Summary: A new study found that artificial intelligence can better identify instances of physical child abuse in emergency rooms compared to traditional diagnostic coding methods. Researchers developed a machine-learning model that more accurately estimated abuse prevalence based on high-risk injuries and physical abuse indicators.
The AI approach outperformed methods relying solely on diagnostic codes, which misdiagnosed about 8.5% of cases. These findings suggest that AI could significantly improve early detection and treatment of child abuse, leading to better protection for vulnerable children.
Key Facts:
- Improved Accuracy: Traditional coding methods missed an average of 8.5% of child abuse cases.
- AI Advantage: Machine learning models provided a more accurate picture of child abuse trends in emergency departments.
- Vulnerable Population: The study focused on 3,317 cases involving children under 10, with most under age 2.
Source: Pediatric Academic Societies
Artificial intelligence (AI) can help better identify prevalence of physical abuse of children seen in the emergency room, a new study found.
The research will be presented at the Pediatric Academic Societies (PAS) 2025 Meeting, held April 24-28 in Honolulu.
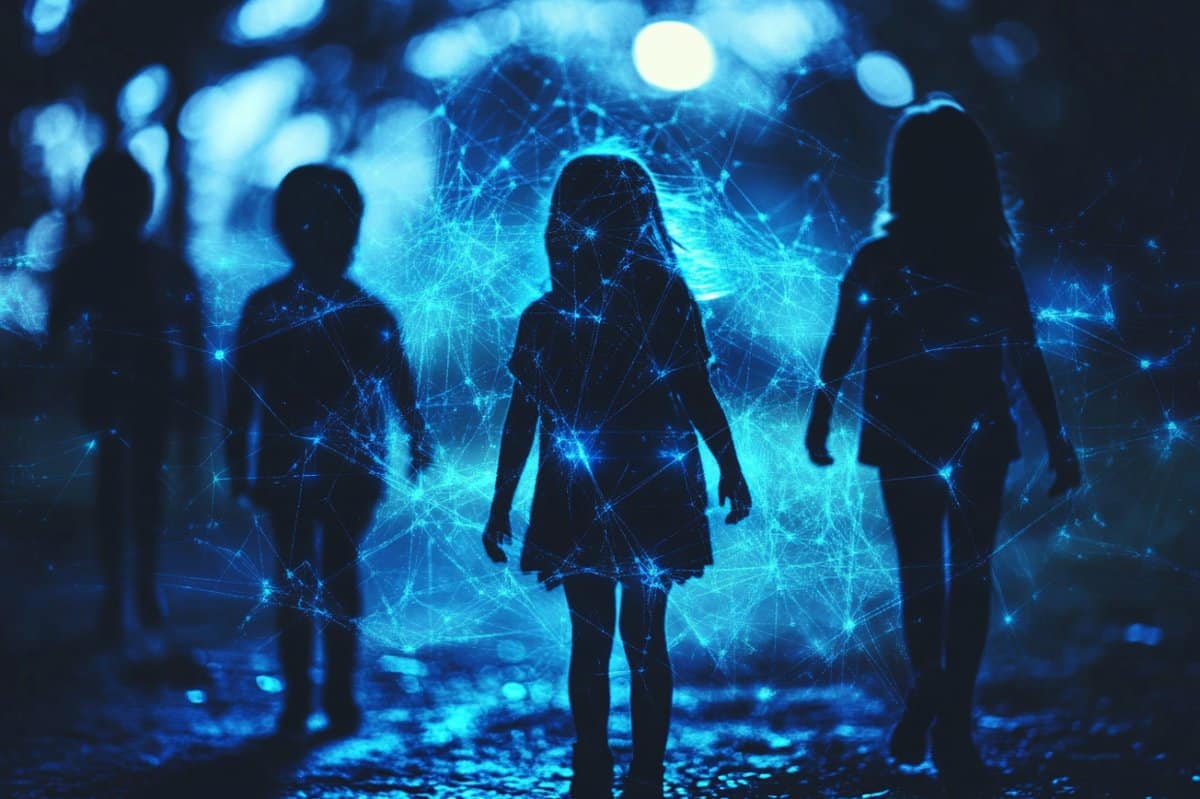
Researchers used a machine-learning model to estimate instances of child abuse seen in emergency departments based on diagnostic codes for high-risk injury and physical abuse.
