Summary: Computer analysis of patients reveals five clearly defined subgroups of psychosis and bipolar disorder. Those in group two were more likely to exhibit suicidal tendencies, while those in group five, for example, were characterized by schizophrenia diagnosis and lower verbal intelligence scores. Defining the characteristics of the subtypes could lead to improved personalized treatments for psychosis related mental illnesses.
Source: LUM
Psychiatrists led by LMU’s Nikolaos Koutsouleris have used a computer-based approach to assign psychotic patients diagnosed as bipolar or schizophrenic to five different subgroups. The method could lead to better therapies for psychoses.
Diagnostic methods capable of discriminating between the various types of psychoses recognized by psychiatrists remain inadequate. Up to now, doctors have assigned psychotic patients to one of two broad classes—bipolar disorder or schizophrenia—essentially on a symptomatic basis, focusing on shared elements of their psychiatric history, the range of symptoms displayed and the overall pattern of disease progression. This categorization remains a fundamental feature of both clinical practice and psychiatric research, although detailed observations indicate that psychotic illnesses, and the underlying genetic risk factors, are more heterogeneous than the conventional diagnostic dichotomy suggests. Now researchers led by LMU psychiatrist Nikolaos Koutsouleris has carried out a longitudinal cohort study on a sample of 1223 patients over a period of 18 months. The results obtained enabled the team to divide patients into five well defined subgroups, thus providing a more differentiated picture of the pathology of psychoses, which has implications for therapeutic interventions.
Data from 756 of the 1223 patients enrolled in the study were used to establish the new classification scheme, which was then independently validated for the remaining subset of participants. All 1223 patients had been diagnosed with classical psychoses, based on assessment of a total of 188 clinical variables relating to the trajectory of the individual’s condition, symptoms, ability to cope with the challenges of everyday life (‘functioning’), and cognitive performance. The study set out to determine whether their high-dimensional clinical dataset covering a wide spectrum of psychoses could be decomposed into defined subgroups based on clustering of statistically correlated variables. The data-driven analytical strategy adopted is based on machine learning, which can discover patterns that reveal ‘hidden structure’ in large collections of multifactorial data. These patterns may in turn point to differences in causal relationships that are of diagnostic relevance. “Our study shows that computer-based analyses can indeed help us to re-evaluate how persons with proven symptoms of psychoses can be differentiated diagnostically,” says LMU psychologist Dominic Dwyer, first author of the study, which appears in the journal JAMA Psychiatry.
The analysis ultimately led to the recognition of five clearly defined subgroups among the experimental population. “In addition to differences in their symptomatic and functional course, patients assigned to the different subgroups could also be distinguished on the basis of defined clinical fingerprints,” says Nikolaos Koutsouleris, who led the study. Members of one of the subgroups were also differentiated from all the others on the basis of their low scores for educational attainment, which is known to be a potential risk factor for psychotic illness.
The researchers used a mathematical approach known as non-negative matrix factorization to detect patterns in their statistical data. Using this procedure, they were able to reduce the starting dataset, comprised of 188 variables, to five subgroups defined by core factors. These factors encode hitherto unrecognized relationships between variables and uncover the functional links that connect them. “By evaluating the relative significance of these factors in individual cases, it is possible to assign patients to different groups on the basis of their overall scores,” Dwyer explains. In this way, the authors of the study were able to define the following five subgroups of psychoses: affective psychosis, suicidal psychosis, depressive psychosis, high-functioning psychosis and severe psychosis.
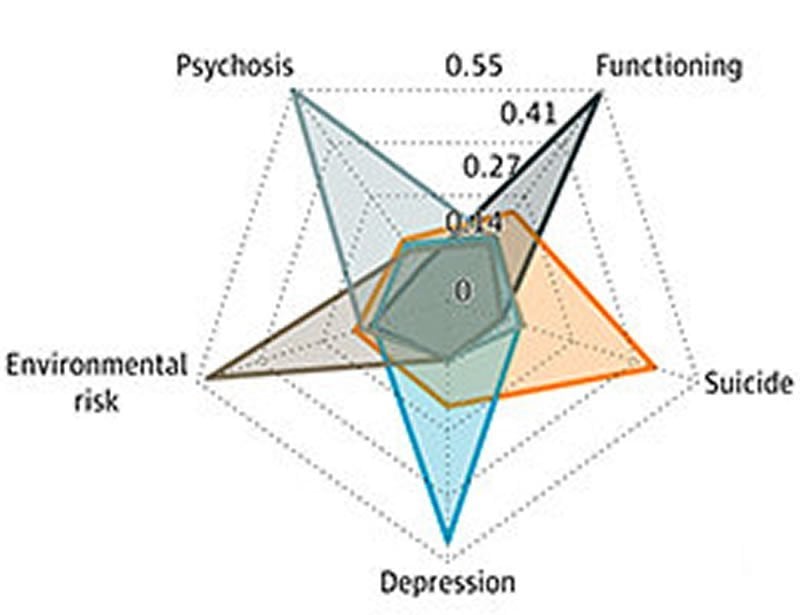
“Each of these subgroups can be clearly delimited from all the others on the basis of the clinical data,” says Koutsouleris. For instance, patients assigned to Group 5 are characterized by the core factors: schizophrenia diagnosis, significantly lower levels of educational attainment and low verbal intelligence. Most of the patients in this category were males and displayed marked symptoms of psychosis, but no indications of depression or mania. In Group 2, on the other hand, suicidal tendencies were clearly present. The results of the classification of this experimental population which provided the underlying data for the construction of the statistical model were confirmed for an independent group of 458 subjects.
