Summary: Researchers have pioneered a method to measure ocular aging by studying proteins in eye fluid. Using artificial intelligence, they crafted an “eye-aging clock” from 26 out of nearly 6,000 proteins which can forecast aging.
Their findings can lead to more personalized medical treatments and help in understanding disease triggers. The team aims to employ this method to other body fluids, enhancing drug development for various ailments.
Key Facts:
- A novel technique, TEMPO, traces protein origins to pinpoint the cellular root of diseases.
- Out of approximately 6,000 proteins, 26 were identified that could predict ocular aging.
- Diseased eyes had proteins that signified advanced aging, with different diseases revealing different cellular culprits.
Source: Stanford
Using a technique they developed for studying eye fluid, Stanford Medicine researchers and their collaborators have found a way to measure ocular aging, opening avenues for treatment of numerous eye diseases.
The scientists looked at nearly 6,000 proteins in the fluid and found that they can use 26 of them to predict aging. Using artificial intelligence, they developed an eye–aging “clock,” indicating which proteins accelerate aging in each disease and revealing new potential targets for therapies.
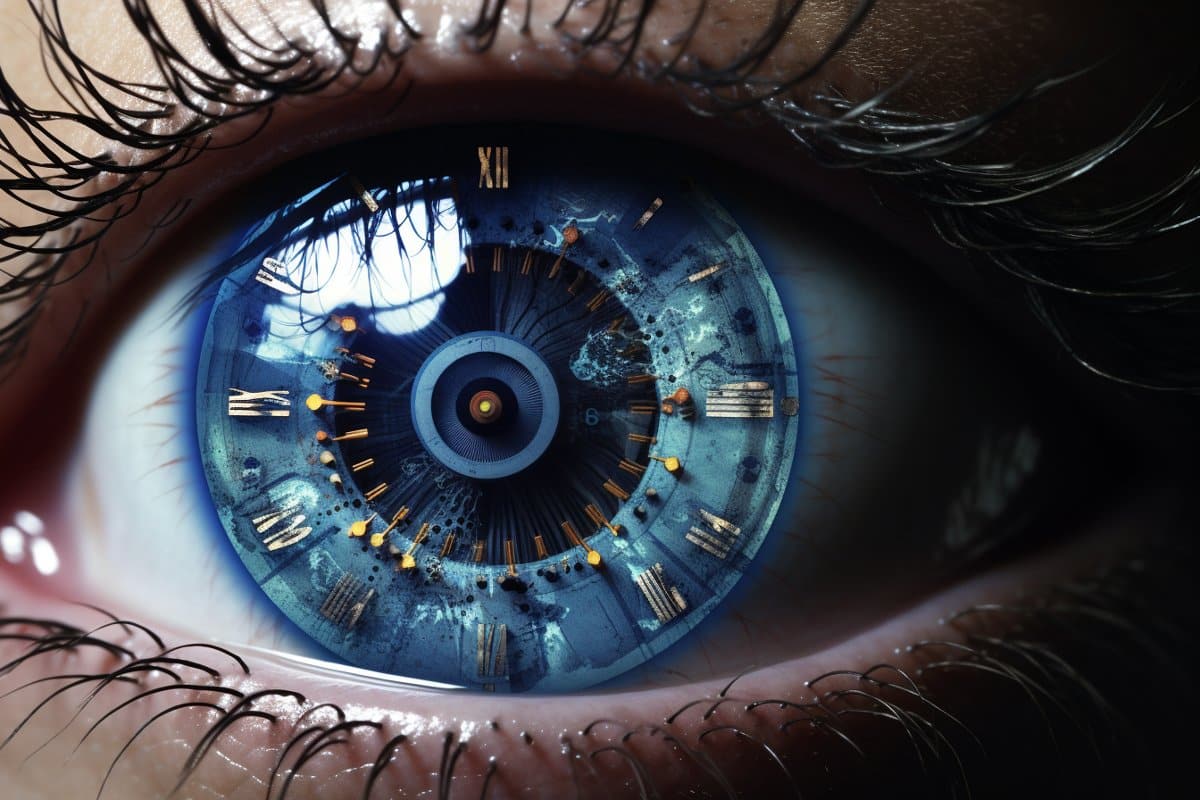
The study was published Oct. 19 in Cell. Vinit Mahajan, MD, PhD, a professor of ophthalmology, is the senior author, and Julian Wolf, MD, a postdoctoral scholar in Mahajan’s lab, is the lead author of the paper.
Mahajan and his colleagues intend to apply the clock method to other bodily fluids to develop more effective drugs for a variety of diseases.
“This is one of the best connections ever made that suggests disease triggers accelerated aging,” he said.
To glean the most information possible with small, renewable samples, Mahajan and his team developed a technique — TEMPO, or tracing expression of multiple protein origins. By tracing proteins to a type of cell where the RNA that creates the proteins resides, TEMPO allows the scientists to understand the cellular origin of disease-driving proteins with the hope that eventually they can target the cells with personalized medical treatments.
