Summary: Researchers have developed a virtual reality and AI-based system that can detect autism spectrum disorder (ASD) in young children with over 85% accuracy—outperforming traditional assessment methods. The system observes children’s motor movements and gaze patterns while they engage in tasks within immersive virtual environments, enabling more naturalistic responses than typical lab settings.
Using a deep learning model, the system identifies behavioral biomarkers linked to ASD and delivers a diagnosis efficiently and affordably. This innovation could significantly expand access to early autism detection and lays the groundwork for studying other motor symptoms in ASD.
Key Facts:
- High Accuracy: The VR-AI system achieved over 85% accuracy in detecting ASD.
- Natural Interaction: Children’s behaviors are assessed in realistic virtual environments, enhancing diagnostic validity.
- Accessible Tools: The system uses commercially available cameras and screens, making widespread use feasible.
Source: UPV
A team from the Human-Tech Institute-Universitat Politècnica de València has developed a new system for the early detection of Autism Spectrum Disorder (ASD) using virtual reality and artificial intelligence.
The system has achieved an accuracy of over 85%, thus surpassing traditional methods of detecting autism in early childhood, which are usually based on psychological tests and interviews carried out manually.
The results of the work of the UPV team have been published in the Expert Systems with Applications journal.
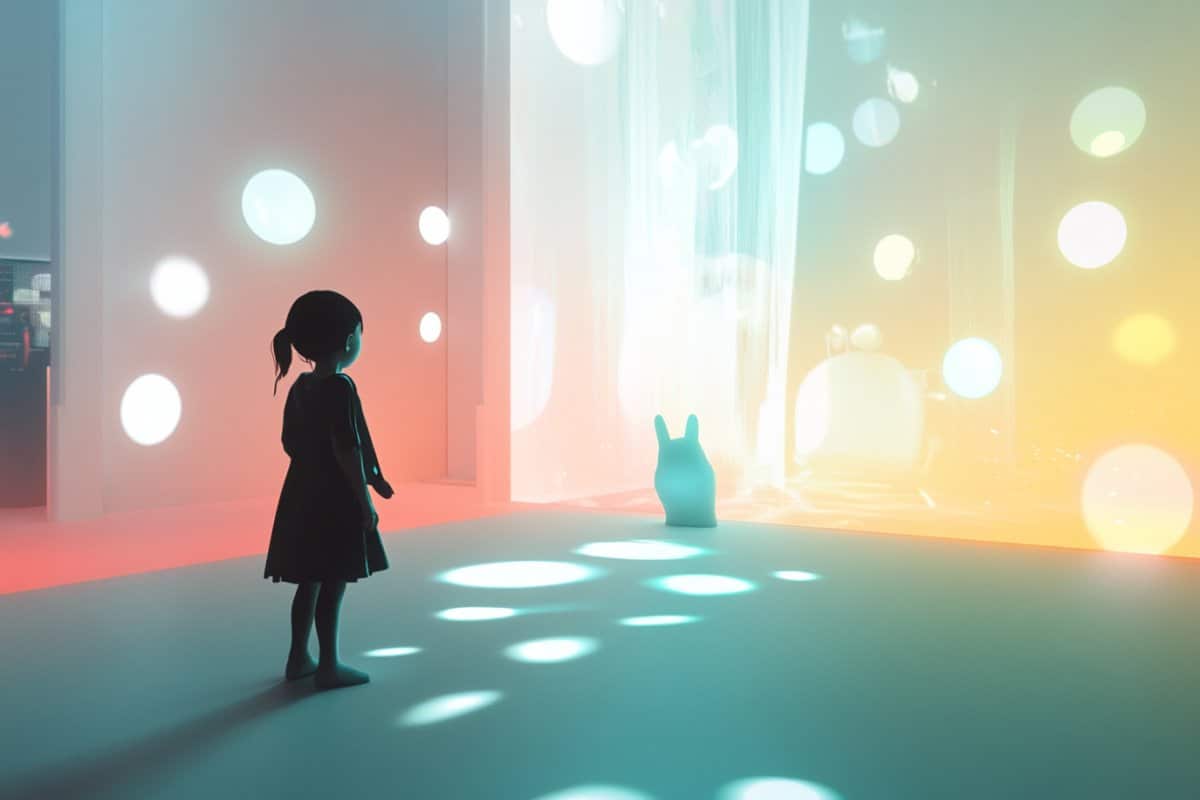
In the study, the team from the Human-Tech Institute analysed the movements of children performing multiple tasks in virtual reality to determine which artificial intelligence technique is most appropriate for identifying ASD.
