Summary: Researchers have combined artificial intelligence and EEG brain activity data to better understand the Other-Race Effect (ORE), where people recognize faces of their own race more accurately than others. Studies revealed that participants processed other-race faces with less neural detail, seeing them as more average, younger, and more expressive.
This lack of distinct processing contributes to difficulties in recognition and may reinforce implicit biases. The findings have real-world implications, from improving facial recognition software and eyewitness testimony to helping address social bias and advancing mental health diagnostics.
Key Facts:
- Reduced Neural Detail: Brain activity shows other-race faces are processed more generally, contributing to poor recognition.
- Perceived Differences: Participants mentally reconstructed other-race faces as more average, younger, and more expressive.
- Potential Applications: Insights could help reduce social bias, refine facial recognition technology, and aid mental health diagnosis.
Source: University of Toronto
U of T Scarborough researchers have harnessed artificial intelligence (AI) and brain activity to shed new light on why we struggle to accurately recognize faces of people from different races.
Across a pair of studies, researchers explored the Other-Race-Effect (ORE), a well-known phenomenon in which people recognize faces of their own race more easily than others.
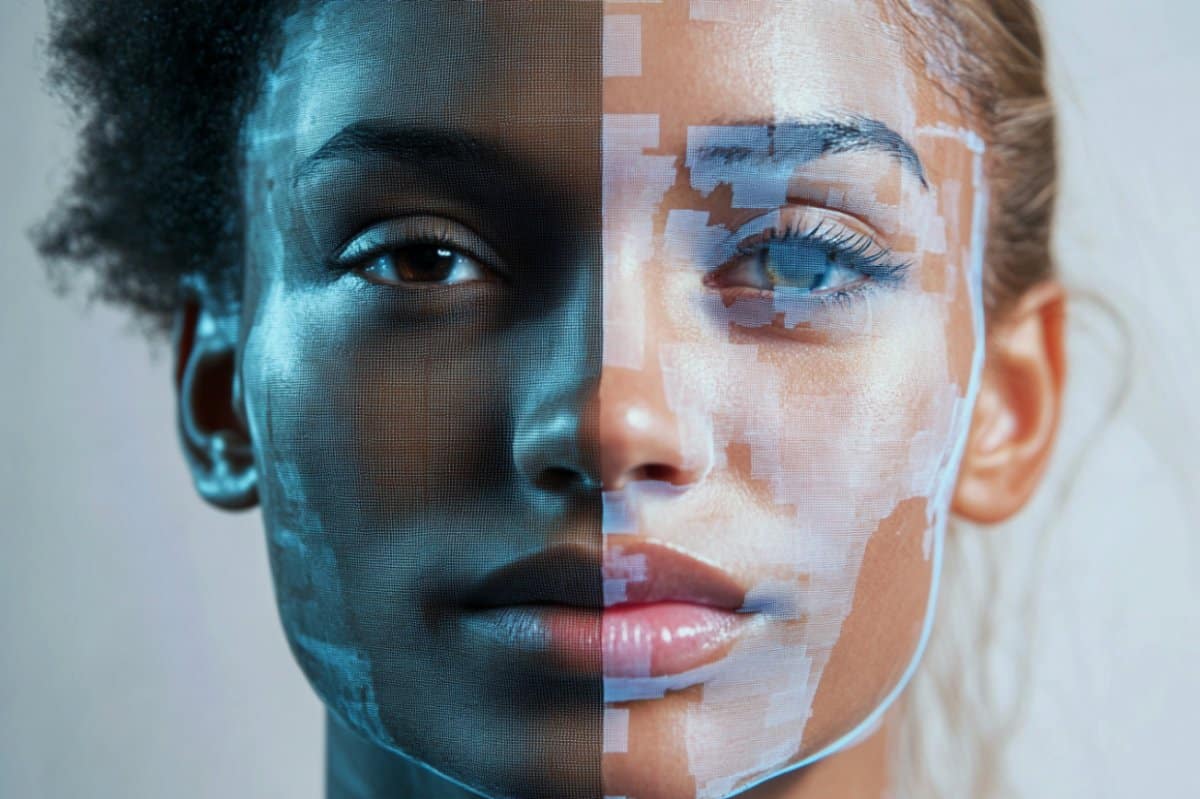
They combined AI and brain activity collected through EEG (electroencephalography) to reveal new insights into how we perceive other-race faces, including visual distortions more deeply ingrained in our brain than previously thought.
