Summary: Researchers have developed a new machine learning algorithm that can autonomously and efficiently identify dendritic spines with 90% accuracy.
Source: Max Planck Florida Institute for Neuroscience.
Is it possible for microscopes to learn a bit about the brain? Even be taught by neuroscientists to reliably recognize parts of brain cells… all on their own? Though it may seem like something straight out of “The Jetsons”, a neuroscientist and software engineer in the lab of MPFI’s Scientific Director, Dr. Ryohei Yasuda, Ph.D., is developing new software with the goal of vastly improving the daily life of a microscope user. Combining a specialized algorithm, aptly named a neural network with a small amount of training, microscopes can now autonomously and efficiently identify small neuronal compartments called dendritic spines with over 90% accuracy. Just like Rosie the robotic maid, microscopes equipped with this Spine Identification software are helping scientists streamline their daily routine, bringing research one step into the future.
In recent years, there has been a rapid expansion and advancement in powerful imaging technologies, capable of taking a look inside the brain with unprecedented resolution and sensitivity. From imaging technology alone, neuroscientists have gained an immense wealth of new information about the brain. But despite many advances in implementation and capabilities, powerful microscopes (and the software running them) are still lacking when it comes to ease of use and overall user experience.
In a new study published in PLOS ONE, Dr. Michael Smirnov, Ph.D., has created an imaging software he hopes will craft a new type of experience for users.
Smirnov explains that “When engineers and scientists design cutting-edge microscopes, they usually focus on the actual physical components and design. They are mostly interested in what these imaging technologies can do, what boundaries they can break and how they perform. Far less attention is placed on how these complex technologies can be made accessible for the average user and really improve their workflow. Each time I write my software, I always think about the user first; how can I make a difference for the person using it, make their research a little easier.”
The Yasuda lab studies the complex process called synaptic plasticity, which is thought to be the cellular basis of learning and memory. When single dendritic spines are stimulated, hundreds of signaling molecules are mobilized to carry new information throughout the neuron. Members of the lab investigate this process in detail utilizing 2-photon microscopy, hoping to glean insights into how the interplay of these molecules translates into memory.
Live 2-photon imaging experiments can be an intensive endeavor. Scientists must patiently sift through a neuron’s dendritic arbor, scanning hundreds of spines for suitable candidates to image. Experiments are frequently repeated to amass sufficient data and those that fail mid-way must be restarted. This often unspoken aspect can quickly turn prolonged imaging into a tedious and time consuming chore, ultimately slowing scientific progress.
Dr. Smirnov’s software aims to rescue neuroscientists from the humdrum of spine imaging. Weaving in an element of machine learning, the algorithm can be taught how to differentiate between dendrite backbone and dendritic spines after being fed a training data set of previously identified spines. Once the training period finishes, the software is capable of automatedly scanning through an image and demarcating spines it comes across with high precision. Unlike previously developed programs which can be computing intensive or optimized only for post imaging analysis, Smirnov’s software is fast, scalable and compatible with most live imaging setups and post analysis applications.
“We incorporated a machine learning approach, because we wanted our software to be flexible, adaptable and as easy to use as possible.” Says Smirnov “The program only requires the user to input an image and specify the scale. After that, the program does the rest.”