The researchers’ approach better predicted abuse rates than those that rely solely on diagnostic codes entered by a provider or administrative staff. Relying on abuse codes alone misdiagnosed on average 8.5% of cases.
“Our AI approach offers a clearer look at trends in child abuse, which helps providers more appropriately treat abuse and improve child safety,” said Farah Brink, MD, child abuse pediatrician at Nationwide Children’s Hospital, and assistant professor at The Ohio State University.
“AI-powered tools hold tremendous potential to revolutionize how researchers understand and work with data on sensitive issues, including child abuse.”
Researchers studied data from 3,317 injury and abuse-related emergency department visits at seven children’s hospitals between February 2021 and December 2022. All children were under the age of 10 and nearly three quarters were under the age of two.
About this AI and child abuse research news
Author: PAS 2025
Source: Pediatric Academic Societies
Contact: PAS 2025 – Pediatric Academic Societies
Image: The image is credited to Neuroscience News
Original Research: The findings will be presented at the Pediatric Academic Societies (PAS) 2025 Meeting
Abstract
A Machine Learning Approach to Improve Estimation of Physical Abuse
Background
International Classification of Diseases, 10th Revision, Clinical Modification (ICD-10-CM) codes are inaccurate for determining child physical abuse (PA) prevalence, particularly in emergency department (ED) settings. Consideration of injury codes along with abuse-specific codes may enable more accurate PA prevalence estimates.
Objective
To develop a coding schema to better estimate PA using machine learning.
Design/Methods
We performed a secondary data analysis of children < 10 years evaluated by a child abuse pediatrician (CAP) due to concerns for PA during Feb 2021-Dec 2022 at 7 children’s hospitals contributing data to both CAPNET, a multicenter child abuse research network, and Pediatric Health Information System (PHIS). We excluded encounters not linked with PHIS and those not evaluated in the ED during the CAPNET encounter.
True PA was defined by CAP assigned rating 5- 7 on a 7-point scale of PA likelihood within the CAPNET database. Abuse-specific codes, including suspected codes, were defined as ICD-10-CM codes for PA modified from the Centers for Disease Control and Prevention child abuse and neglect syndromic surveillance definition. All 4-digit injury ICD-10-CM codes were used.
We developed LASSO logistic regression models to predict CAP¬ determined PA for encounters with and without abuse-specific codes and used the models to calculate site-specific estimates of PA prevalence. We calculated the estimation error for site estimates based on 1) abuse-specific codes alone and 2) our LASSO predictive models. Estimation error was defined as estimated PA prevalence minus CAP-determined PA prevalence (true value).
Results
3317 of 6178 CAPNET encounters were successfully linked with PHIS and seen in the ED. Median age was 8.4 months with 74% < 2 years and 59% < 1 year. CAP diagnosed PA in 35% (n=l145) of all encounters, 12.7% (n=240) of encounters without abuse-specific codes, and 63.4% (n=905) of encounters with abuse-specific codes.
At least one abuse-specific code was assigned for 43% of encounters. Site-specific estimates of PA prevalence based only on assignment of abuse-specific codes overestimated prevalence with estimation errors ranging from 2.0% to 14.3% (average absolute error 8.5%).
Estimates of site-specific PA prevalence based on our predictive models had reduced errors from -3.0% to 2.6% (average absolute error 1.8%) (Fig. 1). Absolute error decreased for 6 of 7 sites and increased by 0.6% for the remaining site (Fig. 2).
Conclusion(s)
Our predictive models more accurately estimated the prevalence of PA compared to abuse-specific codes alone.