Summary: Widely used music algorithms are more likely to recommend and select music by male artists, at the detriment of female musicians. A new study addresses gender disparities in music-based algorithms.
Source: UPF Barcelona
Although the problem of gender discrimination is already found in the music industry, music recommendation algorithms are increasing the gender gap.
Andrés Ferraro and Xavier Serra, researchers of the Music Technology research group (MTG) of the UPF Department of Information and Communication Technologies (DTIC), with Christine Bauer, of the University of Utrecht (Netherlands), recently published a paper on gender balance in music recommendation systems in which they asked themselves how the system should work to avoid gender bias?
At the outset, the authors identified that gender justice was one of the artists’ main concerns.
Initially, the work by Ferraro, Serra and Bauer aimed to understand the fairness of music platforms available online from the artists’ point of view. In interviews conducted with music artists, they identified that gender justice was one of their main concerns.
Women have less exposure
The authors tested a commonly used music recommendation algorithm based on collaborative filtering and analysed the results of two datasets. In both cases, they found that the algorithm reproduces the existing bias in the dataset, in which only 25% of artists are women. In addition, the algorithm generates a ranking with artists to recommend to the user.
The authors saw that on average the first recommendation of a woman artist comes in 6th or 7th position, while that of a male artist is in the first position.
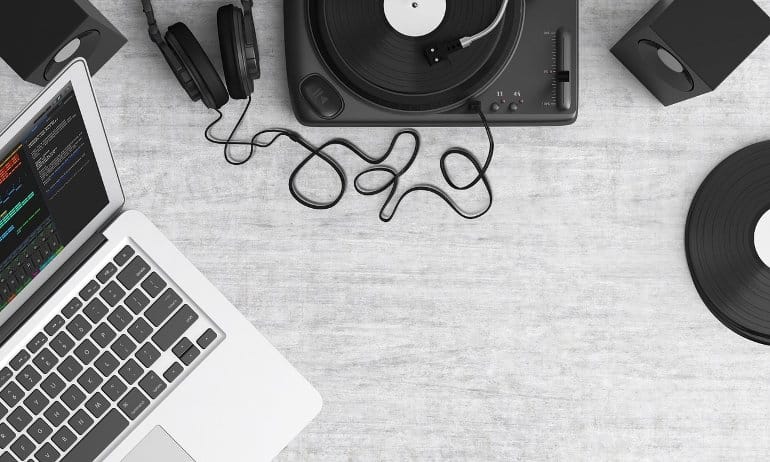
Ferraro, first author of the paper adds: “The bias in exposure comes from the way recommendations are generated”. This means that women have less exposure based on the recommendations of the system.
The situation worsens when taking into account that as users listen to recommended songs, the algorithm learns. This creates a feedback loop.
With the help of the reordered algorithm, users begin to change their behaviour so that they listen to more female artists
A new approach to compensate for gender bias
The authors of the study propose a new approach that would allow greater exposure of female artists and would consist of reordering the recommendation, which would move a specified number of positions downwards in order to solve the existing gender bias.
In a simulation, the authors studied how classified recommendations would affect user behaviour in the long run. The results showed that, with the help of the reclassified algorithm, users would begin to change their behaviour and thus listen to more female artists than with other music recommendation algorithms and, moreover, the new algorithm, based on machine learning, would consolidate this change in behaviour.
About this AI and gender discrimination research news
Source: UPF Barcelona
Contact: Núria Pérez – UPF Barcelona
Image: The image is in the public domain
Original Research: Open access.
“Break the Loop: Gender Imbalance in Music Recommenders” by Andrés Ferraro, Xavier Serra, Christine Bauer. Proceedings of the 2021 Conference on Human Information Interaction and Retrieval
Abstract
Break the Loop: Gender Imbalance in Music Recommenders
As recommender systems play an important role in everyday life, there is an increasing pressure that such systems are fair. Besides serving diverse groups of users, recommenders need to represent and serve item providers fairly as well.
In interviews with music artists, we identified that gender fairness is one of the artists’ main concerns. They emphasized that female artists should be given more exposure in music recommendations.
We analyze a widely-used collaborative filtering approach with two public datasets-enriched with gender information-to understand how this approach performs with respect to the artists’ gender. To achieve gender balance, we propose a progressive re-ranking method that is based on the insights from the interviews.
For the evaluation, we rely on a simulation of feedback loops and provide an in-depth analysis using state-of-the-art performance measures and metrics concerning gen-der fairness.