Summary: Focusing on the largest pyramidal neurons in the motor cortex, researchers found dendritic branches do not simply pass movement information forward. Each sub-branch calculates the information and passes it to larger sub-branches, which in turn, perform the same operation. Multiple dendritic branchlets can interact with each other to amplify their combined computational product.
Source: Technion-Isreal Institute of Technology
From the moment we are born, and even before that, we interact with the world through movement. We move our lips to smile or to talk. We extend our hand to touch. We move our eyes to see. We wiggle, we walk, we gesture, we dance.
How does our brain remember this wide range of motions? How does it learn new ones? How does it make the calculations necessary for us to grab a glass of water, without dropping it, squashing it, or missing it?
Technion Professor Jackie Schiller from the Ruth and Bruce Rappaport Faculty of Medicine and her team examined the brain at a single-neuron level to shed light on this mystery.
They found that computation happens not just in the interaction between neurons (nerve cells ), but within each individual neuron. Each of these cells, it turns out, is not a simple switch, but a complicated calculating machine.
This discovery, published recently in the Science magazine, promises changes not only to our understanding of how the brain works, but better understanding of conditions ranging from Parkinson’s disease to autism. And if that weren’t enough, these same findings are expected to advance machine learning, offering inspiration for new architectures.
Movement is controlled by the primary motor cortex of the brain. In this area, researchers are able to pinpoint exactly which neuron(s) fire at any given moment to produce the movement we see. Prof. Schiller’s team was the first to get even closer, examining the activity not of the whole neuron as a single unit, but of its parts.
Every neuron has branched extensions called dendrites. These dendrites are in close contact with the terminals (called axons) of other nerve cells, allowing the communication between them.
A signal travels from the dendrites to the cell’s body, and then transferred onwards through the axon. The number and structure of dendrites varies greatly between nerve cells, like the crown of one tree differs from the crown of another.
The particular neurons Prof. Schiller’s team focused on were the largest pyramidal neurons of the cortex. These cells, known to be heavily involved in movement, have a large dendritic tree, with many branches, sub-branches, and sub-sub-branches.
What the team discovered is that these branches do not merely pass information onwards. Each sub-sub-branch performs a calculation on the information it receives and passes the result to the bigger sub-branch.
The sub-branch than performs a calculation on the information received from all its subsidiaries and passes that on. Moreover, multiple dendritic branchlets can interact with one another to amplify their combined computational product.
The result is a complex calculation performed within each individual neuron. For the first time, Prof. Schiller’s team showed that the neuron is compartmentalised, and that its branches perform calculations independently.
“We used to think of each neuron as a sort of whistle, which either toots, or doesn’t,” Prof. Schiller explains.
“Instead, we are looking at a piano. Its keys can be struck simultaneously, or in sequence, producing an infinity of different tunes.” This complex symphony playing in our brains is what enables us to learn and perform an infinity of different, complex and precise movements.
Multiple neurodegenerative and neurodevelopmental disorders are likely to be linked to alterations in the neuron’s ability to process data.
In Parkinson’s disease, it has been observed that the dendritic tree undergoes anatomical and physiological changes. In light of the new discoveries by the Technion team, we understand that as a result of these changes, the neuron’s ability to perform parallel computation is reduced.
In autism, it looks possible that the excitability of the dendritic branches is altered, resulting in the numerous effects associated with the condition.
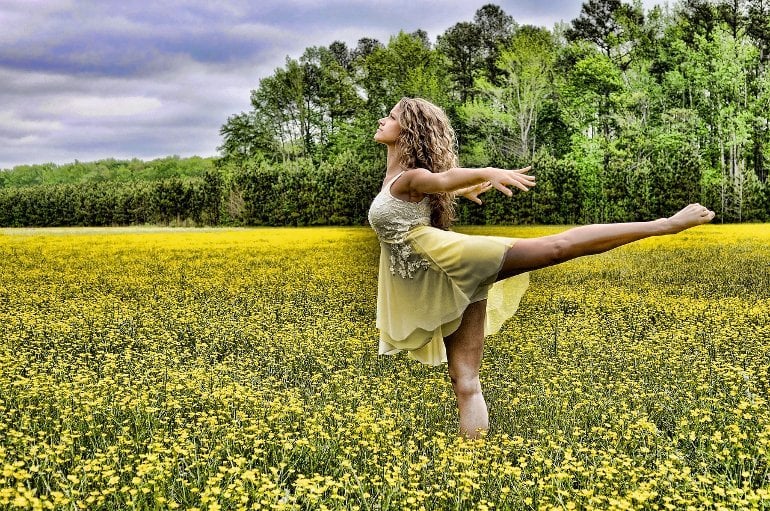
The novel understanding of how neurons work opens new research pathways with regards to these and other disorders, with the hope of their alleviation.
These same findings can also serve as an inspiration for the machine learning community. Deep neural networks, as their name suggests, attempt to create software that learns and functions somewhat similarly to a human brain.
Although their advances constantly make the news, these networks are primitive compared to a living brain. A better understanding of how our brain actually works can help in designing more complex neural networks, enabling them to perform more complex tasks.
This study was led by two of Prof. Schiller’s M.D.-Ph.D. candidate students Yara Otor and Shay Achvat, who contributed equally to the research. The team also included postdoctoral fellow Nate Cermak (now a neuroengineer) and Ph.D. student Hadas Benisty, as well as three collaborators: Professors Omri Barak, Yitzhak Schiller, and Alon Poleg-Polsky.
Funding: The study was partially supported by the Israeli Science Foundation, Prince funds, the Rappaport Foundation, and the Zuckerman Postdoctoral Fellowship.
About this movement research news
Author: Doron Shaham
Source: Technion-Israel Institute of Technology
Contact: Doron Shaham – Technion-Israel Institute of Technology
Image: The image is in the public domain
Original Research: Closed access.
“Dynamic compartmental computations in tuft dendrites of layer 5 neurons during motor behavior” by Jackie Schiller et al. Science
Abstract
Dynamic compartmental computations in tuft dendrites of layer 5 neurons during motor behavior
Tuft dendrites of layer 5 pyramidal neurons form specialized compartments important for motor learning and performance, yet their computational capabilities remain unclear.
Structural-functional mapping of the tuft tree from the motor cortex during motor tasks revealed two morphologically distinct populations of layer 5 pyramidal tract neurons (PTNs) that exhibit specific tuft computational properties.
Early bifurcating and large nexus PTNs showed marked tuft functional compartmentalization, representing different motor variable combinations within and between their two tuft hemi-trees.
By contrast, late bifurcating and smaller nexus PTNs showed synchronous tuft activation. Dendritic structure and dynamic recruitment of the N-methyl-D-aspartate (NMDA)–spiking mechanism explained the differential compartmentalization patterns.
Our findings support a morphologically dependent framework for motor computations, in which independent amplification units can be combinatorically recruited to represent different motor sequences within the same tree.