‘The use of virtual reality allows us to use recognisable environments that generate realistic and authentic responses, imitating how children interact in their daily lives.
‘This is a significant improvement over laboratory tests, in which responses are often artificial. With virtual reality, we can study more genuine reactions and better understand the symptoms of autism,’ says Mariano Alcañiz, director of the Human-Tech Institute at the UPV.
The virtual system consists of projecting, on the walls of a room or a large-format screen, a simulated environment in which the child’s image is integrated while performing multiple tasks, captured by a camera that analyses their movements.
‘This method standardises the detection of autism by analysing biomarkers related to behaviour, motor activity and gaze direction.
‘Our system only requires a large screen and a type of camera that is already on the market and is cheaper than the usual test-based evaluation method. Without doubt, it would facilitate access to diagnosis as it could be included in any early intervention space’, emphasises Mariano Alcañiz.
New artificial intelligence model
On the other hand, as explained by the researcher Alberto Altozano, who developed the AI model together with Professor Javier Marín, taking advantage of the experience acquired in the analysis of motor data, the UPV team compared traditional AI techniques with an innovative deep learning model.
‘The results reveal that the proposed new model can identify ASD with greater precision and in a greater number of tasks within the VR experience,’ says Altozano.
Once the child’s movements during the virtual experience have been automatically processed, the system establishes a diagnosis that, according to those responsible for the study, improves both the accuracy and the efficiency of conventional techniques.
Eight years of collaboration to improve early detection
Over the last eight years, the Human-Tech Institute of the UPV team has worked on perfecting the early detection of ASD, collaborating with the Red Cenit cognitive development centre, and developing and validating the semi-immersive system.
Within this framework, the researcher Eleonora Minissi recently presented her doctoral thesis, in which not only was the virtual reality system validated through studies with autistic children, but also the effectiveness of the various biomarkers measured during the virtual experience was compared.
Her research highlights that, despite the growing interest in social-visual attention in ASD, atypical motor patterns have received less diagnostic attention.
The researcher concludes that the ‘ease with which this data can be collected and its high effectiveness in detecting autism make the motor activity a promising biomarker’.
In addition, the latest results of the work of the Human-Tech Institute team suggest that the new AI can be adapted and trained to analyse the movements of ASD patients in other tasks.
‘This opens the door to future explorations of the motor symptomatology of autism such as: what are the motor characteristics of autistic children when walking or talking?’ adds Mariano Alcañiz.
About this AI, ASD, and virtual reality research news
Author: Luis Zurano
Source: UPV
Contact: Luis Zurano – UPV
Image: The image is credited to Neuroscience News
Original Research: Open access.
“Introducing 3DCNN ResNets for ASD full-body kinematic assessment: A comparison with hand-crafted features” by Mariano Alcañiz et al. Expert Systems with Applications
Abstract
Introducing 3DCNN ResNets for ASD full-body kinematic assessment: A comparison with hand-crafted features
Autism Spectrum Disorder (ASD) is characterized by challenges in social communication and restricted patterns, with motor abnormalities gaining traction for early detection.
However, kinematic analysis in ASD is limited, often lacking robust validation and relying on hand-crafted features for single tasks, leading to inconsistencies across studies.
End-to-end models have emerged as promising methods to overcome the need for feature engineering.
Our aim is to propose a newly adapted 3DCNN ResNet from action recognition and compare it to widely used hand-crafted features for motor ASD assessment.
Specifically, we developed a virtual reality environment with multiple motor tasks and trained models using both approaches.
We prioritized a reliable validation framework with subject-wise nestedrepeated cross-validation.
Results show the proposed model achieves a maximum accuracy of 85±3%, outperforming state-of-the-art end-to-end models with short 1-to-3 min samples.
Our comparative analysis with hand-crafted features shows feature-engineered models outperformed our end-to-end model in certain tasks.
However, generalized linear mixed-effects models showed that our end-to-end model achieved a statistically higher mean AUC (0.80±0.03) and Sensitivity (66±3%), while showing less variability across all VR tasks, demonstrating domain generalization and reliability.
These findings show that end-to-end models enable less variable and context-independent ASD classification without requiring domain knowledge or task specificity.
However, they also recognize the effectiveness of hand-crafted features in specific task scenarios.