Summary: Using AI to analyze language associated with depression on social media during the first wave of the COVID-19 pandemic, researchers found people were more resilient than previously thought.
Source: University of Alberta
Researchers who analyzed language related to depression on social media during the pandemic say the data suggest people learned to cope as the waves wore on.
University of Alberta researcher Alona Fyshe and her collaborators at the University of Western Ontario hypothesized that depression-related language would spike during each wave of COVID-19. But their study shows that wasn’t the case.
“There was a big reaction at the beginning and then people sort of found their new normal,” says Fyshe, an assistant professor of computing science and psychology. “It’s a message of resilience, people figuring out how to keep on keeping on in a pandemic.”
For the study, the researchers turned their attention to online platforms such as Reddit and Twitter. Social media is a useful tool in assessing mental health at the population level, explains Fyshe, a fellow of the Alberta Machine Intelligence Institute and Canada CIFAR AI chair.
The researchers first identified keywords by analyzing the type of language posters were using in discussions on Reddit. The self-identification found in those subreddits and forums isn’t replicated in many other social media platforms, Fyshe explains.
“Essentially we trained a machine learning model that can differentiate between the language of people who post to a thread on the topic of depression versus people who don’t,” says Fyshe.
Using this information and the identified keywords, they turned their attention to Twitter. They analyzed data from four cities — Sydney, Mumbai, Seattle and Toronto – with different waves of COVID-19 so they could determine which changes in language were due to global trends and which were local. They restricted the data to areas with a large percentage of English tweets so they could use the same methodology to analyze all the data.
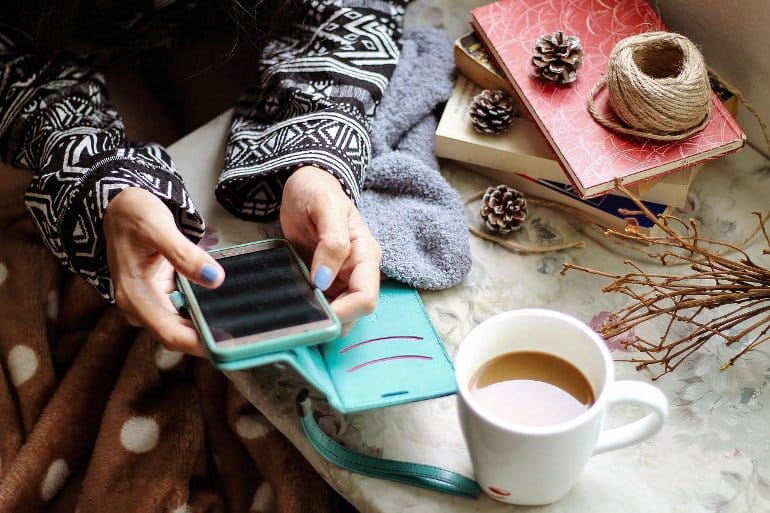
The results were surprising, says Fyshe. In general, spikes in COVID-19 cases and the various waves throughout the pandemic weren’t reflected in the data. In fact, the only city with an increase in depression-related language after the first wave was Mumbai, which saw a significant second wave.
Fyshe says the machine learning methods used to scrape Reddit subforums to identify keywords and analyze Twitter data could be applied to a wide range of subjects. For example, when examining data in Seattle, they found strong reactions to the Black Lives Matter movement.
“It was indicative of there being a large change to the general mood — what people were talking about and how people were feeling about the world they lived in.”
About this language and depression research news
Author: Ross Neitz
Source: University of Alberta
Contact: Ross Neitz – University of Alberta
Image: The image is in the public domain
Original Research: Open access.
“Quantifying Depression-Related Language on Social Media During the COVID-19 Pandemic” by Alona Fyshe et al. International Journal of Population Data Science
Abstract
Quantifying Depression-Related Language on Social Media During the COVID-19 Pandemic
Introduction
The COVID-19 pandemic had clear impacts on mental health. Social media presents an opportunity for assessing mental health at the population level.
Objectives
1) Identify and describe language used on social media that is associated with discourse about depression. 2) Describe the associations between identified language and COVID-19 incidence over time across several geographies.
Methods
We create a word embedding based on the posts in Reddit’s /r/Depression and use this word embedding to train representations of active authors. We contrast these authors against a control group and extract keywords that capture differences between the two groups. We filter these keywords for face validity and to match character limits of an information retrieval system, Elasticsearch. We retrieve all geo-tagged posts on Twitter from April 2019 to June 2021 from Seattle, Sydney, Mumbai, and Toronto. The tweets are scored with BM25 using the keywords. We call this score rDD. We compare changes in average score over time with case counts from the pandemic’s beginning through June 2021.
Results
We observe a pattern in rDD across all cities analyzed: There is an increase in rDD near the start of the pandemic which levels off over time. However, in Mumbai we also see an increase aligned with a second wave of cases.
Conclusions
Our results are concordant with other studies which indicate that the impact of the pandemic on mental health was highest initially and was followed by recovery, largely unchanged by subsequent waves. However, in the Mumbai data we observed a substantial rise in rDD with a large second wave. Our results indicate possible un-captured heterogeneity across geographies, and point to a need for a better understanding of this differential impact on mental health.