The analyses suggested that unbiased, data-driven clustering may be used to stratify individuals into groups that have different clinical signatures, illness trajectories, and genetic underpinnings. In the future, such computer-assisted categorizations may be integrated into clinical routine through the use of online tools. Koutsouleris and his team have developed a prototype of such an online tool that can be used to stratify new individuals into the same groups and predict outcomes that can be tested at www.proniapredictors.eu.
Source:
LUM
Media Contacts:
Press Office – LUM
Image Source:
The image is credited to AG Koutsouleris.
Original Research: Closed access
“An Investigation of Psychosis Subgroups With Prognostic Validation and Exploration of Genetic Underpinnings: The PsyCourse Study”. Nikolaos Koutsouleris et al.
JAMA Psychiatry doi:10.1001/jamapsychiatry.2019.4910.
Abstract
An Investigation of Psychosis Subgroups With Prognostic Validation and Exploration of Genetic Underpinnings: The PsyCourse Study
Importance
Identifying psychosis subgroups could improve clinical and research precision. Research has focused on symptom subgroups, but there is a need to consider a broader clinical spectrum, disentangle illness trajectories, and investigate genetic associations.
Objective
To detect psychosis subgroups using data-driven methods and examine their illness courses over 1.5 years and polygenic scores for schizophrenia, bipolar disorder, major depression disorder, and educational achievement.
Design, Setting, and Participants
This ongoing multisite, naturalistic, longitudinal (6-month intervals) cohort study began in January 2012 across 18 sites. Data from a referred sample of 1223 individuals (765 in the discovery sample and 458 in the validation sample) with DSM-IV diagnoses of schizophrenia, bipolar affective disorder (I/II), schizoaffective disorder, schizophreniform disorder, and brief psychotic disorder were collected from secondary and tertiary care sites. Discovery data were extracted in September 2016 and analyzed from November 2016 to January 2018, and prospective validation data were extracted in October 2018 and analyzed from January to May 2019.
Main Outcomes and Measures
A clinical battery of 188 variables measuring demographic characteristics, clinical history, symptoms, functioning, and cognition was decomposed using nonnegative matrix factorization clustering. Subtype-specific illness courses were compared with mixed models and polygenic scores with analysis of covariance. Supervised learning was used to replicate results in validation data with the most reliably discriminative 45 variables.
Results
Of the 765 individuals in the discovery sample, 341 (44.6%) were women, and the mean (SD) age was 42.7 (12.9) years. Five subgroups were found and labeled as affective psychosis (n = 252), suicidal psychosis (n = 44), depressive psychosis (n = 131), high-functioning psychosis (n = 252), and severe psychosis (n = 86). Illness courses with significant quadratic interaction terms were found for psychosis symptoms (R2 = 0.41; 95% CI, 0.38-0.44), depression symptoms (R2 = 0.28; 95% CI, 0.25-0.32), global functioning (R2 = 0.16; 95% CI, 0.14-0.20), and quality of life (R2 = 0.20; 95% CI, 0.17-0.23). The depressive and severe psychosis subgroups exhibited the lowest functioning and quadratic illness courses with partial recovery followed by reoccurrence of severe illness. Differences were found for educational attainment polygenic scores (mean [SD] partial η2 = 0.014 [0.003]) but not for diagnostic polygenic risk. Results were largely replicated in the validation cohort.
Conclusions and Relevance
Psychosis subgroups were detected with distinctive clinical signatures and illness courses and specificity for a nondiagnostic genetic marker. New data-driven clinical approaches are important for future psychosis taxonomies. The findings suggest a need to consider short-term to medium-term service provision to restore functioning in patients stratified into the depressive and severe psychosis subgroups.