Summary: A new mathematical model can predict how the brain reacts when learning a rhythmic beat. The model shows how a neural network can act as a ‘neural metronome’ by estimating time intervals between beats within tens of milliseconds. The metronome relies on gamma oscillations to keep track of time.
Source: PLOS
A new mathematical model demonstrates how neurons in the brain could work together to learn and keep a musical beat. The framework, developed by Amitabha Bose of New Jersey Institute of Technology and Aine Byrne and John Rinzel of New York University, is described in PLOS Computational Biology.
Many experimental studies have established which brain areas are active when a person listens to music and discerns a beat. However, the neuronal mechanisms underlying the brain’s ability to learn a beat–and then keep it after the music stops–are unknown. Bose and his colleagues set out to explore what these neuronal mechanisms might be.
Using neurobiological principles, the researchers built a mathematical model of a group of neurons that can cooperate to learn a musical beat from a rhythmic stimulus and keep the beat after the stimulus stops. The model demonstrates how a network of neurons could act as a “neuronal metronome” by accurately estimating time intervals between beats within tens of millisecond accuracy. This metronome relies on rhythmic brain activity patterns known as gamma oscillations to keep track of time.
“We listen to music and within a few measures our body moves to the beat,” says Rinzel. “Our model suggests how the brain might learn a rhythm and learn it so fast.”
Next, the researchers plan to test their model with real-world psychoacoustic experiments and electroencephalogram (EEG) tests, which reveal activity in a person’s brain. These experiments will show how accurately the model might reflect actual neuronal mechanisms involved in learning a beat.
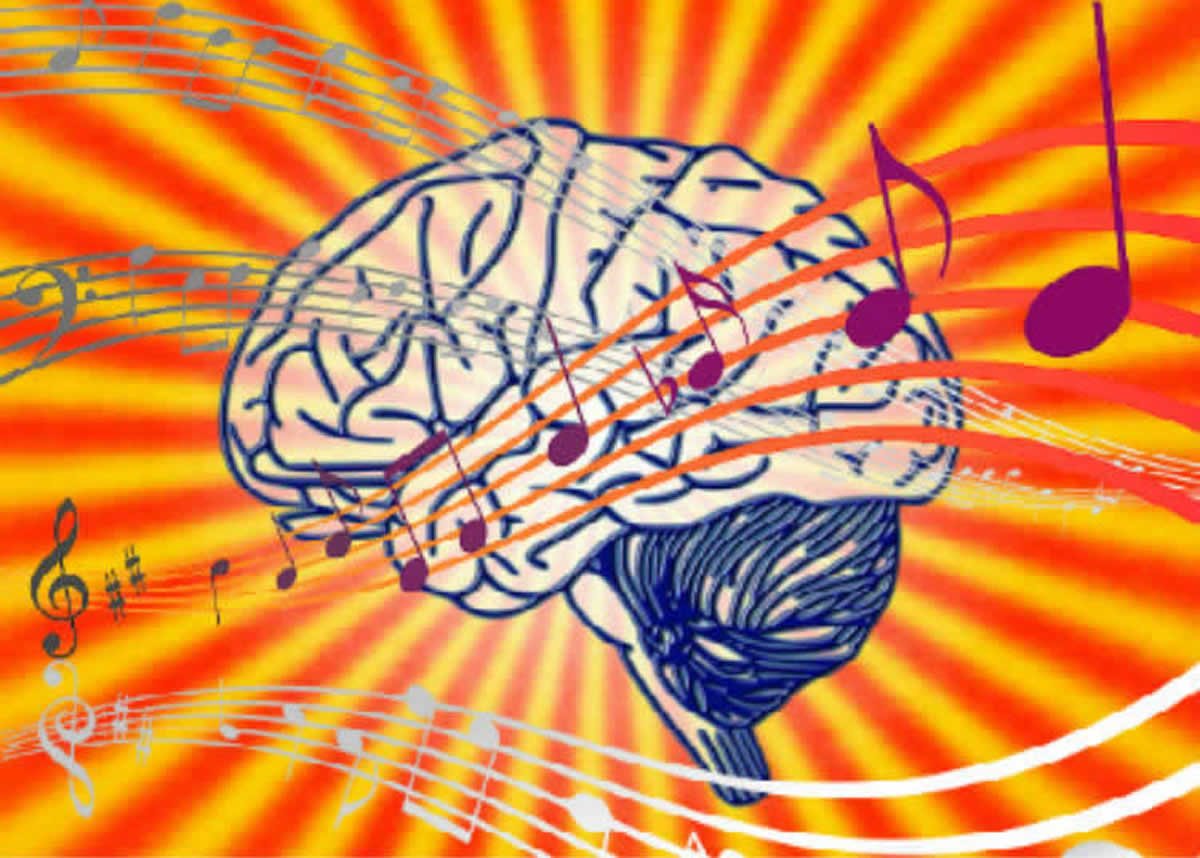
“Our findings provide new insights into how the brain might synthesize prior knowledge to make predictions about upcoming events, specifically in the realm of musical rhythm and keeping time,” Bose says. Beyond music, the new model could help improve understanding of conditions in which the ability to accurately estimate time is impaired, such as in Parkinson’s disease.
Funding: The authors A Bose and JR received no specific funding for this work. A Byrne was funded by the Swartz Foundation on a postdoctoral fellowship. The funders had no role in study design, data collection and analysis, decision to publish, or preparation of the manuscript.
Source:
PLOS
Media Contacts:
Amitabha Bose – PLOS
Image Source:
The image is in the public domain.
Original Research: Open access
“A neuromechanistic model for rhythmic beat generation”. Amitabha Bose, Áine Byrne, John Rinzel.
PLOS Computational Biology. doi:10.1371/journal.pcbi.1006450
Abstract
A neuromechanistic model for rhythmic beat generation
When listening to music, humans can easily identify and move to the beat. Numerous experimental studies have identified brain regions that may be involved with beat perception and representation. Several theoretical and algorithmic approaches have been proposed to account for this ability. Related to, but different from the issue of how we perceive a beat, is the question of how we learn to generate and hold a beat. In this paper, we introduce a neuronal framework for a beat generator that is capable of learning isochronous rhythms over a range of frequencies that are relevant to music and speech. Our approach combines ideas from error-correction and entrainment models to investigate the dynamics of how a biophysically-based neuronal network model synchronizes its period and phase to match that of an external stimulus. The model makes novel use of on-going faster gamma rhythms to form a set of discrete clocks that provide estimates, but not exact information, of how well the beat generator spike times match those of a stimulus sequence. The beat generator is endowed with plasticity allowing it to quickly learn and thereby adjust its spike times to achieve synchronization. Our model makes generalizable predictions about the existence of asymmetries in the synchronization process, as well as specific predictions about resynchronization times after changes in stimulus tempo or phase. Analysis of the model demonstrates that accurate rhythmic time keeping can be achieved over a range of frequencies relevant to music, in a manner that is robust to changes in parameters and to the presence of noise.