Summary: New research reveals that individuals with autism express emotions using the same facial muscles as neurotypical individuals, but at intensities too subtle for the human eye to detect. Using a novel method called micromovement spikes, researchers captured these faint expressions in five-second videos, uncovering emotion where others see none.
This subtlety can lead to misunderstandings, contributing to social disconnect and misperceptions about communication in autistic individuals. The findings may lead to better diagnostic tools and improved communication through AI-powered smartphone apps.
Key Facts:
- Hidden Expressions: Autistic individuals express emotions subtly, with intensities outside the range neurotypicals easily perceive.
- Micromovement Tracking: A new method captures facial microexpressions via brief smartphone videos and advanced data analysis.
- Social Understanding: The research aims to bridge the communication gap between autistic and nonautistic individuals.
Source: Rutgers
A study led by Rutgers University-New Brunswick researchers suggests that tiny facial movements – too slight for the human eye to notice – could help scientists better understand social communication in people with autism.
Published in Frontiers in Psychiatry, the study found that while individuals with autism express emotions like everyone else, their facial expressions may be too subtle for the human eye to detect.
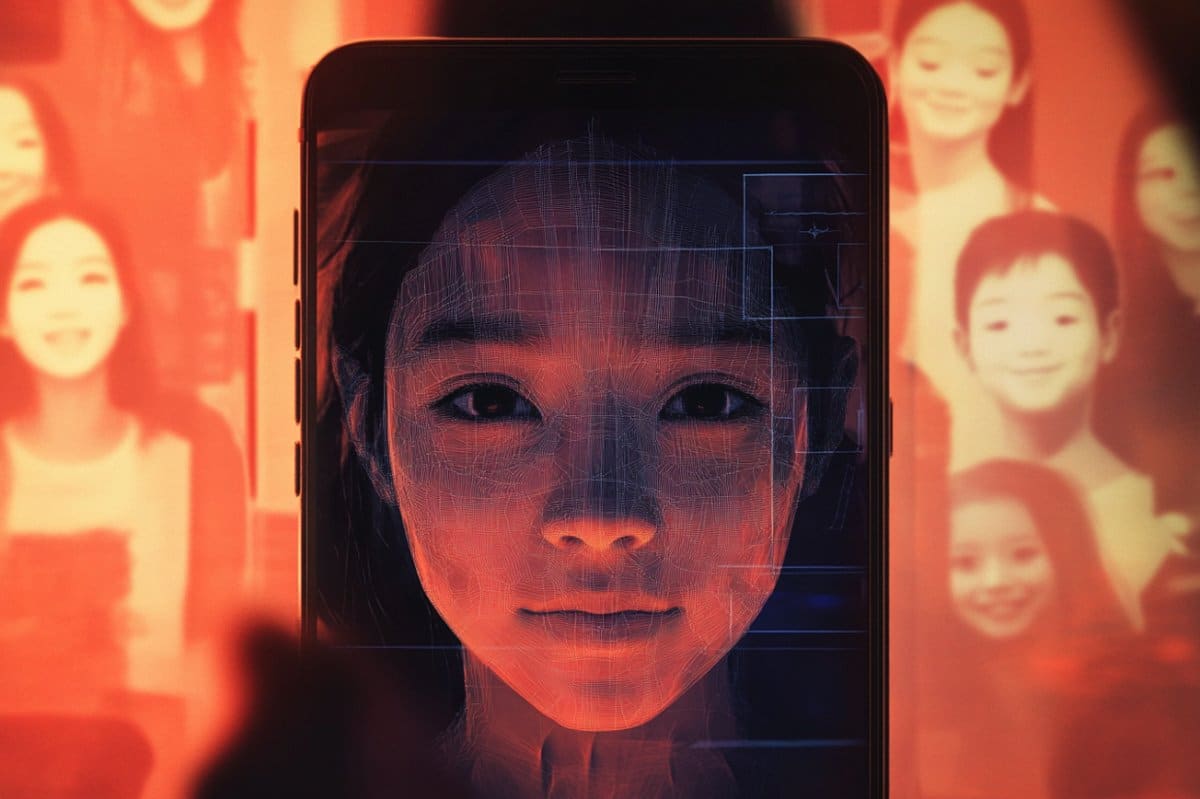
“Autistic individuals use the same basic facial movements to express emotions, but their intensity often falls outside the culturally familiar range that most people recognize,” said Elizabeth Torres, a psychology professor at the Rutgers School of Arts and Sciences.
“This disconnect can lead to missed social cues, causing others to overlook or misinterpret their emotions.”
The researchers said individuals on the autism spectrum – especially those who cannot speak or require significant support for movement – also may have more unpredictable and varied facial expressions, making it more difficult for doctors and caregivers to recognize their emotional cues.
As a result, some may mistakenly assume these individuals aren’t trying to communicate at all.
