Summary: A new AI algorithm can detect behavioral symptoms associated with anxiety with over 90% accuracy.
Source: Simon Fraser University
Researchers are using artificial intelligence (AI) to detect behavioral signs of anxiety with more than 90 percent accuracy, and suggest that AI could have future applications for addressing mental health and well-being.
Their research is published in the journal Pervasive and Mobile Computing.
“In the two years since the onset of COVID-19, and one climate disaster after another, more and more people are experiencing anxiety,” says Simon Fraser University visiting professor and social psychologist Gulnaz Anjum. “Our research appears to show that AI could provide a highly reliable measurement for recognizing the signs that someone is anxious.”
Anjum and collaborators Nida Saddaf Khan and Sayeed Ghani from the Institute of Business Administration in Karachi, Pakistan collected an extensive range of data from adult participants for their Human Activity Recognition (HAR) study. Participants performed a series of activities in a specific order while wearing sensors that recorded their movements.
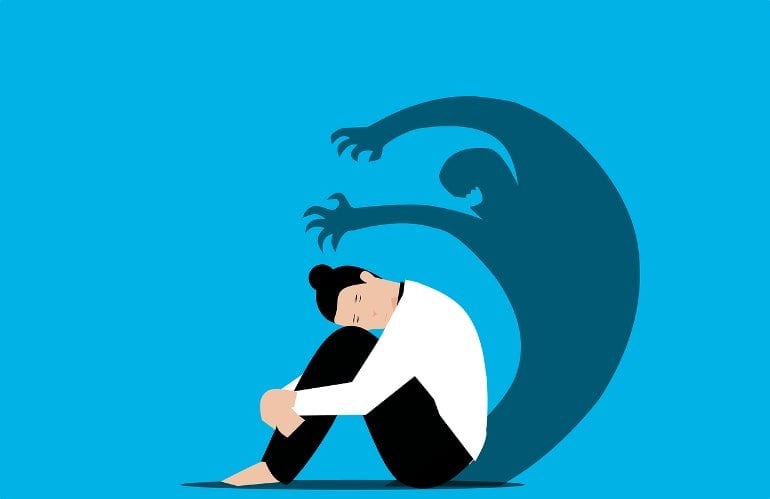
The researchers created a dataset of activities of typical anxiety-displaying behaviors for the sensors to detect, including idle sitting, nail biting, knuckle cracking and hands tapping. Their behaviors were analyzed using deep learning algorithms and computational hybrid models.
Anxiety disorder is the most common form of mental disorder, according to the American Psychiatric Association (APA), impacting 30 percent of the adult population at some point in their lives. The researchers suggest AI could help in the analysis, diagnosis, treatment and monitoring of psychological disorders such as anxiety disorder (AD).
“We’re hopeful that as more work is done to develop this method we can help to provide more accurate data for clinical research and practitioners,” says Anjum. “Our goal with this research is to expand horizons for the identification of anxiety disorders and ultimately, the improvement of people’s mental health.”
Khan says the rapid development in the field of AI and sensor technology “has made it possible to access and process the data related to mental, emotional and behavior disorders. It can be further researched and explored to understand unspoken behaviors and improve mental health at large.”
Ghani believes “there is tremendous opportunity for the healthcare industry to benefit from the applications of AI, and our research in using motion sensors for detecting anxiety-related behaviors is an example of what is possible.”
About this AI and anxiety research news
Author: Press Office
Source: Simon Fraser University
Contact: Press Office – Simon Fraser University
Image: The image is in the public domain
Original Research: Closed access.
“ADAM-sense: Anxiety-displaying activities recognition by motion sensors” by Nida Saddaf Khan et al. Pervasive and Mobile ComputingÂ
Abstract
ADAM-sense: Anxiety-displaying activities recognition by motion sensors
In the field of Human Activity Recognition (HAR), human activities are recognized based on sensors’ streaming data. HAR has been utilized widely in various fields of application where the studies of human behaviors are conducted such as healthcare, personal care, aged care, and several other domains.
This approach can also be beneficial in the field of psychiatry where the patients suffer from mental, emotional, and behavioral disorder. According to American Psychiatric Association (APA), the most common form of mental disorder is Anxiety Disorder (AD) effecting 30% of the adult population at some point in their life. In this paper, a HAR based method is proposed to recognize some behaviors pertaining to anxiety display. To make such model, a novel dataset of anxious behaviors is also created with unique features using motion sensors of smartphone and Inertial Measurement Unit (IMU).
Several deep learning-based models are created and compared against random forest and gradient boost algorithms, where a deep model comprising Convolution Neural Network (CNN) and Long-Short Term Memory (LSTM) is shown to perform better than other models and could recognize anxiety-related behaviors with over 92% accuracy.