Summary: A new study shows that brain connectivity patterns, especially in the dorsal anterior cingulate cortex, can help predict how patients with major depressive disorder (MDD) will respond to antidepressants. By combining brain imaging with clinical data, researchers developed machine learning models that accurately predicted treatment outcomes across two large trials.
Adding brain-based biomarkers significantly improved prediction accuracy over traditional factors like age or symptom severity. This marks a major step toward personalized, faster, and more effective treatment for depression—reducing the frustration of trial-and-error prescribing.
Key Facts:
- Brain-Based Prediction: Connectivity in a key brain region improves antidepressant outcome forecasts.
- Cross-Trial Success: Models trained in one study accurately predicted outcomes in another.
- Toward Personalization: The findings pave the way for tailored depression treatments based on brain scans.
Source: CAMH
Finding the right antidepressant treatment can be a frustrating, time-consuming process — one that often requires individuals to endure weeks of ineffective medication before trying something new.
Now, a new study offers hope for a more personalized approach.
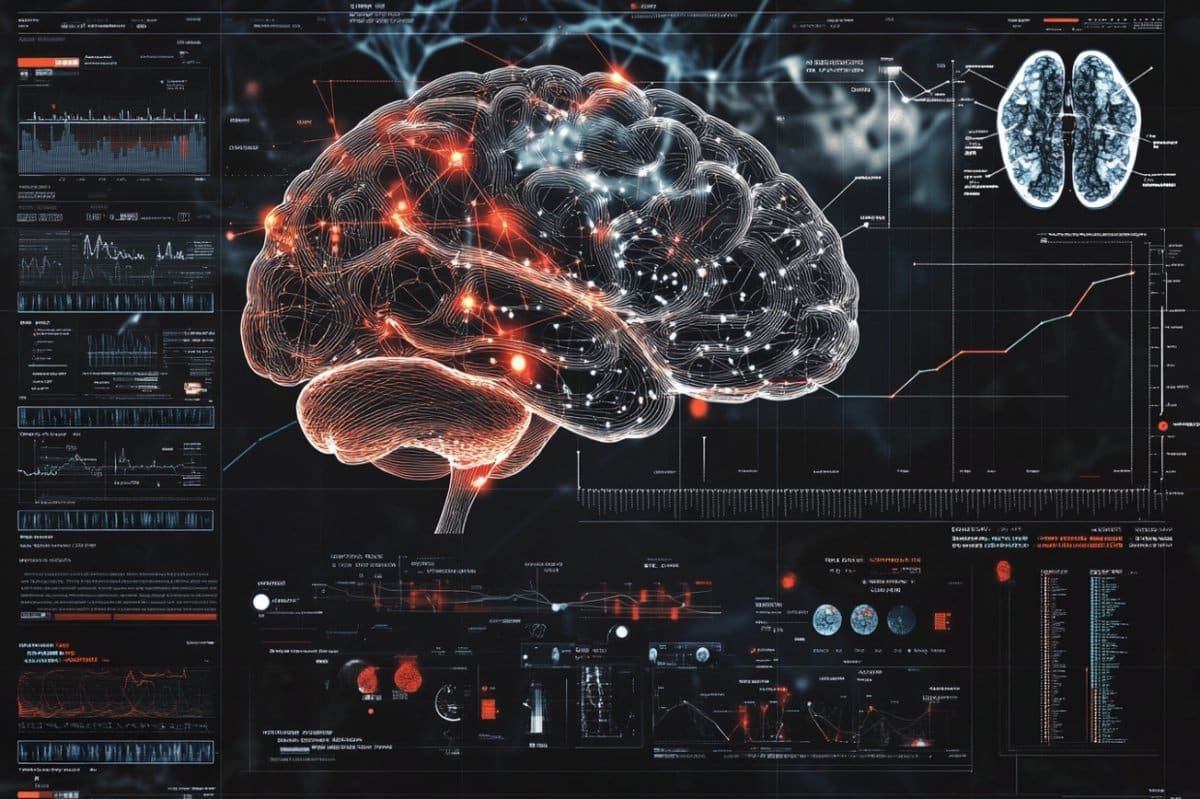
Published in JAMA Network Open, the study reveals promising progress toward predicting how patients with major depressive disorder (MDD) will respond to antidepressant medications using brain imaging and clinical data.
The research demonstrated that brain connectivity patterns — specifically in the dorsal anterior cingulate cortex — could significantly improve predictions of treatment response across two large, independent clinical trials.
“In spite of the availability of several antidepressant treatments, including medications and psychotherapy, many individuals with depression have difficulties finding the treatment that works best for them,” said Diego Pizzagalli, PhD, director of the Noel Drury, M.D. Institute for Translational Depression Discoveries at UC Irvine and Distinguished Professor at the Charlie Dunlop School of Biological Sciences and the School of Medicine.
“As a result, for many, treatment follows a trial-and-error approach. Discovering brain-based markers predicting positive antidepressant response promises to allow a more personalized treatment and thereby speed up reduction of symptoms.”
Using machine learning models trained on clinical and neuroimaging data from more than 350 participants in two international trials — EMBARC in the U.S. and CANBIND-1 in Canada — the researchers evaluated whether their algorithms could reliably predict who would respond to common antidepressants like sertraline and escitalopram.
They found that adding a brain connectivity marker to traditional clinical data (such as age, sex and baseline depression severity) significantly improved prediction performance across both studies.
