Summary: A recent study offers new insights into how brain regions coordinate during rest, using resting-state fMRI (rsfMRI) and neural recordings in mice. By comparing blood flow patterns with direct neural activity, researchers found that some brain activity remains “invisible” in traditional rsfMRI scans. This hidden activity suggests that current brain imaging techniques may miss key elements of neural behavior.
The findings, potentially applicable to human studies, may refine our understanding of brain networks. Further research could improve the accuracy of interpreting brain activity.
Key Facts:
- rsfMRI measures brain activity by tracking blood flow changes but may miss “invisible” neural signals.
- Simultaneous neural recordings revealed mismatches in spatial and temporal alignment with rsfMRI.
- Findings suggest a new component in brain activity interpretation, potentially aiding human studies.
Source: Penn State
To understand how different regions of the brain work together, researchers use a method called resting-state functional magnetic resonance imaging (rsfMRI). The method measures brain activity by observing changes in blood flow to different parts of the brain; however, rsfMRI does not explain how these blood flow changes to different brain regions relate to what is happening with the brain’s neurons — cells that send and receive messages in the form of electronic signals.
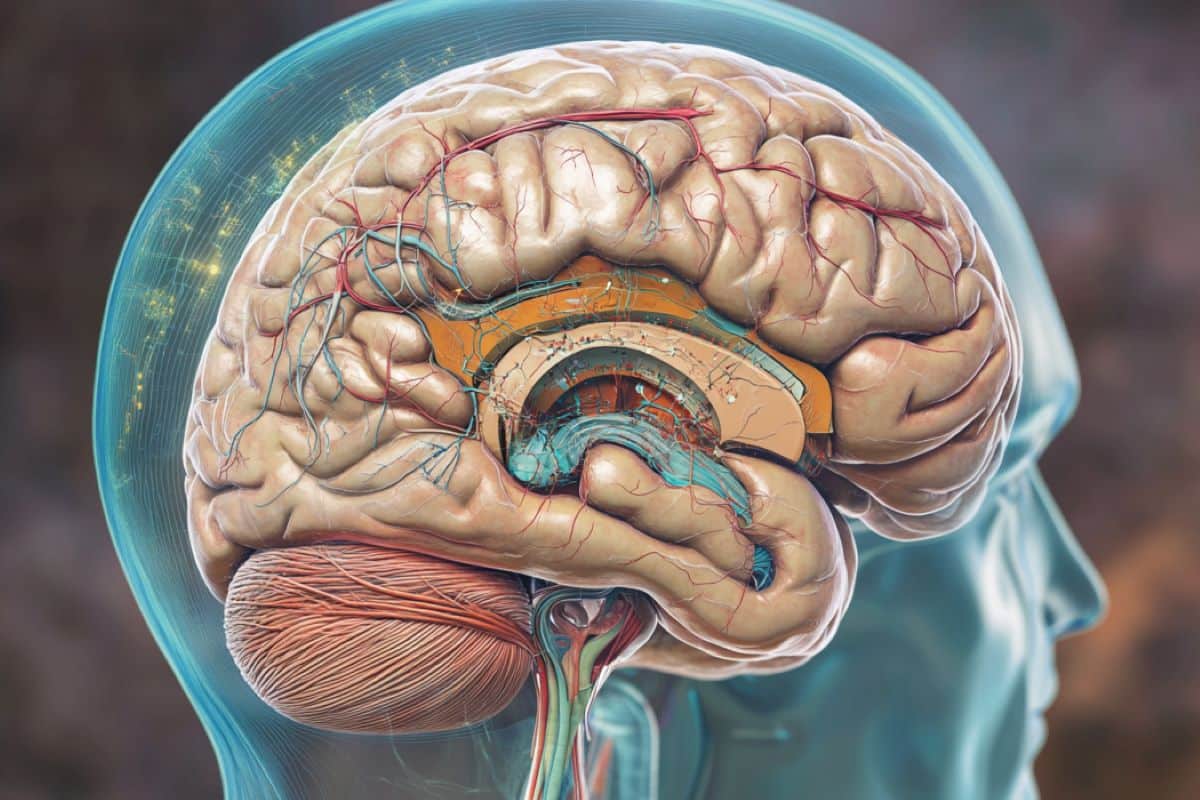
A team of researchers led by Nanyin Zhang, the Dorothy Foehr Huck and J. Lloyd Huck Chair in Brain Imaging and professor of biomedical engineering at Penn State, set out to answer this question. They recently published their findings, made in mice, in the journal eLife.
