Summary: Researchers developed an AI-based tool, MeAgainMeds.com, to help match patients with the most effective antidepressants based on their medical history. This free website aims to reduce the trial-and-error process in finding the right medication, improving patient outcomes.
By analyzing data from millions of patients, the AI provides evidence-based recommendations, which patients can discuss with their healthcare providers.
Key Facts:
- AI-Powered Matching: MeAgainMeds.com uses AI to match patients with the most effective antidepressant based on their medical history.
- Large-Scale Data Analysis: The tool analyzed data from over 3.6 million patients and 10.2 million antidepressant prescriptions to create its recommendations.
- Patient-Centered: The website does not require personal identifiable information and advises patients to consult their healthcare providers for medication changes.
Source: George Mason University
Researchers in George Mason University’s College of Public Health have leveraged the power of artificial intelligence (AI) analytical models to match a patient’s medical history to the most effective antidepressant, allowing patients to find symptom relief sooner.
The free website, MeAgainMeds.com, provides evidence-based recommendations, allowing clinicians and patients to find the optimal antidepressant the first time.
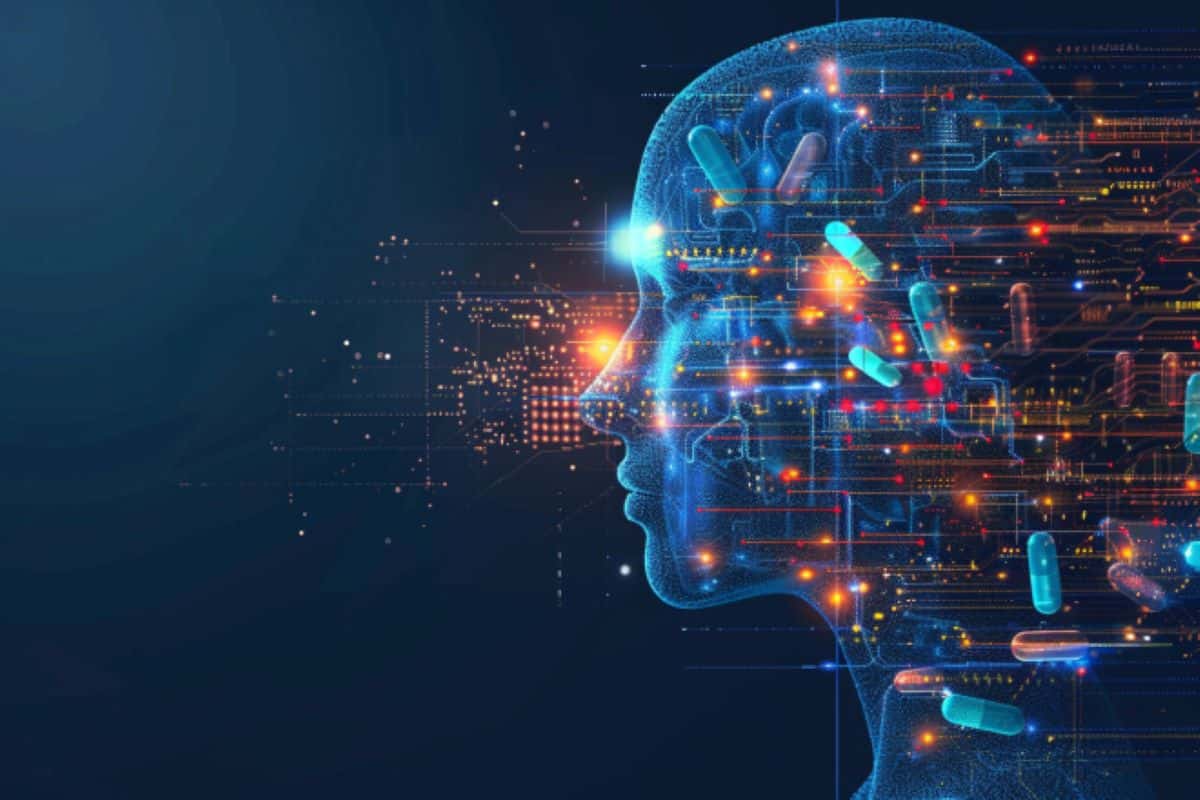
“Many people with depression must try multiple antidepressants before finding the right one that alleviates their symptoms. Our website reduces the number of medications that patients are asked to try.
“The system recommends to the patient what has worked for at least 100 other patients with the same exact relevant medical history,” said Farrokh Alemi, principal investigator and professor of health informatics at George Mason University’s College of Public Health.
AI helped to simplify the very complex task of making thousands of guidelines easily accessible to patients and clinicians. The guidelines that researchers created are complicated because of the amount of clinical information that is relevant in prescribing an antidepressant; AI seamlessly simplifies the task.
With AI at its core, MeAgainMeds.com analyzes clinician or patient responses to a few anonymous medical history questions to determine which oral antidepressant would best meet the specific needs. The website does not ask for any personal identifiable information and it does not prescribe medication changes. Patients are advised to visit their primary health care provider for any changes in medication.
