Summary: A new study reveals how a soft, compliant robotic hand, built with silicone skin, springs, and bendable joints, can self-organize grasps without needing precise environmental data or complex programming. The ADAPT hand succeeded in grasping 24 different objects with a 93% success rate using only four programmed motions, adapting naturally through mechanical flexibility.
Unlike traditional rigid robots that require exact control, this system relies on distributed mechanical intelligence to mimic the adaptability of human hands. Researchers now plan to integrate sensors and AI to combine the benefits of compliance with real-time feedback, expanding robotic potential in human-centered environments.
Key Facts:
- Self-Organized Grasping: ADAPT hand achieved 93% grasp success using only 4 motion instructions.
- Mechanical Intelligence: Compliance in skin, joints, and springs allows natural adaptation without extra feedback.
- Future Integration: Adding pressure sensors and AI will enhance control in unpredictable settings.
Source: EPFL
When you reach out your hand to grasp an object like a bottle, you generally don’t need to know the bottle’s exact position in space to pick it up successfully.
But as EPFL researcher Kai Junge explains, if you want to make a robot that can pick up a bottle, you must know everything about the surrounding environment very precisely.
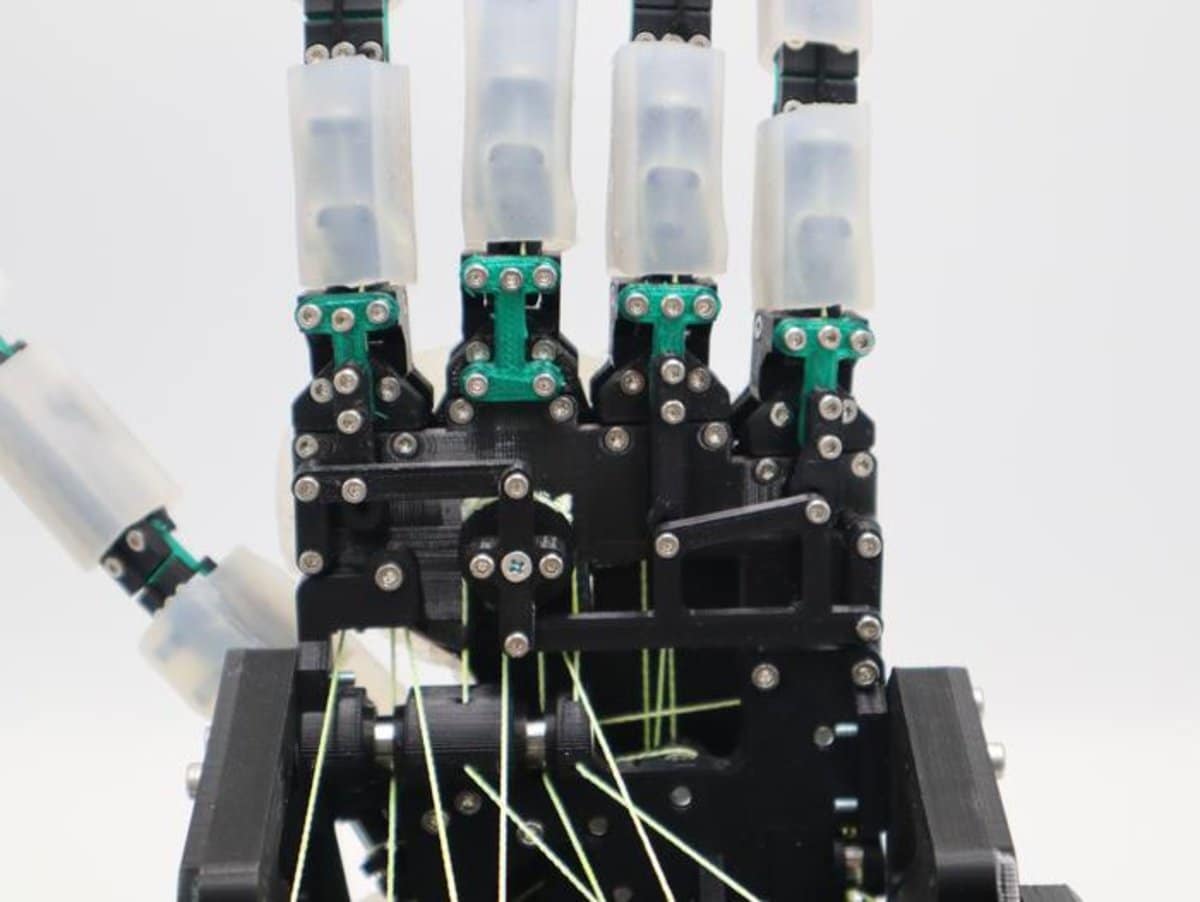
“As humans, we don’t really need too much external information to grasp an object, and we believe that’s because of the compliant – or soft – interactions that happen at the interface between an object and a human hand,” says Junge, a PhD student in the School of Engineering’s Computational Robot Design & Fabrication (CREATE) Lab, led by Josie Hughes.
