Summary: Using deep learning technology in combination with neuroimaging data, researchers were able to accurately predict who would abstain from cocaine during treatment for addiction.
Source: Yale.
By measuring the strength of connections between different brain networks, Yale researchers successfully predicted who would abstain from cocaine during treatment, they report Jan. 4 in the American Journal of Psychiatry.
The machine-learning technique developed at Yale — called connectome-based predictive modeling (CPM) or “neural fingerprinting” — successfully identified a complex brain network that predicted future abstinence from cocaine during a 12-week treatment program.
“We don’t have a good predictor of who will benefit from a course of treatment,” said Sarah Yip, assistant professor of psychiatry and lead author of the study. “Using this data, we hope to one day be able to tailor treatments to individuals.”
Research led by Yip and Kathleen Carroll, the Albert E. Kent Professor of Psychiatry, analyzed fMRI data from 53 individuals scanned before undergoing treatment for cocaine dependence. They measured the strength of connections between a variety of brain networks of those who successfully abstained from cocaine use and those who did not. Using these neural fingerprints, they then analyzed neuroimaging data from a second group of 45 individuals undergoing treatment, and found that they could correctly predict treatment outcomes with 70% accuracy.
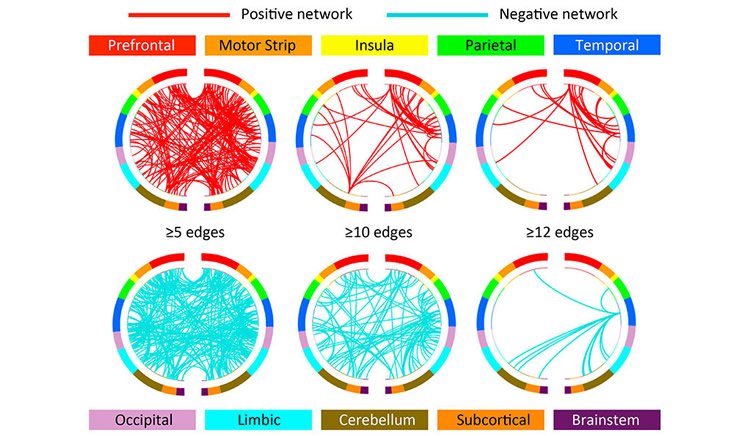
Yip said that measurements of the strength of connections between different brain regions and their correlation to treatment success might also guide scientists in the development of new drugs to aid treatment efforts.
Dustin Scheinost, a co-developer of CPM, and Marc N. Potenza are co-authors of the paper.
Funding: The research was primarily funded by the National Institute on Drug Abuse.
Source: Bill Hathaway – Yale
Publisher: Organized by NeuroscienceNews.com.
Image Source: NeuroscienceNews.com image is credited to Yale.
Original Research: Abstract for “Connectome-Based Prediction of Cocaine Abstinence” by Sarah W. Yip, Ph.D., M.Sc., Dustin Scheinost, Ph.D., Marc N. Potenza, M.D., Ph.D., and Kathleen M. Carroll, Ph.D. American Journal of Psychiatry. Published January 4 2019.
doi:10.1176/appi.ajp.2018.17101147
[cbtabs][cbtab title=”MLA”]Yale”How the Brain Decides Whether to Hold ’em or Fold ’em.” NeuroscienceNews. NeuroscienceNews, 7 January 2019.
<https://neurosciencenews.com/cocaine-abstain-neural-fingerprint-10470/>.[/cbtab][cbtab title=”APA”]Yale(2019, January 7). How the Brain Decides Whether to Hold ’em or Fold ’em. NeuroscienceNews. Retrieved January 7, 2019 from https://neurosciencenews.com/cocaine-abstain-neural-fingerprint-10470/[/cbtab][cbtab title=”Chicago”]Yale”How the Brain Decides Whether to Hold ’em or Fold ’em.” https://neurosciencenews.com/cocaine-abstain-neural-fingerprint-10470/ (accessed January 7, 2019).[/cbtab][/cbtabs]
Abstract
Connectome-Based Prediction of Cocaine Abstinence
Objective:
The authors sought to identify a brain-based predictor of cocaine abstinence by using connectome-based predictive modeling (CPM), a recently developed machine learning approach. CPM is a predictive tool and a method of identifying networks that underlie specific behaviors (“neural fingerprints”).
Methods:
Fifty-three individuals participated in neuroimaging protocols at the start of treatment for cocaine use disorder, and again at the end of 12 weeks of treatment. CPM with leave-one-out cross-validation was conducted to identify pretreatment networks that predicted abstinence (percent cocaine-negative urine samples during treatment). Networks were applied to posttreatment functional MRI data to assess changes over time and ability to predict abstinence during follow-up. The predictive ability of identified networks was then tested in a separate, heterogeneous sample of individuals who underwent scanning before treatment for cocaine use disorder (N=45).
Results:
CPM predicted abstinence during treatment, as indicated by a significant correspondence between predicted and actual abstinence values (r=0.42, df=52). Identified networks included connections within and between canonical networks implicated in cognitive/executive control (frontoparietal, medial frontal) and in reward responsiveness (subcortical, salience, motor/sensory). Connectivity strength did not change with treatment, and strength at posttreatment assessment also significantly predicted abstinence during follow-up (r=0.34, df=39). Network strength in the independent sample predicted treatment response with 64% accuracy by itself and 71% accuracy when combined with baseline cocaine use.
Conclusions:
These data demonstrate that individual differences in large-scale neural networks contribute to variability in treatment outcomes for cocaine use disorder, and they identify specific abstinence networks that may be targeted in novel interventions.