Summary: Researchers cook up a neuromorphic brain-mimicking processing system with a blend of circuits and memristive devices.
Source: American Institute of Physics
During the 1990s, Carver Mead and colleagues combined basic research in neuroscience with elegant analog circuit design in electronic engineering. This pioneering work on neuromorphic electronic circuits inspired researchers in Germany and Switzerland to explore the possibility of reproducing the physics of real neural circuits by using the physics of silicon.
The field of “brain-mimicking” neuromorphic electronics shows great potential not only for basic research but also for commercial exploitation of always-on edge computing and “internet of things” applications.
In Applied Physics Letters, from AIP Publishing, Elisabetta Chicca, from Bielefeld University, and Giacomo Indiveri, from the University of Zurich and ETH Zurich, present their work to understand how neural processing systems in biology carry out computation, as well as a recipe to reproduce these computing principles in mixed signal analog/digital electronics and novel materials.
One of the most distinctive computational features of neural networks is learning, so Chicca and Indiveri are particularly interested in reproducing the adaptive and plastic properties of real synapses. They used both standard complementary metal-oxide semiconductor (CMOS) electronic circuits and advanced nanoscale memory technologies, such as memristive devices¬, to build intelligent systems that can learn.
This work is significant, because it can lead to a better understanding of how to implement sophisticated signal processing using extremely low-power and compact devices.
Their key findings are that the apparent disadvantages of these low-power computing technologies, mainly related to low precision, high sensitivity to noise and high variability, can actually be exploited to perform robust and efficient computation, very much like the brain can use highly variable and noisy neurons to implement robust behavior.
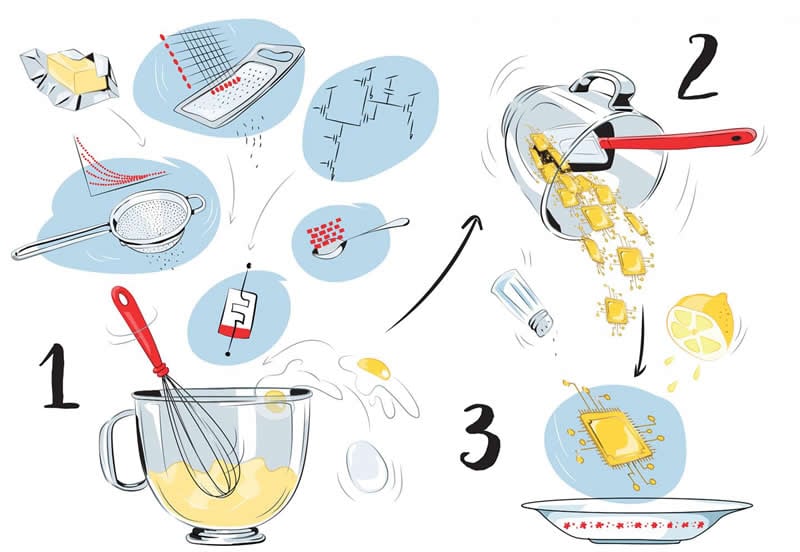
The researchers said it is surprising to see the field of memory technologies, typically concerned with bit-precise high-density device technologies, now looking at animal brains as a source of inspiration for understanding how to build adaptive and robust neural processing systems. It is very much in line with the basic research agenda that Mead and colleagues were following more than 30 years ago.
“The electronic neural processing systems that we build are not intended to compete with the powerful and accurate artificial intelligence systems that run on power-hungry large computer clusters for natural language processing or high-resolution image recognition and classification,” said Chicca.
In contrast, their systems “offer promising solutions for those applications that require compact and very low-power (submilliwatt) real-time processing with short latencies,” Indiveri said.
He said examples of such applications fall within “the ‘extreme-edge computing’ domain, which require a small amount of artificial intelligence to extract information from live or streaming sensory signals, such as for bio-signal processing in wearable devices, brain-machine interfaces and always-on environmental monitoring.”
Source:
American Institute of Physics
Media Contacts:
Larry Frum – American Institute of Physics
Image Source:
The image is credited to Elisabetta Chicca.
Original Research: Open access
“A recipe for creating ideal hybrid memristive-CMOS neuromorphic computing systems”. Elisabetta Chicca and Giacomo Indiveri.
Applied Physics Letters doi:10.1063/1.5142089.
Abstract
A recipe for creating ideal hybrid memristive-CMOS neuromorphic computing systems
The development of memristive device technologies has reached a level of maturity to enable the design and fabrication of complex and large-scale hybrid memristive-Complementary Metal-Oxide Semiconductor (CMOS) neural processing systems. These systems offer promising solutions for implementing novel in-memory computing architectures for machine learning and data analysis problems. We argue that they are also ideal building blocks for integration in neuromorphic electronic circuits suitable for ultra-low power brain-inspired sensory processing systems, therefore leading to innovative solutions for always-on edge-computing and Internet-of-Things applications. Here, we present a recipe for creating such systems based on design strategies and computing principles inspired by those used in mammalian brains. We enumerate the specifications and properties of memristive devices required to support always-on learning in neuromorphic computing systems and to minimize their power consumption. Finally, we discuss in what cases such neuromorphic systems can complement conventional processing ones and highlight the importance of exploiting the physics of both the memristive devices and the CMOS circuits interfaced to them.