“This compliance is what we are interested in exploring for robots.”
In robotics, compliant materials are those that deform, bend, and squish. In the case of the CREATE Lab’s robotic ADAPT hand (Adaptive Dexterous Anthropomorphic Programmable sTiffness), the compliant materials are relatively simple: strips of silicone wrapped around a mechanical wrist and fingers, plus spring-loaded joints, combined with a bendable robotic arm.
But this strategically distributed compliance is what allows the device to pick up a wide variety of objects using “self-organized” grasps that emerge automatically, rather than being programmed.
In a series of experiments, the ADAPT hand, which can be controlled remotely, was able to pick up 24 objects with a success rate of 93%, using self-organized grasps that mimicked a natural human grasp with a direct similarity of 68%.
The research has been published in Nature Communications Engineering.
‘Bottom-up’ robotic intelligence
While a traditional robotic hand would need a motor to actuate each joint, the ADAPT hand has only 12 motors, housed in the wrist, for its 20 joints. The rest of the mechanical control comes from springs, which can be made stiffer or looser to tune the hand’s compliance, and from the silicone ‘skin’, which can also be added or removed.
As for software, the ADAPT hand is programmed to move through just four general waypoints, or positions, to lift an object.
Any further adaptations required to complete the task occur without additional programming or feedback; in robotics, this is called ‘open loop’ control. For example, when the team programmed the robot to use a certain motion, it was able to adapt its grasp pose to various objects ranging from a single bolt to a banana.
The researchers analyzed this extreme robustness — thanks to the robot’s spatially distributed compliance — with over 300 grasps and compared them against a rigid version of the hand.
“Developing robots that can perform interactions or tasks that humans do automatically is a lot harder than most people expect,” Junge says.
“That’s why we are interested in exploiting this distributed mechanical intelligence of different body parts like skin, muscles, and joints, as opposed to the top-down intelligence of the brain.”
Balancing compliance and control
Junge emphasizes that the goal of the ADAPT study was not necessarily to create a robotic hand that can grasp like a human, but to show for the first time how much a robot can achieve through compliance alone.
Now that this has been demonstrated systematically, the EPFL team is building on the potential of compliance by re-integrating elements of closed-loop control into the ADAPT hand, including sensory feedback – via the addition of pressure sensors to the silicone skin – and artificial intelligence. This synergistic approach could lead to robots that combine compliance’s robustness to uncertainty, and the precision of closed-loop control.
“A better understanding of the advantages of compliant robots could greatly improve the integration of robotic systems into highly unpredictable environments, or into environments designed for humans,” Junge summarizes.
About this robotics research news
Author: Celia Luterbacher
Source: EPFL
Contact: Celia Luterbacher – EPFL
Image: The image is credited to CREATE Lab EPFL
Original Research: Open access.
“Spatially distributed biomimetic compliance enables robust anthropomorphic robotic manipulation” by Kai Junge et al. Communications Engineering
Abstract
Spatially distributed biomimetic compliance enables robust anthropomorphic robotic manipulation
The impressive capabilities of humans to robustly perform manipulation stems from compliant interactions, enabled by the structure and materials distributed in the hands.
We propose that mimicking this spatially distributed compliance in an anthropomorphic robotic hand enhances open-loop manipulation robustness and leads to human-like behaviors.
Here we introduce the ADAPT Hand, equipped with configurable compliant elements on the skin, fingers, and wrist.
After quantifying the effect of compliance on individual components against a rigid configuration, we experimentally analyze the performance of the full hand.
Through automated pick-and-place tests, we show the grasping robustness mirrors the estimated geometric theoretical limit, while stress-testing the robot to perform 800+ grasps.
Finally, 24 items with varying geometries are grasped in a constrained environment with a 93% success rate. We demonstrate that the hand-object self-organization behavior, driven by passive adaptation, underpins this robustness.
The hand exhibits different grasp types based on object geometries, with a 68% similarity to natural human grasps.