Summary: Despite a lot of public worry that the AI revolution is going to impact the workforce, researchers report this is likely not to be the case. However, they note, the introduction to machine learning in the workforce could transform the economy as much as the introduction of the steam engine and other technologies did in the past.
Source: Carniegie Mellon University.
Machine learning computer systems, which get better with experience, are poised to transform the economy much as steam engines and electricity have in the past. They can outperform people in a number of tasks, though they are unlikely to replace people in all jobs.
So say Carnegie Mellon University’s Tom Mitchell and MIT’s Erik Brynjolfsson in a Policy Forum commentary to be published in the Dec. 22 edition of the journal Science. Mitchell, who founded the world’s first Machine Learning Department at CMU, and Brynjolfsson, director of the MIT Initiative on the Digital Economy in the Sloan School of Management, describe 21 criteria to evaluate whether a task or a job is amenable to machine learning (ML).
“Although the economic effects of ML are relatively limited today, and we are not facing the imminent ‘end of work’ as is sometimes proclaimed, the implications for the economy and the workforce going forward are profound,” they write. The skills people choose to develop and the investments businesses make will determine who thrives and who falters once ML is ingrained in everyday life, they argue.
ML is one element of what is known as artificial intelligence. Rapid advances in ML have yielded recent improvements in facial recognition, natural language understanding and computer vision. It already is widely used for credit card fraud detection, recommendation systems and financial market analysis, with new applications such as medical diagnosis on the horizon.
Predicting how ML will affect a particular job or profession can be difficult because ML tends to automate or semi-automate individual tasks, but jobs often involve multiple tasks, only some of which are amenable to ML approaches.
“We don’t know how all of this will play out,” acknowledged Mitchell, the E. Fredkin University Professor in CMU’s School of Computer Science. Earlier this year, for instance, researchers showed that a ML program could detect skin cancers better than a dermatologist. That doesn’t mean ML will replace dermatologists, who do many things other than evaluate lesions.
“I think what’s going to happen to dermatologists is they will become better dermatologists and will have more time to spend with patients,” Mitchell said. “People whose jobs involve human-to-human interaction are going to be more valuable because they can’t be automated.”
Tasks that are amenable to ML include those for which a lot of data is available, Mitchell and Brynjolfsson write. To learn how to detect skin cancer, for instance, ML programs were able to study more than 130,000 labeled examples of skin lesions. Likewise, credit card fraud detection programs can be trained with hundreds of millions of examples.
ML can be a game changer for tasks that already are online, such as scheduling. Jobs that don’t require dexterity, physical skills or mobility also are more suitable for ML. Tasks that involve making quick decisions based on data are a good fit for ML programs; not so if the decision depends on long chains of reasoning, diverse background knowledge or common sense.
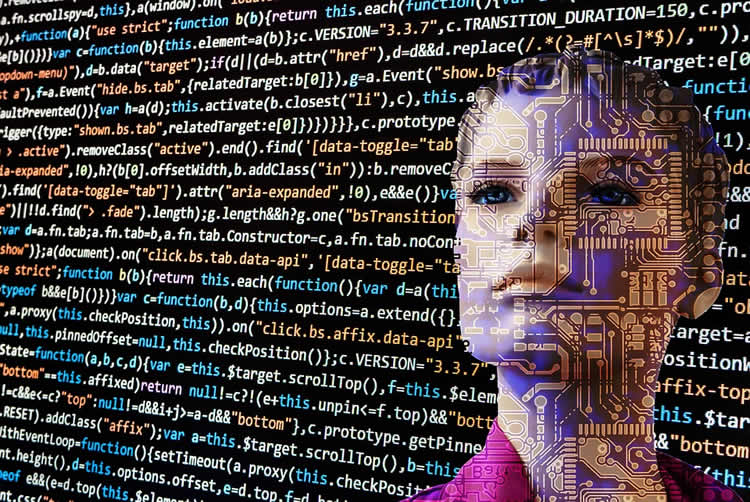
ML is not a good option if the user needs a detailed explanation for how a decision was made, according to the authors. In other words, ML might be better than a physician at detecting skin cancers, but a dermatologist is better at explaining why a lesion is cancerous or not.
Work is underway, however, on “explainable” ML systems.
Understanding the precise applicability of ML in the workforce is critical for understanding its likely economic impact, the authors say. Earlier this year, a National Academies of Sciences, Engineering and Medicine study on information technology and the workforce, co-chaired by Mitchell and Brynjolfsson, noted that information technology advances have contributed to growing wage inequality.
“Although there are many forces contributing to inequality, such as increased globalization, the potential for large and rapid changes due to ML, in many cases within a decade, suggests that the economic effects may be highly disruptive, creating both winners and losers,” they write. “This will require considerable attention among policy makers, business leaders, technologists and researchers.”
Source: Byron Spice – Carniegie Mellon University
Publisher: Organized by NeuroscienceNews.com.
Image Source: NeuroscienceNews.com image is credited to Tommy Leonardi.
Original Research: Abstract for “What can machine learning do? Workforce implications” by Erik Brynjolfsson and Tom Mitchell in Science. Published online December 21 2017 doi:10.1126/science.aap8062
[cbtabs][cbtab title=”MLA”]Carniegie Mellon University “How Machine Learning May Change Jobs.” NeuroscienceNews. NeuroscienceNews, 22 December 2017.
<https://neurosciencenews.com/machine-learning-jobs-8229/>.[/cbtab][cbtab title=”APA”]Carniegie Mellon University (2017, December 22). How Machine Learning May Change Jobs. NeuroscienceNews. Retrieved December 22, 2017 from https://neurosciencenews.com/machine-learning-jobs-8229/[/cbtab][cbtab title=”Chicago”]Carniegie Mellon University “How Machine Learning May Change Jobs.” https://neurosciencenews.com/machine-learning-jobs-8229/ (accessed December 22, 2017).[/cbtab][/cbtabs]
Abstract
What can machine learning do? Workforce implications
Digital computers have transformed work in almost every sector of the economy over the past several decades (1). We are now at the beginning of an even larger and more rapid transformation due to recent advances in machine learning (ML), which is capable of accelerating the pace of automation itself. However, although it is clear that ML is a “general purpose technology,” like the steam engine and electricity, which spawns a plethora of additional innovations and capabilities (2), there is no widely shared agreement on the tasks where ML systems excel, and thus little agreement on the specific expected impacts on the workforce and on the economy more broadly. We discuss what we see to be key implications for the workforce, drawing on our rubric of what the current generation of ML systems can and cannot do [see the supplementary materials (SM)]. Although parts of many jobs may be “suitable for ML” (SML), other tasks within these same jobs do not fit the criteria for ML well; hence, effects on employment are more complex than the simple replacement and substitution story emphasized by some. Although economic effects of ML are relatively limited today, and we are not facing the imminent “end of work” as is sometimes proclaimed, the implications for the economy and the workforce going forward are profound.
“What can machine learning do? Workforce implications” by Erik Brynjolfsson and Tom Mitchell in Science. Published online December 21 2017 doi:10.1126/science.aap8062