Summary: Lower gray matter volume in the brain is indicative of a higher risk of developing mental health disorders including depression and psychosis. However, those with slightly greater gray matter volume were more likely to recover from their disorders. Researchers believe reduced gray matter volume may be linked to higher levels of inflammation, reduced concentration, and other cognitive impairments associated with disorders like schizophrenia.
Source: University of Birmingham
The brain structure of patients with recent-onset psychosis and depression can offer important biological insights into these illnesses and how they might develop.
In a new study published in Biological Psychiatry, researchers at the University of Birmingham show that by examining structural MRI scans of the brain, it’s possible to identify patients most susceptible to poor outcomes.
By identifying these patients in the early stages of their illness, clinicians will be able to offer more targeted and effective treatments.
“Currently, the way we diagnose most mental health disorders is based on a patient’s history, symptoms, and clinical observations, rather than on biological information,” says lead author Paris Alexandros Lalousis.
“That means patients might have similar underlying biological mechanisms in their illness, but different diagnoses. By understanding those mechanisms more fully, we can give clinicians better tools to use in planning treatments.”
In the study, the researchers used data from around 300 patients with recent onset psychosis and recent onset depression taking part in the PRONIA study. PRONIA is a European Union-funded cohort study investigating prognostic tools for psychoses which is taking place across seven European research centres including Birmingham.
The researchers used a machine learning algorithm to assess data from patients’ brain scans and sort these into groups, or clusters. Two clusters were identified based on the scans, each of which contained both patients with psychosis and patients with depression. Each cluster revealed distinctive characteristics which related strongly to their likelihood of recovery.
In the first cluster, lower volumes of grey matter – the darker tissue inside the brain involved in muscle control and functions such as memory, emotions, and decision-making – were associated with patients who went on to have poorer outcomes.
In the second group, in contrast, higher levels of grey matter signalled patients who were more likely to recover well from their illness.
A second algorithm was then used to predict the patients’ condition nine months following the initial diagnosis. The researchers found a higher level of accuracy in predicting outcomes when using the biologically based clusters compared to traditional diagnostic systems.
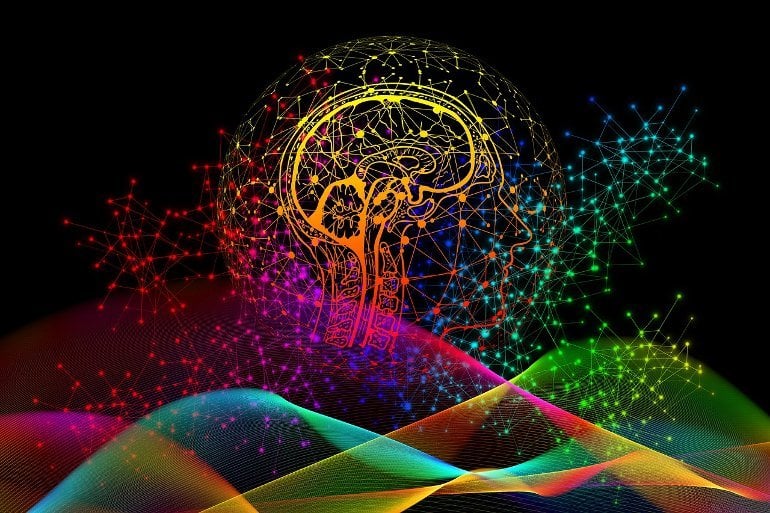
Evidence also showed that patients in the cluster with lower volumes of grey matter in their brain scans may have higher levels of inflammation, poorer concentration, and other cognitive impairments previously associated with depression and schizophrenia.
Finally, the team tested the clusters in other large cohort studies in Germany and the US and were able to show that the same identified clusters could be used to predict patient outcomes.
“While the PRONIA study contained people who were recently diagnosed with their illness, the other datasets we used contained people with chronic conditions,” explains Lalousis.
“We found that the longer the duration of illness, the more likely it was that a patient would fit into the first cluster with lower grey matter volume. That really adds to the evidence that structural MRI scans may be able to offer useful diagnostic information to help guide targeted treatment decisions.”=
The next step for the team is to start to validate the clusters in the clinic, gathering patient data in real time, before planning larger scale clinical trials.
About this mental health research news
Author: Beck Lockwood
Source: University of Birmingham
Contact: Beck Lockwood – University of Birmingham
Image: The image is in the public domain
Original Research: Open access.
“Neurobiologically Based Stratification of Recent Onset Depression and Psychosis: Identification of Two Distinct Transdiagnostic Phenotypes” by Paris Alexandros Lalousis et al. Biological Psychiatry
Abstract
Neurobiologically Based Stratification of Recent Onset Depression and Psychosis: Identification of Two Distinct Transdiagnostic Phenotypes
Background
Identifying neurobiologically based transdiagnostic categories of depression and psychosis may elucidate heterogeneity, and provide better candidates for predictive modelling. We aimed to identify clusters across patients with recent onset depression (ROD) and recent onset psychosis (ROP) based on structural neuroimaging data. We hypothesized that these transdiagnostic clusters would identify patients with poor outcome and allow more accurate prediction of symptomatic remission than traditional diagnostic structures.
Methods
HYDRA (HeterogeneitY through DiscRiminant Analysis) was trained on whole brain volumetric measures from 577 participants from the discovery sample of the multi-site PRONIA study to identify neurobiologically driven clusters which were then externally validated in the PRONIA replication sample (n=404) and three datasets of chronic samples (COBRE, n=146; MCIC, n=202; MUC, n=470).
Results
The optimal clustering solution was two transdiagnostic clusters (Cluster 1, n=153, 67 ROP, 86 ROD and Cluster 2, n=149, 88 ROP, 61 ROD; ARI=.618). The two clusters contained both ROP and ROD. One cluster had widespread GMV deficits, more positive, negative, and functional deficits (impaired cluster) and one cluster revealed a more preserved neuroanatomical signature and more ‘core’ depressive symptomatology (preserved cluster). The clustering solution was internally and externally validated and assessed for clinical utility in predicting 9-month symptomatic remission -outperforming traditional diagnostic structures.
Conclusions
We identified two transdiagnostic neuroanatomically informed clusters which are clinically and biologically distinct, challenging current diagnostic boundaries in recent onset mental health disorders. These results may aid understanding of aetiology of poor outcome patients transdiagnostically and improve development of stratified treatments.