Summary: Researchers have developed a neural network based AI system that can decode and predict what a person is seeing or imagining.
Source: Kyoto University.
Kyoto scientists enhance mind reading technology.
Scanning your brain to decode the contents of your mind has been a subject of intense research interest for some time. As studies have progressed, scientists have gradually been able to interpret what test subjects see, remember, imagine, and even dream.
There have been significant limitations, however, beginning with a necessity to extensively catalog each subject’s unique brain patterns, which are then matched with a small number of pre-programmed images. These procedures require that subjects undergo lengthy and expensive fMRI testing.
Now a team of researchers in Kyoto has used neural network-based artificial intelligence to decode and predict what a person is seeing or imagining, referring to a significantly larger catalog of images. Their results are reported in Nature Communications.
“When we gaze at an object, our brains process these patterns hierarchically, starting with the simplest and progressing to more complex features,” explains team leader Yukiyasu Kamitani of Kyoto University.
“The AI we used works on the same principle. Named ‘Deep Neural Network’, or DNN, it was trained by a group now at Google.”
The team from Kyoto University and ATR (Advanced Telecommunications Research) Computational Neuroscience Laboratories discovered that brain activity patters can be decoded, or translated, into signal patterns of simulated neurons in the DNN when both are shown the same image.
Additionally, the researchers found that lower and higher visual areas in the brain were better at decoding respective layers of the DNN, revealing a homology between the human brain and the neural network.
“We tested whether a DNN signal pattern decoded from brain activity can be used to identify seen or imagined objects from arbitrary categories,” explains Kamitani. “The decoder takes neural network patterns and compares these with image data from a large database. Sure enough, the decoder could identify target objects with high probability.”
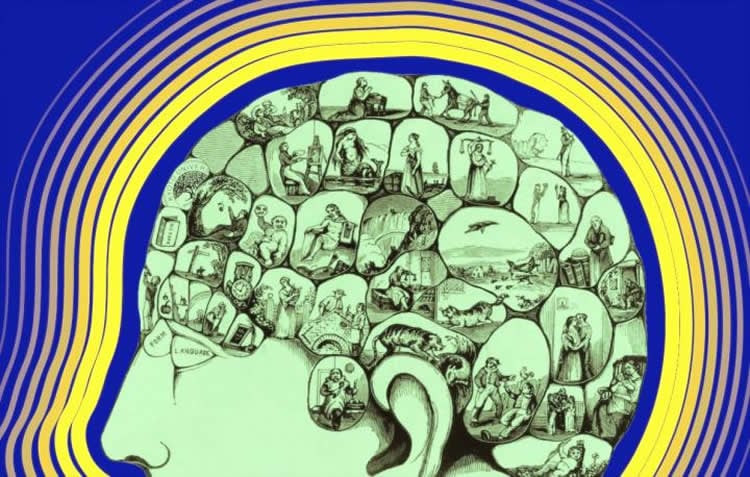
As brain decoding and AI development advance, Kamitani hopes to improve the image identification accuracy of their technique. He concludes, “Bringing AI research and brain science closer together could open the door to new brain-machine interfaces, perhaps even bringing us closer to understanding consciousness itself.”
Funding: New Energy and Industrial Technology Development Organization, Japan Society for the Promotion of Science, Japan Science and Technology Agency funded this research.
Source: Raymond Kunikane Terhune – Kyoto University
Image Source: NeuroscienceNews.com image is credited to Kyoto University.
Original Research: Full open access research for “Generic decoding of seen and imagined objects using hierarchical visual features” by Tomoyasu Horikawa & Yukiyasu Kamitani in Nature Communications. Published online May 22 2017 doi:10.1038/ncomms15037
[cbtabs][cbtab title=”MLA”]Kyoto University “Take A Look and You’ll See Into Your Imagination.” NeuroscienceNews. NeuroscienceNews, 1 June 2017.
<https://neurosciencenews.com/fmri-imagination-6814/>.[/cbtab][cbtab title=”APA”]Kyoto University (2017, June 1). Take A Look and You’ll See Into Your Imagination. NeuroscienceNew. Retrieved June 1, 2017 from https://neurosciencenews.com/fmri-imagination-6814/[/cbtab][cbtab title=”Chicago”]Kyoto University “Take A Look and You’ll See Into Your Imagination.” https://neurosciencenews.com/fmri-imagination-6814/ (accessed June 1, 2017).[/cbtab][/cbtabs]
Abstract
Generic decoding of seen and imagined objects using hierarchical visual features
Object recognition is a key function in both human and machine vision. While brain decoding of seen and imagined objects has been achieved, the prediction is limited to training examples. We present a decoding approach for arbitrary objects using the machine vision principle that an object category is represented by a set of features rendered invariant through hierarchical processing. We show that visual features, including those derived from a deep convolutional neural network, can be predicted from fMRI patterns, and that greater accuracy is achieved for low-/high-level features with lower-/higher-level visual areas, respectively. Predicted features are used to identify seen/imagined object categories (extending beyond decoder training) from a set of computed features for numerous object images. Furthermore, decoding of imagined objects reveals progressive recruitment of higher-to-lower visual representations. Our results demonstrate a homology between human and machine vision and its utility for brain-based information retrieval.
“Generic decoding of seen and imagined objects using hierarchical visual features” by Tomoyasu Horikawa & Yukiyasu Kamitani in Nature Communications. Published online May 22 2017 doi:10.1038/ncomms15037