“But that’s not the case,” said Torres, a computational neuroscientist with more than 17 years of experience working with individuals with autism.
“Their emotions and social signals are there – we just haven’t been able to see them properly. This research could help bridge that gap, fostering a better understanding between autistic and nonautistic individuals.”
This unintentional disconnect can contribute to social isolation and misunderstandings about autistic behavior, she added.
The study, led by Torres and her team at the Rutgers Sensory Motor Integration Lab, used a novel data type she developed called micromovement spikes.
This method captures microscopic facial movements using statistical techniques developed by Torres and nonlinear dynamics methods developed by Theodoros Bermperidis, a postdoctoral associate.
By recording short, five-to-six-second videos on smartphones or tablets, researchers tracked facial micromovements that typically go unnoticed.
“We wanted to investigate whether brief microexpressions appeared during common emotional expressions, like smiling or showing surprise,” Torres said.
“Our goal was to uncover what was really happening beneath the surface when expressions go unnoticed.”
The research team developed an app to guide participants through four stages: practicing video capture, recording a resting face, smiling and making a surprised face. Data was collected in various settings, including schools, therapy gyms and social events, with some participants submitting videos from home.
The study analyzed data from 126 participants, including 55 nonspeaking individuals who communicate by typing. Researchers found that while there were differences in facial micro-movements between autistic and neurotypical individuals – varying by age and sex – the facial muscles responsible for emotional expression were active in both groups.
Torres noted the key difference was in the intensity of these expressions.
“The challenge isn’t a lack of expression – it’s that their intensity falls outside what neurotypical individuals are accustomed to perceiving,” she said.
“This means we are quite literally missing each other’s social cues.”
The implications of this research are far-reaching, said Torres, who also created a mobile application to screen, diagnose and track nervous system disorders. As the chief scientific officer of NeuroInversa LLC, a Rutgers spinoff company co-founded with Chris Dudick, she works on using technology to monitor treatment effectiveness over time.
She said this study challenges common misconceptions about autism and introduces a scalable method for understanding social interactions in autistic individuals.
“This research gives us a powerful tool to expand autism studies beyond simply detecting differences,” Torres said.
“Now, we can work toward bridging the gap—helping neurotypical individuals recognize different expressions of emotion and fostering better social understanding.”
The researchers said their findings could lead to improved diagnostic methods and new ways to support communication between autistic and nonautistic individuals.
By using accessible tools such as smartphone cameras powered by AI, this study paves the way for more inclusive and real-world autism research, Torres added.
Study co-authors included Bermperidis; former and current doctoral students Richa Rai and Joe Vero; and Neel Drain, a student at Robert Wood Johnson Medical School.
Funding: The research was funded by the Nancy Lurie Marks Family Foundation.
About this autism and emotion research news
Author: Megan Schumann
Source: Rutgers
Contact: Megan Schumann – Rutgers
Image: The image is credited to Neuroscience News
Original Research: Open access.
“Hidden social and emotional competencies in autism spectrum disorders captured through the digital lens” by Elizabeth Torres et al. Frontiers in Psychiatry
Abstract
Hidden social and emotional competencies in autism spectrum disorders captured through the digital lens
Background/objectives: The current deficit model of autism leaves us ill-equipped to connect with persons on the spectrum, thus creating disparities and inequalities in all aspects of social exchange in which autistic individuals try to participate.
Traditional research models also tend to follow the clinical definition of impairments in social communication and emotions without offering personalized therapeutic help to autistic individuals. There is a critical need to redefine autism with the aim of co-adapting and connecting with this exponentially growing sector of society.
Here, we hypothesize that there are social and emotional competencies hidden in the movements’ nuances that escape the naked eye. Further, we posit that we can extract such information using highly scalable means such as videos from smartphones.
Methods: Using a phone/tablet app, we recorded brief face videos from 126 individuals (56 on the spectrum of autism) to assess their facial micro-motions during several emotional probes in relation to their resting state.
We extracted the micro-movement spikes (MMSs) from the motion speed along 68 points of the OpenFace grid and empirically determined the continuous family of probability distribution functions best characterizing the MMSs in a maximum likelihood sense.
Further, we analyzed the action units across the face to determine their presence and intensity across the cohort.
Results: We find that the continuous Gamma family of probability distribution functions describes best the empirical face speed variability and offers several parameter spaces to automatically classify participants.
Unambiguous separation at rest denotes marked differences in stochastic patterns between neurotypicals and autistic individuals amenable to further separate autistic individuals according to the required level of support.
Both groups have comparable action units present during emotional probes. They, however, operate within parameter ranges that fall outside our perceptual umwelt and, as such, do not meet our expectations from prior experiences. We cannot detect them.
Conclusions: This work offers new methods to detect hidden facial features and begin the path of augmenting our perception to include those signatures of the autism spectrum that can enhance our capacity for social interactions, communication, and emotional support to meet theirs.