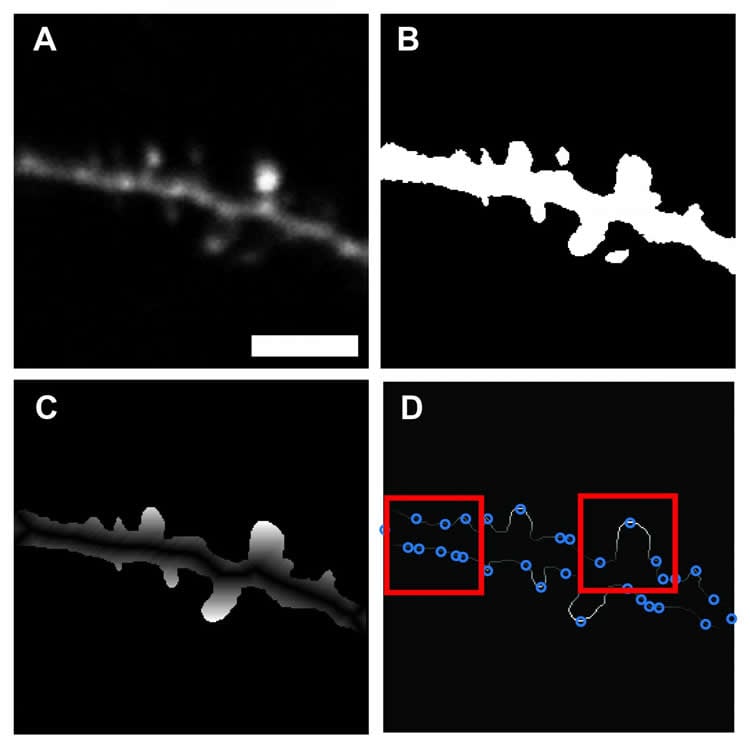
Smirnov describes that by automating the process of spine identification, the software has the potential to dramatically increase experiment workflow, shaving off hours of time. In addition, he has also made his program’s code readily available to the broader neuroscience community. A move he hopes will engage the community of coders working in the field and give them the ability to freely improve upon and customize the software, making it useful for wider applications.
Dr. Smirnov notes that Scientists at MPFI are not only committed to conducting high caliber basic neuroscience research, but also look for ways to continuously improve the research process; fast tracking new discoveries and positively impacting scientists’ daily lives.
Funding: NIH/National Institute of Neurological Disorders and Stroke, Max Planck Florida Institute for Neuroscience and Max Planck Society funded this study.
Source: Helena Decker – Max Planck Florida Institute for Neuroscience
Publisher: Organized by NeuroscienceNews.com.
Image Source: NeuroscienceNews.com image is credited to Max Planck Florida Institute for Neuroscience.
Original Research: Open access research for “An open-source tool for analysis and automatic identification of dendritic spines using machine learning” by Michael S. Smirnov, Tavita R. Garrett, and Ryohei Yasuda in PLOS ONE. Published July 5 2018.
doi:10.1371/journal.pone.0199589
[cbtabs][cbtab title=”MLA”]Max Planck Florida Institute for Neuroscience”New Software Designed for Rapid and Automated Identification of Dendritic Spines.” NeuroscienceNews. NeuroscienceNews, 5 July 2018.
<https://neurosciencenews.com/dendritic-spine-neurotech-9519/>.[/cbtab][cbtab title=”APA”]Max Planck Florida Institute for Neuroscience(2018, July 5). New Software Designed for Rapid and Automated Identification of Dendritic Spines. NeuroscienceNews. Retrieved July 5, 2018 from https://neurosciencenews.com/dendritic-spine-neurotech-9519/[/cbtab][cbtab title=”Chicago”]Max Planck Florida Institute for Neuroscience”New Software Designed for Rapid and Automated Identification of Dendritic Spines.” https://neurosciencenews.com/dendritic-spine-neurotech-9519/ (accessed July 5, 2018).[/cbtab][/cbtabs]
Abstract
An open-source tool for analysis and automatic identification of dendritic spines using machine learning
Synaptic plasticity, the cellular basis for learning and memory, is mediated by a complex biochemical network of signaling proteins. These proteins are compartmentalized in dendritic spines, the tiny, bulbous, post-synaptic structures found on neuronal dendrites. The ability to screen a high number of molecular targets for their effect on dendritic spine structural plasticity will require a high-throughput imaging system capable of stimulating and monitoring hundreds of dendritic spines in various conditions. For this purpose, we present a program capable of automatically identifying dendritic spines in live, fluorescent tissue. Our software relies on a machine learning approach to minimize any need for parameter tuning from the user. Custom thresholding and binarization functions serve to “clean” fluorescent images, and a neural network is trained using features based on the relative shape of the spine perimeter and its corresponding dendritic backbone. Our algorithm is rapid, flexible, has over 90% accuracy in spine detection, and bundled with our user-friendly, open-source, MATLAB-based software package for spine analysis.