Summary: A new machine-learning algorithm which videos of echocardiograms is able to accurately predict patients who will die within a year.
Source: Geisinger Health System
Researchers at Geisinger have found that a computer algorithm developed using echocardiogram videos of the heart can predict mortality within a year.
The algorithm–an example of what is known as machine learning, or artificial intelligence (AI)–outperformed other clinically used predictors, including pooled cohort equations and the Seattle Heart Failure score.
The results of the study were published in Nature Biomedical Engineering.
“We were excited to find that machine learning can leverage unstructured datasets such as medical images and videos to improve on a wide range of clinical prediction models,” said Chris Haggerty, Ph.D., co-senior author and assistant professor in the Department of Translational Data Science and Informatics at Geisinger.
Imaging is critical to treatment decisions in most medical specialties and has become one of the most data-rich components of the electronic health record (EHR). For example, a single ultrasound of the heart yields approximately 3,000 images, and cardiologists have limited time to interpret these images within the context of numerous other diagnostic data. This creates a substantial opportunity to leverage technology, such as machine learning, to manage and analyze this data and ultimately provide intelligent computer assistance to physicians.
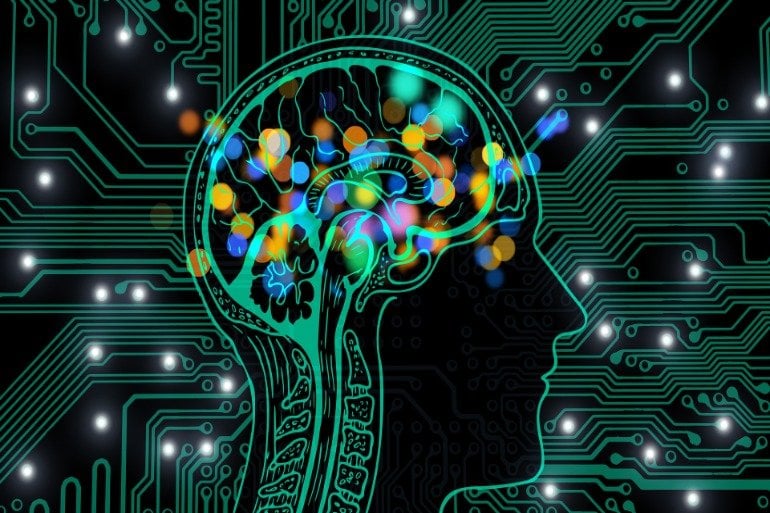
For their study, the research team used specialized computational hardware to train the machine learning model on 812,278 echocardiogram videos collected from 34,362 Geisinger patients over the last ten years. The study compared the results of the model to cardiologists’ predictions based on multiple surveys. A subsequent survey showed that when assisted by the model, cardiologists’ prediction accuracy improved by 13 percent. Leveraging nearly 50 million images, this study represents one of the largest medical image datasets ever published.
“Our goal is to develop computer algorithms to improve patient care,” said Alvaro Ulloa Cerna, Ph.D., author and senior data scientist in the Department of Translational Data Science and Informatics at Geisinger. “In this case, we’re excited that our algorithm was able to help cardiologists improve their predictions about patients, since decisions about treatment and interventions are based on these types of clinical predictions.”
Funding: The research was supported in part by funding from the Pennsylvania Department of Health and the Geisinger Health Plan and Clinic.
About this artificial intelligence research news
Source: Geisinger Health System
Contact: Ashley Andyshak Hayes – Geisinger Health System
Image: The image is in the public domain
Original Research: Closed access.
“Deep-learning-assisted analysis of echocardiographic videos improves predictions of all-cause mortality” by Chris Haggerty et al. Nature Biomedical Engineering
Abstract
Deep-learning-assisted analysis of echocardiographic videos improves predictions of all-cause mortality
Machine learning promises to assist physicians with predictions of mortality and of other future clinical events by learning complex patterns from historical data, such as longitudinal electronic health records. Here we show that a convolutional neural network trained on raw pixel data in 812,278 echocardiographic videos from 34,362 individuals provides superior predictions of one-year all-cause mortality. The model’s predictions outperformed the widely used pooled cohort equations, the Seattle Heart Failure score (measured in an independent dataset of 2,404 patients with heart failure who underwent 3,384 echocardiograms), and a machine learning model involving 58 human-derived variables from echocardiograms and 100 clinical variables derived from electronic health records. We also show that cardiologists assisted by the model substantially improved the sensitivity of their predictions of one-year all-cause mortality by 13% while maintaining prediction specificity. Large unstructured datasets may enable deep learning to improve a wide range of clinical prediction models.