In 2018, the Centers for Disease Control reported that more than 13% of adults use antidepressants, and the number has only increased since the pandemic and other epidemics since 2020. This website could help millions of people find relief more quickly.
Alemi and his team analyzed 3,678,082 patients who took 10,221,145 antidepressants. The oral antidepressants analyzed were amitriptyline, bupropion, citalopram, desvenlafaxine, doxepin, duloxetine, escitalopram, fluoxetine, mirtazapine, nortriptyline, paroxetine, sertraline, trazodone, and venlafaxine.
From the data, they created 16,770 subgroups of at least 100 cases, using reactions to prior antidepressants, current medication, history of physical illness, history of mental illness, key procedures, and other information. The subgroups and remission rates drive the AI to produce an evidence-based medication recommendation.
“By matching patients to the subgroups, clinicians can prescribe the medication that works best for people with similar medical history,” said Alemi. The researchers and website recommend that patients who use the site take the information to their clinicians, who will ultimately decide whether to prescribe the recommended medicine.
Alemi and his team tested a protype version of the site in 2023, which they advertised on social media. At that time, 1,500 patients used the website. Their goal is to improve the website and expand its user base. The initial research was funded by the Commonwealth of Virginia and by the Robert Wood Johnson Foundation.
The researchers’ most recent paper in a series of papers on response to antidepressants analyzed 2,467 subgroups of patients who had received psychotherapy.\
Additional authors include Tulay G Soylu from Temple University, and Mary Cannon and Conor McCandless from Royal College of Surgeons in Dublin, Ireland.
About this AI and psychopharmacology research
Author: Mary Cunningham
Source: George Mason University
Contact: Mary Cunningham – George Mason University
Image: The image is credited to Neuroscience News
Original Research: Closed access.
“Effectiveness of Antidepressants in Combination with Psychotherapy” by Farrokh Alemi et al. The Journal of Mental Health Policy and Economics
Abstract
Effectiveness of Antidepressants in Combination with Psychotherapy
Background: Consensus-guidelines for prescribing antidepressants recommend that clinicians should be vigilant to match antidepressants to patient’s medical history but provide no specific advice on which antidepressant is best for a given medical history.
Aims of the study: For patients with major depression who are in psychotherapy, this study provides an empirically derived guideline for prescribing antidepressant medications that fit patients’ medical history.
Methods: This retrospective, observational, cohort study analyzed a large insurance database of 3,678,082 patients. Data was obtained from healthcare providers in the U.S. between January 1, 2001, and December 31, 2018. These patients had 10,221,145 episodes of antidepressant treatments.
This study reports the remission rates for the 14 most commonly prescribed single antidepressants (amitriptyline, bupropion, citalopram, desvenlafaxine, doxepin, duloxetine, escitalopram, fluoxetine, mirtazapine, nortriptyline, paroxetine, sertraline, trazodone, and venlafaxine) and a category named “Other” (other antidepressants/combination of antidepressants).
The study used robust LASSO regressions to identify factors that affected remission rate and clinicians’ selection of antidepressants. The selection bias in observational data was removed through stratification.
We organized the data into 16,770 subgroups, of at least 100 cases, using the combination of the largest factors that affected remission and selection bias. This paper reports on 2,467 subgroups of patients who had received psychotherapy.
Results: We found large, and statistically significant, differences in remission rates within subgroups of patients. Remission rates for sertraline ranged from 4.5% to 77.86%, for fluoxetine from 2.86% to 77.78%, for venlafaxine from 5.07% to 76.44%, for bupropion from 0.5% to 64.63%, for desvenlafaxine from 1.59% to 75%, for duloxetine from 3.77% to 75%, for paroxetine from 6.48% to 68.79%, for escitalopram from 1.85% to 65%, and for citalopram from 4.67% to 76.23%.
Clearly these medications are ideal for patients in some subgroups but not others. If patients are matched to the subgroups, clinicians can prescribe the medication that works best in the subgroup. Some medications (amitriptyline, doxepin, nortriptyline, and trazodone) always had remission rates below 11% and therefore were not suitable as single antidepressant therapy for any of the subgroups.
Discussions: This study provides an opportunity for clinicians to identify an optimal antidepressant for their patients, before they engage in repeated trials of antidepressants.
Implications for health care provision and use: To facilitate the matching of patients to the most effective antidepressants, this study provides access to a free, non-commercial, decision aid at http://MeAgainMeds.com.
Implications for health policies: Policymakers should evaluate how study findings can be made available through fragmented electronic health records at point-of-care. Alternatively, policymakers can put in place an AI system that recommends antidepressants to patients online, at home, and encourages them to bring the recommendation to their clinicians at their next visit.
Implications for further research: Future research could investigate (i) the effectiveness of our recommendations in changing clinical practice, (ii) increasing remission of depression symptoms, and (iii) reducing cost of care. These studies need to be prospective but pragmatic. It is unlikely random clinical trials can address the large number of factors that affect remission.