Summary: It may be possible to optimize the stimulation parameters of brain implants in animals without human intervention. The study highlights the potential for autonomous optimization of prostheses implanted in the brain. The advance may prove to be beneficial for those with spinal cord injury and diseases that affect movement.
Source: University of Montreal
Scientists have long studied neurostimulation to treat paralysis and sensory deficits caused by strokes and spinal cord injuries, which in Canada affect some 380,000 people across the country.
A new study published in the journal Cell Reports Medicine demonstrates the possibility of autonomously optimizing the stimulation parameters of prostheses implanted in the brains of animals, without human intervention.
The work was done at Université de Montréal by neuroscience professors Marco Bonizzato, Numa Dancause and Marina Martinez, in collaboration with mathematics professor and Mila researcher Guillaume Lajoie.
The study grew out of an important interdisciplinary collaboration between researchers who combine expertise in neuroscience and artificial intelligence, two fields of expertise in which the UdeM stands out internationally.
‘A very promising phase’
“Neuroprostheses—devices designed to restore connections between neurons following a loss of motor function—are entering a very promising phase of their development,” said Lajoie. “We are demonstrating the benefits obtained by autonomously optimizing their parameters.”
If the performance of these prostheses has increased, it’s thanks to the autonomous learning algorithms put forward by the researchers, added Bonizzato.
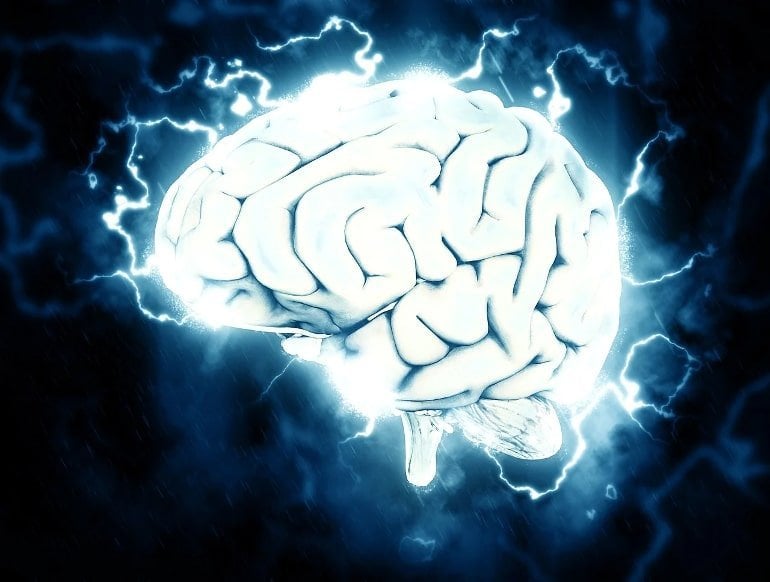
“Optimization algorithms allow us to design very refined neurostimulation protocols and personalize treatments according to the condition of each patient.”
For his part, Dancause believes that although “there are several ways of stimulating the brain, the contribution of artificial intelligence is essential to make the most of the data collected and anticipate conditions that do not yet exist.”
With these technological advances, scientists are closer to finding new neuroprosthetic solutions to improve the treatment of pathologies such as spinal cord injuries and strokes, or deep brain stimulation through neuromodulation to treat conditions such as Parkinson’s disease.
About this neurotech research news
Author: Press Office
Source: University of Montreal
Contact: Press Office – University of Montreal
Image: The image is in the public domain
Original Research: Open access.
“Autonomous optimization of neuroprosthetic stimulation parameters that drive the motor cortex and spinal cord outputs in rats and monkeys” by Marco Bonizzato et al. Cell Reports Medicine
Abstract
Autonomous optimization of neuroprosthetic stimulation parameters that drive the motor cortex and spinal cord outputs in rats and monkeys
Highlights
- Autonomous learning algorithm optimizes complex neuromodulation patterns in vivo
- It enables “intelligent” neuroprostheses, immediately alleviating motor deficits
- The application is robust to changes, e.g., due to plasticity or interface failure
- Knowledge transfer to experts/clinicians is supported with an open-source framework
Summary
Neural stimulation can alleviate paralysis and sensory deficits. Novel high-density neural interfaces can enable refined and multipronged neurostimulation interventions. To achieve this, it is essential to develop algorithmic frameworks capable of handling optimization in large parameter spaces.
Here, we leveraged an algorithmic class, Gaussian-process (GP)-based Bayesian optimization (BO), to solve this problem. We show that GP-BO efficiently explores the neurostimulation space, outperforming other search strategies after testing only a fraction of the possible combinations.
Through a series of real-time multi-dimensional neurostimulation experiments, we demonstrate optimization across diverse biological targets (brain, spinal cord), animal models (rats, non-human primates), in healthy subjects, and in neuroprosthetic intervention after injury, for both immediate and continual learning over multiple sessions. GP-BO can embed and improve “prior” expert/clinical knowledge to dramatically enhance its performance.
These results advocate for broader establishment of learning agents as structural elements of neuroprosthetic design, enabling personalization and maximization of therapeutic effectiveness.