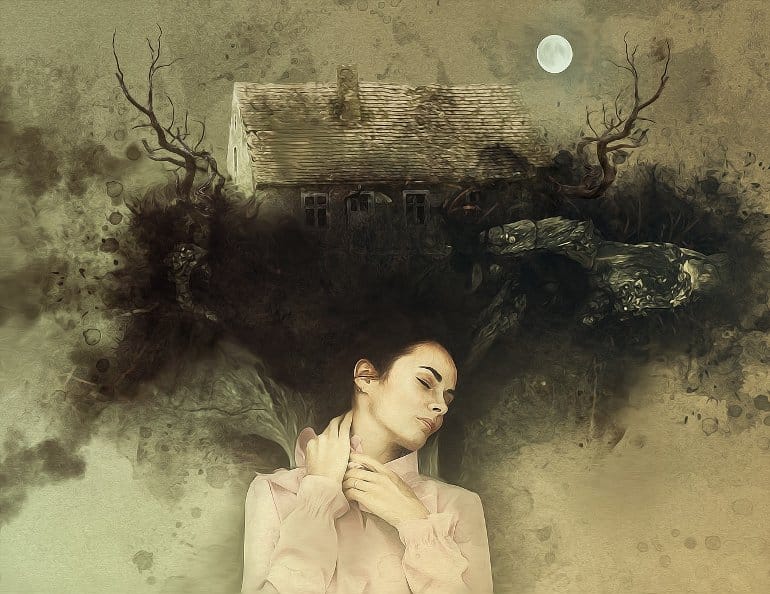
Interestingly, it was when the REM sleep phase was suppressed in the model, or when these dreams were made less creative, that the accuracy of the classifier decreased. When the NREM sleep phase was removed, these representations tended to be more sensitive to sensory perturbations (here, occlusions).
According to this study, wakefulness, non-REM and REM sleep appear to have complementary functions for learning: experiencing the stimulus, solidifying that experience, and discovering semantic concepts. “We think these findings suggest a simple evolutionary role for dreams, without interpreting their exact meaning,” says Deperrois.
“It shouldn’t be surprising that dreams are bizarre: this bizarreness serves a purpose. The next time you’re having crazy dreams, maybe don’t try to find a deeper meaning—your brain may be simply organizing your experiences.”
About this sleep, dreaming, and learning research news
Author: Roberto Inchingolo
Source: Human Brain Project
Contact: Roberto Inchingolo – Human Brain Project
Image: The image is in the public domain
Original Research: Open access.
“Learning cortical representations through perturbed and adversarial dreaming” by Nicolas Deperrois et al. eLife
Abstract
Learning cortical representations through perturbed and adversarial dreaming
Humans and other animals learn to extract general concepts from sensory experience without extensive teaching. This ability is thought to be facilitated by offline states like sleep where previous experiences are systemically replayed. However, the characteristic creative nature of dreams suggests that learning semantic representations may go beyond merely replaying previous experiences.
We support this hypothesis by implementing a cortical architecture inspired by generative adversarial networks (GANs).
Learning in our model is organized across three different global brain states mimicking wakefulness, non-rapid eye movement (NREM), and REM sleep, optimizing different, but complementary, objective functions.
We train the model on standard datasets of natural images and evaluate the quality of the learned representations.
Our results suggest that generating new, virtual sensory inputs via adversarial dreaming during REM sleep is essential for extracting semantic concepts, while replaying episodic memories via perturbed dreaming during NREM sleep improves the robustness of latent representations.
The model provides a new computational perspective on sleep states, memory replay, and dreams, and suggests a cortical implementation of GANs.