Summary: A new physiological test relies on an algorithm that analyzes metabolites in blood samples to predict whether a person has an ASD diagnosis. The algorithm was 94.7% effective in determining autism diagnosis and was slightly more reliable when co-occurring conditions, such as gastrointestinal disorders, were taken into account.
Source: Rensselaer Polytechnic Institute
Developing a physiological test for diagnosing autism spectrum disorder (ASD), one that measures certain components in the blood, has the potential to be a paradigm shift for diagnosing ASD. However, the large heterogeneity of how ASD affects individuals has long been viewed as a key obstacle to the development of such a test.
Research conducted at Rensselaer Polytechnic Institute, and published online today in the journal Research in Autism Spectrum Disorders, represents a significant step toward addressing this challenge.
The research, led by Juergen Hahn, the head of the Department of Biomedical Engineering at Rensselaer, builds upon his team’s previous discoveries, including the development of a physiological test for autism.
That physiological test relies on an algorithm that analyzes measurements of metabolites in a blood sample to predict whether or not a person has an ASD diagnosis.
Hahn and his team sought to assess the strength of their algorithm even further, by testing it with data collected from children with ASD who also have one or more other conditiosn — so called co-occurring conditions — like allergies or gastrointestinal symptoms.
“We wanted to see if the results from our previous analysis still hold up even in the presence or absence of a number of co-occurring conditions,” said Hahn, who is also a member of the Center for Biotechnology & Interdisciplinary Studies at Rensselaer. “We found that, for the conditions that we looked at, the accuracy of the prediction results was only minimally affected by the presence of co-occurring conditions.”
The model, according to the research, was able to successfully identify 124 of 131 children with ASD — 94.7% — regardless of whether or not the child also had a co-occurring condition. In general, Hahn said, the algorithm actually worked slightly better when a co-occurring condition was present. The reason for that finding, he said, needs to be examined further.
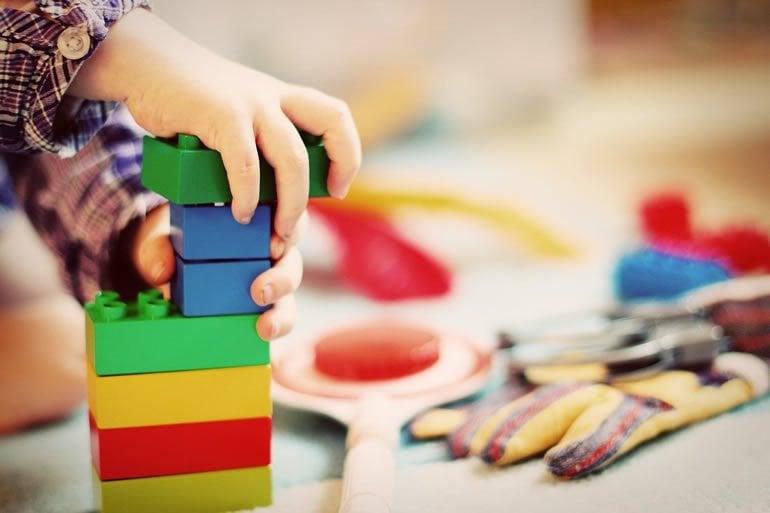
Hahn’s big data approach to uncovering new insights about autism has also been used to examine the effectiveness of possible treatments, and the idea that ASD — although extremely heterogonous — may have some subgroups.
He and his team use depersonalized medical data to perform these analyses, and continue to dig deeper into their model and its abilities as more data becomes available.
“This was a question we definitely had to answer as many individuals with ASD have one or more co-occurring condition,” Hahn said. “These findings will also guide some of our future research.”
About this autism research article
Source:
Rensselaer Polytechnic Institute
Contacts:
Reeve Hamilton – Rensselaer Polytechnic Institute
Image Source:
The image is in the public domain.
Original Research: Closed access
“Classification of autism spectrum disorder from blood metabolites: Robustness to the presence of co-occurring conditions” by Juergen Hahn et al. Research in Autism Spectrum Disorders.
Abstract
Classification of autism spectrum disorder from blood metabolites: Robustness to the presence of co-occurring conditions
Background
Previous studies have found plasma measurements of metabolites from the folate-dependent one-carbon metabolism (FOCM) and transsulfuration (TS) pathways to be useful for differentiating individuals with autism spectrum disorder (ASD) from their typically developing peers. However, ASD is heterogeneous due to wide variation in the presence of co-occurring behavioral and medical conditions, and it is unknown how these conditions influence the ability to identify ASD based on FOCM/TS metabolites.
Method
This study employs a previously developed multivariate model that makes use of five FOCM/TS measurements (S-adenosylmethionine/S-adenosylhomocysteine, glutamylcysteine, glutathione disulfide, free cystine/free cysteine, and percent oxidized glutathione) to distinguish children with ASD from typically developing children. The model is used here to evaluate an independent cohort of individuals having ASD with diagnosed co-occurring conditions (age range 2–17 years old) and assess classifier performance in the presence/absence of these conditions. The four categories of co-occurring conditions considered were allergic disorders, gastrointestinal disorders, immune/metabolic disorders, and neurological disorders. All data were collected and retrospectively analyzed from previous clinical studies.
Results
The model was able to identify 124 of 131 participants with ASD (94.7 %) correctly regardless of co-occurring condition status. Model performance was generally not sensitive to the absence or presence of most co-occurring conditions, with the exceptions of ever/never having allergies or gastrointestinal symptoms, or currently (not) having any condition, all of which had minor impacts on model prediction accuracy.
Conclusion
The results of this exploratory study suggest that a FOCM/TS-based classifier for diagnosing ASD may potentially be robust to variations in co-occurring conditions and potentially applicable across ASD subtypes. Larger, more comprehensive follow-up studies with typically developing and/or developmentally delayed control groups are required to provide a more conclusive assessment of classifier robustness to co-occurring conditions.