Summary: Artificial intelligence helps shed new light on why many with autism have a difficult time when it comes to processing emotions via facial expressions.
Source: MIT
Many of us easily recognize emotions expressed in others’ faces. A smile may mean happiness, while a frown may indicate anger. Autistic people often have a more difficult time with this task. It’s unclear why.
But new research, published June 15 in The Journal of Neuroscience, sheds light on the inner workings of the brain to suggest an answer. And it does so using a tool that opens new pathways to modeling the computation in our heads: artificial intelligence.
Researchers have primarily suggested two brain areas where the differences might lie. A region on the side of the primate (including human) brain called the inferior temporal (IT) cortex contributes to facial recognition.
Meanwhile, a deeper region called the amygdala receives input from the IT cortex and other sources and helps process emotions.
Kohitij Kar, a research scientist in the lab of MIT Professor James DiCarlo, hoped to zero in on the answer. (DiCarlo, the Peter de Florez Professor in the Department of Brain and Cognitive Sciences, is a member of the McGovern Institute for Brain Research and director of MIT’s Quest for Intelligence.)
Kar began by looking at data provided by two other researchers: Shuo Wang at Washington University in St. Louis and Ralph Adolphs at Caltech. In one experiment, they showed images of faces to autistic adults and to neurotypical controls.
The images had been generated by software to vary on a spectrum from fearful to happy, and the participants judged, quickly, whether the faces depicted happiness. Compared with controls, autistic adults required higher levels of happiness in the faces to report them as happy.
Modeling the brain
Kar, who is also a member of the Center for Brains, Minds and Machines, trained an artificial neural network, a complex mathematical function inspired by the brain’s architecture, to perform the same task. The network contained layers of units that roughly resemble biological neurons that process visual information.
These layers process information as it passes from an input image to a final judgment indicating the probability that the face is happy. Kar found that the network’s behavior more closely matched the neurotypical controls than it did the autistic adults.
The network also served two more interesting functions. First, Kar could dissect it. He stripped off layers and retested its performance, measuring the difference between how well it matched controls and how well it matched autistic adults. This difference was greatest when the output was based on the last network layer.
Previous work has shown that this layer in some ways mimics the IT cortex, which sits near the end of the primate brain’s ventral visual processing pipeline. Kar’s results implicate the IT cortex in differentiating neurotypical controls from autistic adults.
The other function is that the network can be used to select images that might be more efficient in autism diagnoses. If the difference between how closely the network matches neurotypical controls versus autistic adults is greater when judging one set of images versus another set of images, the first set could be used in the clinic to detect autistic behavioral traits.
“These are promising results,” Kar says. Better models of the brain will come along, “but oftentimes in the clinic, we don’t need to wait for the absolute best product.”
Next, Kar evaluated the role of the amygdala. Again, he used data from Wang and colleagues. They had used electrodes to record the activity of neurons in the amygdala of people undergoing surgery for epilepsy as they performed the face task.
The team found that they could predict a person’s judgment based on these neurons’ activity. Kar reanalyzed the data, this time controlling for the ability of the IT-cortex-like network layer to predict whether a face truly was happy.
Now, the amygdala provided very little information of its own. Kar concludes that the IT cortex is the driving force behind the amygdala’s role in judging facial emotion.
Noisy networks
Finally, Kar trained separate neural networks to match the judgments of neurotypical controls and autistic adults. He looked at the strengths or “weights” of the connections between the final layers and the decision nodes. The weights in the network matching autistic adults, both the positive or “excitatory” and negative or “inhibitory” weights, were weaker than in the network matching neurotypical controls. This suggests that sensory neural connections in autistic adults might be noisy or inefficient.
