Summary: A new computational algorithm shows how the brain maintains information in the short term using specific types of neurons.
Source: Salk Institute
If you’ve ever forgotten something mere seconds after it was at the forefront of your mind–the name of a dish you were about to order at a restaurant, for instance–then you know how important working memory is. This type of short-term recall is how people retain information for a matter of seconds or minutes to solve a problem or carry out a task, like the next step in a series of instructions. But, although it’s critical in our day-to-day lives, exactly how the brain manages working memory has been a mystery.
Now, Salk scientists have developed a new computational model showing how the brain maintains information short-term using specific types of neurons. Their findings, published in Nature Neuroscience on December 7, 2020, could help shed light on why working memory is impaired in a broad range of neuropsychiatric disorders, including schizophrenia, as well as in normal aging.
“Most research on working memory focuses on the excitatory neurons in the cortex, which are numerous and broadly connected, rather than the inhibitory neurons, which are locally connected and more diverse,” says Terrence Sejnowski, head of Salk’s Computational Neurobiology Laboratory and senior author of the new work. “However, a recurrent neural network model that we taught to perform a working memory task surprised us by using inhibitory neurons to make correct decisions after a delay.”
In the new paper, Sejnowski and Robert Kim, a Salk and UC San Diego MD/PhD student, developed a computer model of the prefrontal cortex, an area of the brain known to manage working memory. The researchers used learning algorithms to teach their model to carry out a test typically used to gauge working memory in primates–the animals must determine whether a pattern of colored squares on a screen matches one that was seen several seconds earlier.
Sejnowski and Kim analyzed how their model was able to perform this task with high accuracy, and then compared it to existing data on the patterns of brain activity seen in monkeys carrying out the task. In both tests, the real and simulated neurons involved in working memory operated on a slower timescale than other neurons.
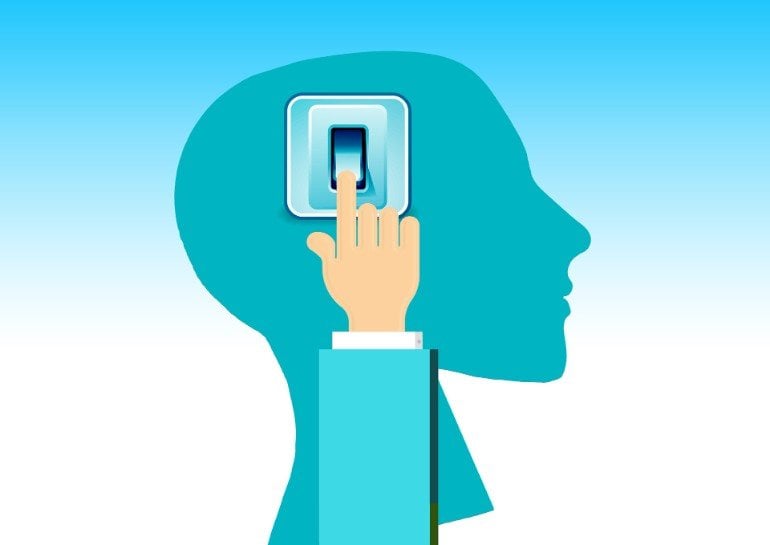
Kim and Sejnowski found that good working memory required both that long-timescale neurons be prevalent, and that connections between inhibitory neurons–which suppress brain activity–be strong. When they altered the strength of connections between these inhibitory neurons in their model, the researchers could change how well the model performed on the working memory test as well as the timescale of the pertinent neurons.
The new observations point toward the importance of inhibitory neurons, and could inspire future research on the role of these cells in working memory, the researchers say. They also could inform studies on why some people with neuropsychiatric disorders, including schizophrenia and autism, struggle with working memory.
“Working memory impairment is common in neuropsychiatric disorders, including schizophrenia and autism spectrum disorders,” says Kim. “If we can elucidate the mechanism of working memory, that’s a step toward understanding how working memory deficits arise in these disorders.”
Funding: The work was supported by a grant from the National Institute of Mental Health.
About this neuroscience and memory research news
Source: Salk Institute
Contact: Press Office – Salk Institute
Image: The image is in the public domain
Original Research: Closed access.
“Strong inhibitory signaling underlies stable temporal dynamics and working memory in spiking neural networks” by Robert Kim & Terrence J. Sejnowski. Nature Neuroscience
Abstract
Strong inhibitory signaling underlies stable temporal dynamics and working memory in spiking neural networks
Cortical neurons process information on multiple timescales, and areas important for working memory (WM) contain neurons capable of integrating information over a long timescale. However, the underlying mechanisms for the emergence of neuronal timescales stable enough to support WM are unclear. By analyzing a spiking recurrent neural network model trained on a WM task and activity of single neurons in the primate prefrontal cortex, we show that the temporal properties of our model and the neural data are remarkably similar. Dissecting our recurrent neural network model revealed strong inhibitory-to-inhibitory connections underlying a disinhibitory microcircuit as a critical component for long neuronal timescales and WM maintenance. We also found that enhancing inhibitory-to-inhibitory connections led to more stable temporal dynamics and improved task performance. Finally, we show that a network with such microcircuitry can perform other tasks without disrupting its pre-existing timescale architecture, suggesting that strong inhibitory signaling underlies a flexible WM network.