“The first step in developing any kind of successful therapy is understanding the molecules,” Mahajan said. “At the molecular level, patients present different manifestations even with the same disease. With a molecular fingerprint like we’ve developed, we could pick drugs that work for each patient.”
The culprit cells behind aging eyes
To better understand which cellular processes contribute to various eye diseases, the team analyzed liquid biopsies taken from the aqueous humor — fluid between the lens and the cornea — while patients were locally anesthetized during surgery.
The fluid was collected in patients with three types of eye diseases: diabetic retinopathy, which causes blood vessels in the eye to leak, leading to vision loss; retinitis pigmentosa, which causes light-sensitive cells in the back of the eye to break down; and uveitis, inflammation inside the eye.
Using eye fluid from 46 healthy patients, Mahajan and his team trained an AI algorithm to predict the age of the patient. They then fed the algorithm the nearly 6,000 proteins present in the fluid to see if a subset of these proteins could predict the patient’s age. They found 26 that could do so when used as a group.
Comparing the diseased eye fluid with the healthy fluid, they found that patients with diseased eyes had proteins that indicated a higher age: 12 years older in patients with early-stage diabetic retinopathy, 31 years in those with late-stage diabetic retinopathy, 16 years in retinitis pigmentosa patients and 29 years in uveitis patients.
The model also found that the cells responsible for indicating increased age were different with each disease: vascular cells in late-stage diabetic retinopathy, retinal cells in retinitis pigmentosa and immune cells in uveitis.
They also found that some cells commonly targeted in treatment are not the ones most involved in disease, encouraging a reevaluation of therapies. For example, diabetes drugs commonly target blood vessel cells because they become leaky with disease, but they found a big increase in proteins from healthy to late-stage diabetic retinopathy is in macrophages, an immune cell that removes dead cells.
The researchers found that some cells had showed accelerated aging before symptoms appeared. Treating the molecular pathway early, Mahajan said, could prevent disease damage before it becomes irreparable.
Informing clinical trials
Targeting both aging and disease cells could make treatment more effective, Mahajan said, because the two appear to act separately but simultaneously to damage the eye.
Mahajan anticipates that researchers will apply the TEMPO technique and aging clock to other organ fluids such as liver bile and joint fluid.
Mahajan hopes that by knowing these biomarkers, researchers will run more successful clinical trials because they will have a more refined look into the cellular processes driving disease. Currently, 90% of drug candidates tested in mice models or human cells fail in clinical trials. Knowing the cells driving disease and aging may increase chances of success, Mahajan said.
“It’s as if we’re holding these living cells in our hands and examining them with a magnifying glass,” Mahajan said. “We’re dialing in and getting to know our patients intimately at a molecular level, which will enable precision health and more informed clinical trials.”
Researchers from the Aarhus University in Denmark, University of Minnesota, Retina Consultants of Minnesota, University of Calgary, University of Iowa and Veterans Affairs Palo Alto Health Care System contributed to the work.
Funding: The research team was supported by NIH grants (R01EY031952, R01EY031360, R01EY030151, P30EY026877, R01EY030151, R01EY031952 and R35GM138353), Stanford University, Research to Prevent Blindness, VitreoRetinal Surgery Foundation, Lundbeck Foundation’s DARE fellowship and BrightFocus Foundation’s Macular Degeneration Research program.
The study was made possible by Stanford researchers affiliated with the Byers Eye Institute who created a biobank of eye fluid collected in the operating room.
About this AI, aging, and visual neuroscience research news
Author: Emily Moskal
Source: Stanford
Contact: Emily Moskal – Stanford
Image: The image is credited to Neuroscience News
Original Research: Closed access.
“Liquid-biopsy proteomics combined with AI identifies cellular drivers of eye aging and disease in vivo” by Vinit Mahajan et al. Cell
Abstract
Liquid-biopsy proteomics combined with AI identifies cellular drivers of eye aging and disease in vivo
Highlights
- Trace the cellular origin of >5,900 proteins in locally enriched fluid compartments
- Assess disease states at cellular resolution in non-regenerative organs in living humans
- AI proteomic clocks reveal accelerated cell aging in non-age-related diseases
- Molecular dysfunction of retinal cells during brain disease
Summary
Single-cell analysis in living humans is essential for understanding disease mechanisms, but it is impractical in non-regenerative organs, such as the eye and brain, because tissue biopsies would cause serious damage.
We resolve this problem by integrating proteomics of liquid biopsies with single-cell transcriptomics from all known ocular cell types to trace the cellular origin of 5,953 proteins detected in the aqueous humor.
We identified hundreds of cell-specific protein markers, including for individual retinal cell types. Surprisingly, our results reveal that retinal degeneration occurs in Parkinson’s disease, and the cells driving diabetic retinopathy switch with disease stage.
Finally, we developed artificial intelligence (AI) models to assess individual cellular aging and found that many eye diseases not associated with chronological age undergo accelerated molecular aging of disease-specific cell types.
Our approach, which can be applied to other organ systems, has the potential to transform molecular diagnostics and prognostics while uncovering new cellular disease and aging mechanisms.