Penn State News sat down with Zhang, who is also affiliated with the electrical engineering and the engineering science and mechanics departments, as well as the Huck Institutes of the Life Sciences, to learn more about his findings.
Q: How does rsfMRI work? What can it tell researchers, and what are its limitations?
Zhang: Resting-state functional magnetic resonance imaging (rsfMRI) allows scientists to study how different parts of the brain work together. This method shows us when different parts of the brain are active together by looking at their spontaneous changes in blood flow.
We call these coordinated patterns “resting-state brain networks” (RSNs). Even though we use these RSNs in many situations, we still don’t fully understand how these blood flow changes are related to what’s happening to neural activities in the brain. Lack of this knowledge highlights a significant gap in our understanding of functional brain networks.
Q: How did you address the limitations of rsfMRI?
Zhang: We aimed to address the question of how RSNs and rsfMRI relate to spontaneous neural activity. We used a technique that involves simultaneous recordings of rsfMRI and electrophysiology signals, which provides the measurement of neural activity and the rsfMRI signal from the same brain site at the same time.
By measuring these two signals at the same time, we hoped to elucidate exactly how spontaneous blood flow changes in the brain are related to neural activities.
Q: What were your findings? What does this new understanding about “invisible” signaling tell us?
Zhang: We found a disparity in the spatial and temporal relationships between the electrophysiology signal, which directly measures neural activity, and the rsfMRI signal.
The brain-wide RSN connectivity spatial patterns revealed by the rsfMRI signal can be recapitulated by the electrophysiology signal. However, these two types of signals do not align well over time.
These seemingly paradoxical findings imply that there are electrophysiology “invisible signals” contributing to the rsfMRI signal, which expands the conventional viewpoint on the relationship of neural activity and rsfMRI signal.
The conventional view believes the electrophysiology signal underlies most of the rsfMRI signal, whereas our results suggest that a major source of the rsfMRI signal might actually originate from an electrophysiology-invisible component.
Q: What are the implications of these findings for studying brains and brain imaging?
Zhang: It is generally believed that the rsfMRI signal can be explained using the electrophysiology signal. The possibility that RSNs may arise from electrophysiology-invisible brain activities that play a significant role in regulating rsfMRI signals suggests our understanding of the neural basis of the rsfMRI signal, and hence the interpretation of RSNs, might not be adequate.
Therefore, it is important to continue to investigate the neural basis of the rsfMRI signal to make sure we are accurately interpreting brain activity.
Q: How does rsfMRI in animals inform research in humans?
Zhang: The neural mechanism of rsfMRI in animals is very likely the same as that in humans. The findings in the current study might have high translational value to human rsfMRI studies.
Funding: The National Institute of Neurological Disorders and Stroke and the National Institute of Mental Health funded this work.
About this neuroimaging and brain mapping research news
Author: Sarah Small
Source: Penn State
Contact: Sarah Small – Penn State
Image: The image is credited to Neuroscience News
Original Research: Open access.
“Disparity in temporal and spatial relationships between resting-state electrophysiological and fMRI signals” by Nanyin Zhang et al. eLife
Abstract
Disparity in temporal and spatial relationships between resting-state electrophysiological and fMRI signals
Resting-state brain networks (RSNs) have been widely applied in health and disease, but the interpretation of RSNs in terms of the underlying neural activity is unclear.
To address this fundamental question, we conducted simultaneous recordings of whole-brain resting-state functional magnetic resonance imaging (rsfMRI) and electrophysiology signals in two separate brain regions of rats.
Our data reveal that for both recording sites, spatial maps derived from band-specific local field potential (LFP) power can account for up to 90% of the spatial variability in RSNs derived from rsfMRI signals.
Surprisingly, the time series of LFP band power can only explain to a maximum of 35% of the temporal variance of the local rsfMRI time course from the same site. In addition, regressing out time series of LFP power from rsfMRI signals has minimal impact on the spatial patterns of rsfMRI-based RSNs.
This disparity in the spatial and temporal relationships between resting-state electrophysiology and rsfMRI signals suggests that electrophysiological activity alone does not fully explain the effects observed in the rsfMRI signal, implying the existence of an rsfMRI component contributed by ‘electrophysiology-invisible’ signals.
These findings offer a novel perspective on our understanding of RSN interpretation.