“What we found was striking — people are so much better at seeing the facial details of people from their own race,” says Adrian Nestor, associate professor in the Department of Psychology and co-author of the studies.
“This is important because we should want to know why we have trouble recognizing faces from other races, and what influence that might have on behaviour.”
In one study, published earlier this year in the journal Behavior Research Methods, the researchers used generative AI to look at individual responses to seeing images of faces.
Two groups of participants (one East Asian, one white) were shown a series of faces on a computer screen and asked to rate them based on similarity.
The researchers were able to generate visual representations of faces using a generative adversarial network (GAN), a type of AI that can be trained to create life-like images.
Using the GAN’s image generating ability, the researchers were able to see the mental images the study participants had of faces.
They discovered that faces from the same race were reconstructed more accurately than those from different races, and that people tend to see faces of other races as more average looking.
One surprising finding was that faces from other races, when reconstructed, also appear younger.
What’s happening in the brain
A second study, recently published in the journal Frontiers, looked more closely at brain activity that might be involved to explain ORE.
Brain activity, which occurs in the first 600 milliseconds of seeing the images, was used to digitally reconstruct how the participants visually process faces in their mind.
If it sounds like mind-reading, it kind of is. Nestor’s lab first showed the potential of harnessing EEG for visual perception back in 2018. Since then the algorithms they used have improved significantly.
Using EEG data, researchers found that the brain processes faces from the same race and faces from different races in distinct ways. Neural recordings associated with visual perception showed less differentiation for other-race faces.
“When it comes to other-race faces, the brain responses were less distinct, indicating that these faces are processed more generally and with less detail,” says Moaz Shoura, a PhD student in Nestor’s lab and co-author of the studies.
“This suggests that our brains tend to group other-race faces together, leading to less accurate recognition and reinforcing ORE.”
One of the most intriguing findings from this study was that other-race faces appeared not just more average-looking, but also younger and more expressive in the minds of the participants, even when they weren’t.
“This could explain why people often have difficulty recognizing faces from other races. The brain isn’t processing facial appearance as distinctly and accurately,” says Nestor.
Potential real-world applications
The research, which received funding from a Natural Sciences and Engineering Research Council of Canada (NSERC) grant, might have far-reaching implications.
Nestor says it could open up possibilities for understanding how bias forms in the brain. It could also be used to improve facial recognition software, gather more accurate eyewitness testimony, or even as a diagnostic tool for mental health disorders such as schizophrenia or borderline personality disorder.
“It’s important to know exactly how people experience distortions in their emotional perception,” says Nestor.
For example, he says by seeing exactly what’s going on in a person’s mind who has trouble perceiving disgust or who misinterprets positive emotions as negative ones, it can help with diagnosing mental health disorders and with developing treatments.
Shoura adds that by further exploring the effect of perceptual bias, it might help in a range of social situations, from job interviews to combating racial bias.
“If we can better understand how the brain processes faces, we can develop strategies to reduce the impact bias can have when we first meet face-to-face with someone from another race.”
About this facial recognition and AI research news
Author: Suniya Kukaswadia
Source: University of Toronto
Contact: Suniya Kukaswadia – University of Toronto
Image: The image is credited to Neuroscience News
Original Research: Closed access.
“Unraveling other‑race face perception with GAN‑based image reconstruction” by Adrian Nestor et al. Behavior Research Methods
Abstract
Unraveling other‑race face perception with GAN‑based image reconstruction
The other-race effect (ORE) is the disadvantage of recognizing faces of another race than one’s own. While its prevalence is behaviorally well documented, the representational basis of ORE remains unclear.
This study employs StyleGAN2, a deep learning technique for generating photorealistic images to uncover face representations and to investigate ORE’s representational basis.
To this end, we collected pairwise visual similarity ratings with same- and other-race faces across East Asian and White participants exhibiting robust levels of ORE.
Leveraging the significant overlap in representational similarity between the GAN’s latent space and perceptual representations in human participants, we designed an image reconstruction approach aiming to reveal internal face representations from behavioral similarity data.
This methodology yielded hyper-realistic depictions of face percepts, with reconstruction accuracy well above chance, as well as an accuracy advantage for same-race over other-race reconstructions, which mirrored ORE in both populations.
Further, a comparison of reconstructions across participant race revealed a novel age bias, with other-race face reconstructions appearing younger than their same-race counterpart.
Thus, our work proposes a new approach to exploiting the utility of GANs in image reconstruction and provides new avenues in the study of ORE.