Summary: Combining machine learning technology with neuroimaging data, clinicians will be better able to fully analyze a patient’s glioblastoma brain tumor and predict cancer progression.
Source: University of Waterloo
Researchers at the University of Waterloo have created a computational model to predict the growth of deadly brain tumors more accurately.
Glioblastoma multiforme (GBM) is a brain cancer with an average survival rate of only one year. It is difficult to treat due to its extremely dense core, rapid growth, and location in the brain. Estimating these tumors’ diffusivity and proliferation rate is useful for clinicians, but that information is hard to predict for an individual patient quickly and accurately.
Researchers at the University of Waterloo and the University of Toronto have partnered with St. Michael’s Hospital in Toronto to analyze MRI data from multiple GBM sufferers. They’re using machine learning to fully analyze a patient’s tumor, to better predict cancer progression.
Researchers analyzed two sets of MRIs from each of five anonymous patients suffering from GBM. The patients underwent extensive MRIs, waited several months, and then received a second set of MRIs. Because these patients, for undisclosed reasons, chose not to receive any treatment or intervention during this time, their MRIs provided the scientists with a unique opportunity to understand how GBM grows when left unchecked.
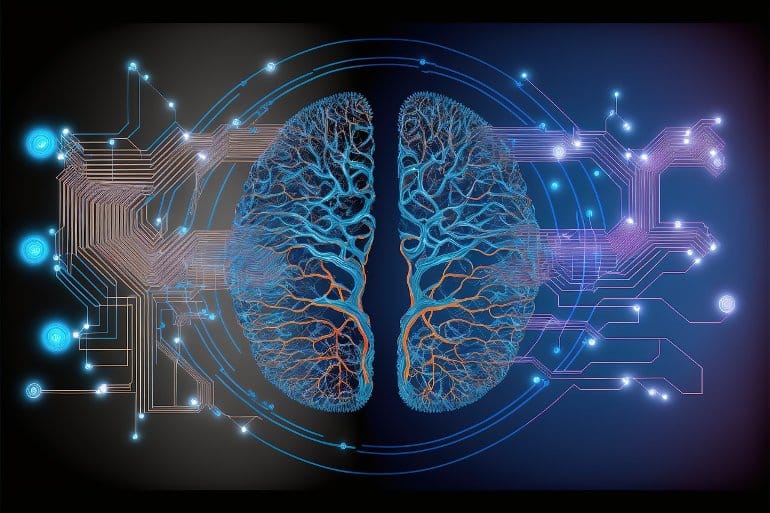
The researchers used a deep learning model to turn the MRI data into patient-specific parameter estimates that inform a predictive model for GBM growth. This technique was applied to patients’ and synthetic tumours, for which the true characteristics were known, enabling them to validate the model.
“We would have loved to do this analysis on a huge data set,” said Cameron Meaney, a PhD candidate in Applied Mathematics and the study’s lead researcher.
“Based on the nature of the illness, however, that’s very challenging because there isn’t a long life expectancy, and people tend to start treatment. That’s why the opportunity to compare five untreated tumours was so rare – and valuable.”
Now that the scientists have a good model of how GBM grows untreated, their next step is to expand the model to include the effect of treatment on the tumours. Then the data set would increase from a handful of MRIs to thousands.
Meaney emphasizes that access to MRI data – and partnership between mathematicians and clinicians – can have huge impacts on patients going forward.
“The integration of quantitative analysis into healthcare is the future,” Meaney said.
About this brain cancer and machine learning research news
Author: Ryon Jones
Source: University of Waterloo
Contact: Ryon Jones – University of Waterloo
Image: The image is in the public domain
Original Research: Open access.
“Deep learning characterization of brain tumours with diffusion weighted imaging” by Cameron Meaney et al. Journal of Theoretical Biology
Abstract
Deep learning characterization of brain tumours with diffusion weighted imaging
Glioblastoma multiforme (GBM) is one of the most deadly forms of cancer. Methods of characterizing these tumours are valuable for improving predictions of their progression and response to treatment.
A mathematical model called the proliferation-invasion (PI) model has been used extensively in the literature to model the growth of these tumours, though it relies on known values of two key parameters: the tumour cell diffusivity and proliferation rate.
Unfortunately, these parameters are difficult to estimate in a patient-specific manner, making personalized tumour forecasting challenging.
In this paper, we develop and apply a deep learning model capable of making accurate estimates of these key GBM-characterizing parameters while simultaneously producing a full prediction of the tumour progression curve.
Our method uses two sets of multi sequence MRI in order to produce estimations and relies on a preprocessing pipeline which includes brain tumour segmentation and conversion to tumour cellularity.
We first apply our deep learning model to synthetic tumours to showcase the model’s capabilities and identify situations where prediction errors are likely to occur. We then apply our model to a clinical dataset consisting of five patients diagnosed with GBM.
For all patients, we derive evidence-based estimates for each of the PI model parameters and predictions for the future progression of the tumour, along with estimates of the parameter uncertainties.
Our work provides a new, easily generalizable method for the estimation of patient-specific tumour parameters, which can be built upon to aid physicians in designing personalized treatments.