Summary: Researchers have created an accurate, easily interpretable new algorithm for predicting mild cognitive impairment and dementia in older adults.
Source: Columbia University
Using ensemble learning techniques and longitudinal data from a large naturalistic driving study, researchers at Columbia University’s Mailman School of Public Health, Fu Foundation School of Engineering and Applied Science, and Vagelos College of Physicians and Surgeons have developed a novel, interpretable and highly accurate algorithm for predicting mild cognitive impairment and dementia in older drivers.
Digital markers refer to variables generated from data captured through recording devices in the real-world setting. These data could be processed to measure driving behavior, performance and tempo-spatial pattern in exceptional detail.
The study is published in the journal Artificial Intelligence in Medicine.
The researchers used an interaction-based classification method for selecting predictive variables in the dataset. This learning model has achieved an accuracy of 96 percent in predicting mild cognitive impairment and dementia, outperforming traditional machine learning models such as logistic regression and random forests—a statistical technique widely used in AI for classifying disease status.
“Our new ensemble learning model based on digital markers and basic demographic characteristics could predict mild cognitive impairment and dementia in older drivers with excellent accuracy,” said Sharon Di, associate professor of civil engineering and engineering mechanics at Columbia Engineering and the study’s lead author.
The investigators constructed 200 variable modules using the naturalistic driving data on the driver, the vehicle and the environment captured by in-vehicle recording devices for 2977 drivers participating in the Longitudinal Research on Aging Drivers (LongROAD) project, a prospective cohort study conducted in five sites across the contiguous United States and sponsored by the AAA Foundation for Traffic Safety.
At the time of enrollment, the participants were active drivers aged 65-79 years who were cognitively intact. Data used in this study came from the first three years of follow-up, spanning from August 2015 through March 2019. During the follow-up, 36 participants were diagnosed with mild cognitive impairment, 8 with Alzheimer’s disease, and 17 with other or unspecified dementia.
The researchers performed a series of computer modeling experiments and found that the new ensemble learning model is 6-10 percent more accurate than random forests and logistic regression models in predicting mild cognitive impairment and dementia.
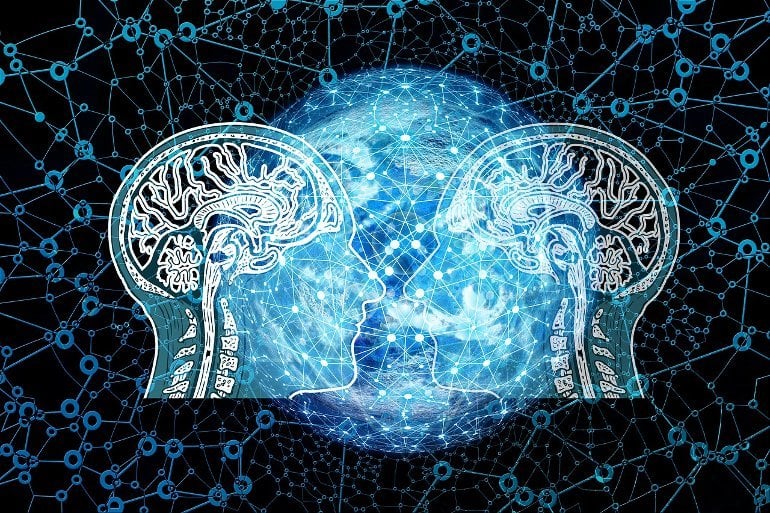
The two most influential driving variables are the right to left turn ratio and the number of hard braking events (defined as maneuvers with deceleration rates ≥ 0.4 g). “With advancing age, drivers make relatively fewer left turns and more right turns because left turns are riskier,” noted Di.
“About 85 percent of older adults in the United States are licensed drivers. As the most preferred mode of personal transportation, driving plays an important role in maintaining independence, self-control, social connection, and quality of life. Safely operating a car requires essential cognitive and physical functions.
“Our study indicates that digital markers embedded in routinely collected driving data can be used through innovative machine learning techniques as valid and reliable artificial intelligence for predicting mild cognitive impairment and dementia,” said Guohua Li, MD, DrPH, professor of epidemiology and anesthesiology at Columbia Mailman School of Public Health and Vagelos College of Physicians and Surgeons, and senior author.
“Early detection of mild cognitive impairment and dementia could lead to timely evaluation, diagnosis, and interventions, which are especially salient in the absence of effective therapeutics.”
About this artificial intelligence and dementia research news
Author: Press Office
Source: Columbia University
Contact: Press Office – Columbia University
Image: The image is in the public domain
Original Research: Closed access.
“Detecting mild cognitive impairment and dementia in older adults using naturalistic driving data and interaction-based classification from influence score” by Xuan Di et al. Artificial Intelligence in Medicine
Abstract
Detecting mild cognitive impairment and dementia in older adults using naturalistic driving data and interaction-based classification from influence score
Several recent studies indicate that atypical changes in driving behaviors appear to be early signs of mild cognitive impairment (MCI) and dementia. These studies, however, are limited by small sample sizes and short follow-up duration.
This study aims to develop an interaction-based classification method building on a statistic named Influence Score (i.e., I-score) for prediction of MCI and dementia using naturalistic driving data collected from the Longitudinal Research on Aging Drivers (LongROAD) project. Naturalistic driving trajectories were collected through in-vehicle recording devices for up to 44 months from 2977 participants who were cognitively intact at the time of enrollment. These data were further processed and aggregated to generate 31 time-series driving variables.
Because of high dimensional time-series features for driving variables, we used I-score for variable selection. I-score is a measure to evaluate variables’ ability to predict and is proven to be effective in differentiating between noisy and predictive variables in big data. It is introduced here to select influential variable modules or groups that account for compound interactions among explanatory variables. It is explainable regarding to what extent variables and their interactions contribute to the predictiveness of a classifier.
In addition, I-score boosts the performance of classifiers over imbalanced datasets due to its association with the F1 score. Using predictive variables selected by I-score, interaction-based residual blocks are constructed over top I-score modules to generate predictors and ensemble learning aggregates these predictors to boost the prediction of the overall classifier.
Experiments using naturalistic driving data show that our proposed classification method achieves the best accuracy (96%) for predicting MCI and dementia, followed by random forest (93%) and logistic regression (88%). In terms of F1 score and AUC, our proposed classifier achieves 98% and 87%, respectively, followed by random forest (with an F1 score of 96% and an AUC of 79%) and logistic regression (with an F1 score of 92% and an AUC of 77%).
The results indicate that incorporating I-score into machine learning algorithms could considerably improve the model performance for predicting MCI and dementia in older drivers.
We also performed the feature importance analysis and found that the right to left turn ratio and the number of hard braking events are the most important driving variables to predict MCI and dementia.