“We identified a brain connectivity marker that was predictive of response to common antidepressants across two large-scale clinical trials in the U.S. and Canada,” explained Peter Zhukovsky, a former postdoctoral fellow in Dr. Pizzagalli’s laboratory and now Scientist in the Brain Health Imaging Centre at the Centre for Addiction and Mental Health (CAMH) and first author of the study.
“The predictive performance of our algorithm was improved by the addition of the brain connectivity feature to clinical and demographic markers, reaching moderate levels.
“Our findings are promising for the search for biomarkers predicting depression response. We hope these efforts will help connect patients with treatments that are most likely to work for them.”
The study also tackled the often-overlooked challenge of generalizability — whether a prediction model developed in one trial will hold up in a completely separate population. That’s where this research stands out. Models trained on one trial performed surprisingly well when tested on another, highlighting the potential for broader real-world use.
“Data harmonization and building a large-scale database with different treatments is challenging,” noted Zhukovsky.
“However, we’re hopeful that cross-trial analyses such as the one we conducted in this project will advance precision medicine goals.”
The study’s implications are far-reaching. By developing biomarkers that are not limited to one treatment setting or population, researchers are laying the groundwork for clinical tools that could eventually match patients with effective treatments earlier, potentially reducing suffering and speeding recovery.
“We investigated biomarkers predicting antidepressant treatment response,” Zhukovsky added.
“However, many other options are available for treating depression and if we can identify markers for specific treatments, then the resulting decision support tools could be tested in biomarker-guided clinical studies.”
As mental health disorders continue to rise globally, the need for faster, data-driven treatment approaches is more urgent than ever.
The team’s findings underscore the promise of brain-based diagnostics to transform how depression is treated. But they also stress that more research is needed — larger trials, new treatment comparisons and real-world implementation studies — to bring these insights from the lab to the clinic.
This line of work will be one of the key priorities within the recently launched Noel Drury, M.D. Institute for Translational Depression Discoveries at UC Irvine.
Funding: This study was made possible through a multi-institutional collaboration among researchers from McLean Hospital and Harvard Medical School; the University of Texas Southwestern Medical Center; the New York State Psychiatric Institute; Columbia University Vagelos College of Physicians and Surgeons; Stony Brook University; the University of Toronto; and the Centre for Depression and Suicide Studies at Unity Health Toronto.
The EMBARC study was supported by grants from the National Institute of Mental Health (U01MH092221 and U01MH092250).
The CAN-BIND-1 trial was conducted in partnership with, and received financial support from, the Ontario Brain Institute and the Brain-CODE platform, with partial funding from the government of Ontario.
About this depression and psychopharmacology research news
Author: Zachary Caldwell
Source: CAMH
Contact: Zachary Caldwell – CAMH
Image: The image is credited to Neuroscience News
Original Research: Open access.
“Generalizability of Treatment Outcome Prediction Across Antidepressant Treatment Trials in Depression” by Diego Pizzagalli et al. JAMA Network Open
Abstract
Generalizability of Treatment Outcome Prediction Across Antidepressant Treatment Trials in Depression
Importance
Although several predictive models for response to antidepressant treatment have emerged on the basis of individual clinical trials, it is unclear whether such models generalize to different clinical and geographical contexts.
Objective
To assess whether neuroimaging and clinical features predict response to sertraline and escitalopram in patients with major depressive disorder (MDD) across 2 multisite studies using machine learning and to predict change in depression severity in 2 independent studies.
Design, Setting, and Participants
This prognostic study included structural and functional resting-state magnetic resonance imaging and clinical and demographic data from the Establishing Moderators and Biosignatures of Antidepressant Response in Clinical Care (EMBARC) randomized clinical trial (RCT), which administered sertraline (in stage 1 and stage 2) and placebo, and the Canadian Biomarker Integration Network in Depression (CANBIND-1) RCT, which administered escitalopram. EMBARC recruited participants with MDD (aged 18-65 years) at 4 academic sites across the US between August 2011 and December 2015. CANBIND-1 recruited participants with MDD from 6 outpatient centers across Canada between August 2013 and December 2016. Data were analyzed from October 2023 to May 2024.
Main Outcomes and Measures
Prediction performance for treatment response was assessed using balanced classification accuracy and area under the curve (AUC). In secondary analyses, prediction performance was assessed using observed vs predicted correlations between change in depression severity.
Results
In 363 adult patients (225 from EMBARC and 138 from CANBIND-1; mean [SD] age, 36.6 [13.1] years; 235 women [64.7%]), the best-performing models using pretreatment clinical features and functional connectivity of the dorsal anterior cingulate had moderate cross-trial generalizability for antidepressant treatment (trained on CANBIND-1 and tested on EMBARC, AUC = 0.62 for stage 1 and AUC = 0.67 for stage 2; trained on EMBARC stage 1 and tested on CANBIND-1, AUC = 0.66). The addition of neuroimaging features improved the prediction performance of antidepressant response compared with clinical features only.
The use of early-treatment (week 2) instead of pretreatment depression severity scores resulted in the best generalization performance, comparable to within-trial performance. Multivariate regressions showed substantial cross-trial generalizability in change in depression severity (predicted vs observed r ranging from 0.31 to 0.39).
Conclusions and Relevance
In this prognostic study of depression outcomes, models predicting response to antidepressants show substantial generalizability across different RCTs of adult MDD.