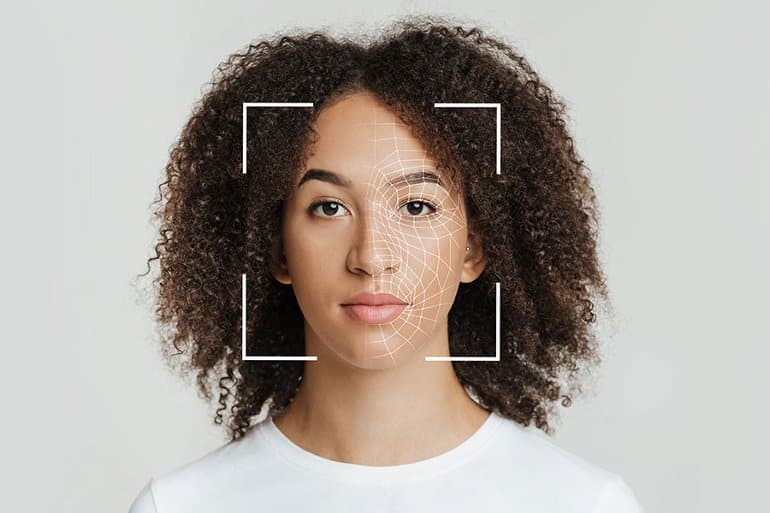
To further test the noise hypothesis, which is popular in the field, Kar added various levels of fluctuation to the activity of the final layer in the network modeling autistic adults. Within a certain range, added noise greatly increased the similarity between its performance and that of the autistic adults.
Adding noise to the control network did much less to improve its similarity to the control participants. This further suggest that sensory perception in autistic people may be the result of a so-called “noisy” brain.
Computational power
Looking forward, Kar sees several uses for computational models of visual processing. They can be further prodded, providing hypotheses that researchers might test in animal models.
“I think facial emotion recognition is just the tip of the iceberg,” Kar says.
They can also be used to select or even generate diagnostic content. Artificial intelligence could be used to generate content like movies and educational materials that optimally engages autistic children and adults. One might even tweak facial and other relevant pixels in what autistic people see in augmented reality goggles, work that Kar plans to pursue in the future.
Ultimately, Kar says, the work helps to validate the usefulness of computational models, especially image-processing neural networks. They formalize hypotheses and make them testable. Does one model or another better match behavioral data?
“Even if these models are very far off from brains, they are falsifiable, rather than people just making up stories,” he says. “To me, that’s a more powerful version of science.”
About this AI and ASD research news
Author: Anne Trafton
Source: MIT
Contact: Anne Trafton – MIT
Image: The image is credited to MIT
Original Research: Closed access.
“A computational probe into the behavioral and neural markers of atypical facial emotion processing in autism” by Kohitij Kar. Journal of Neuroscience
Abstract
A computational probe into the behavioral and neural markers of atypical facial emotion processing in autism
Despite ample behavioral evidence of atypical facial emotion processing in individuals with autism spectrum disorder (ASD), the neural underpinnings of such behavioral heterogeneities remain unclear.
Here, I have used brain-tissue mapped artificial neural network (ANN) models of primate vision to probe candidate neural and behavior markers of atypical facial emotion recognition in ASD at an image-by-image level. Interestingly, the ANNs’ image-level behavioral patterns better matched the neurotypical subjects’ behavior than those measured in ASD.
This behavioral mismatch was most remarkable when the ANN behavior was decoded from units that correspond to the primate inferior temporal (IT) cortex. ANN-IT responses also explained a significant fraction of the image-level behavioral predictivity associated with neural activity in the human amygdala (from epileptic patients without ASD)— strongly suggesting that the previously reported facial emotion intensity encodes in the human amygdala could be primarily driven by projections from the IT cortex.
In sum, these results identify primate IT activity as a candidate neural marker and demonstrate how ANN models of vision can be used to generate neural circuit-level hypotheses and guide future human and non-human primate studies in autism.
Significance Statement:
Moving beyond standard parametric approaches that predict behavior with high-level categorical descriptors of a stimulus (e.g., level of happiness/fear in a face image), in this study, I demonstrate how an image-level probe, utilizing current deep-learning based artificial neural network (ANN) models, allows identification of more diagnostic stimuli for autism spectrum disorder enabling the design of more powerful experiments.
This study predicts that inferior temporal (IT) cortex activity is a key candidate neural marker of atypical facial emotion processing in people with ASD.
Importantly, the results strongly suggest that ASD-related atypical facial emotion intensity encodes in the human amygdala could be primarily driven by projections from the IT cortex.