Summary: A novel AI system recognizes a neuron in microscope images of the brain more efficiently than any previous approach. The system improves current methods of tracing neurons and their connections, which can help improve brain mapping.
Source: Cold Spring Harbor Laboratory
Cold Spring Harbor Laboratory (CSHL) scientists have taught computers to recognize a neuron in microscope images of the brain more efficiently than any previous approach. The researchers improved the efficiency of automated methods for tracing neurons and their connections, a task that is increasingly in demand as researchers work to map the brain’s densely interconnected circuits. They did it by teaching the computer to recognize different parts of neurons, each of which have different characteristics.
Such connection maps are critical for learning how the brain processes information to generate thoughts and behavior.
In recent years, new imaging technologies and an expanded capacity to store digital images have led to an enormous volume of data, capturing the paths of neurons as they wend their way through the brains of mice and other model organisms. But there are not enough experts to analyze all those images, says CSHL Professor Partha Mitra, whose team developed the new artificial intelligence (AI) tool and reported on it in the journal Nature Machine Intelligence. Mitra says:
“I think of this project as building a virtual neuroanatomist. And the reason we need it is that the work that we’re doing has traditionally been done by expert humans who require, of course, decades of training. They have a tremendous amount of knowledge. They have looked at–I don’t know–hundreds of thousands of images. They understand the context. And they can offer expert judgment and interpretation.”
Automated methods must take over this work, Mitra says, but computers aren’t as good as humans at interpreting visual information. What an expert anatomist quickly recognizes as a single neuron meandering across a crowded microscope image is not as obvious to an algorithm–at least not without extensive training in which the computer is allowed to learn, over and over again, from large datasets.
“Modern machine learning techniques . . . are still not good enough. And what’s missing is that they often don’t have some of the prior knowledge or information that we as humans would have in making these judgments,” Mitra says. “So we need to build in some kind of prior information.”
The researchers did this using a form of mathematics called topological data analysis, a way to see things as 3D spaces with hills, valleys, and curves. Topology is sometimes called “‘rubber sheet geometry’ that emphasizes connectivity,” says Mitra, in contrast with the kind of geometry that relies on precise lengths and angles.
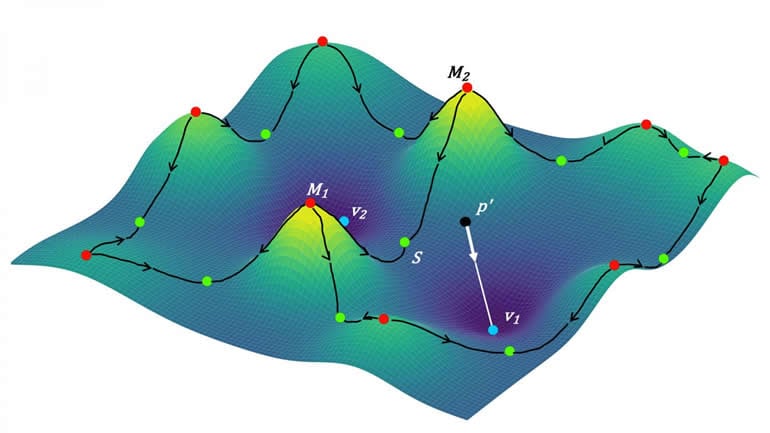
The researchers used simplified mathematical descriptions of the shapes of neuronal parts–plump cell bodies, slender axons, and branched dendrites. Neurons vary tremendously in their overall shapes, but by showing the computer how neurons connect using a few basic forms, the team significantly improved the program’s ability to detect axons and dendrites.
Mitra says, “automated image analysis will still require a human proofreader for the foreseeable future, to ensure quality in scientific applications–but by enhancing the computer’s accuracy, this new method significantly reduces the work that must be done by an expert.”
With the help of a new National Institutes of Health grant, Mitra’s group will develop their AI data analysis tools even more. These tools are critical to the U.S. Brain Initiative, of which his research is a part. He hopes this approach will untangle the mysteries of how brains connect so humans can think about how brains actually work.
About this artificial intelligence research article
Source:
Garvan Institute of Medical Research
Contacts:
Sara Roncero-Menendez – Cold Spring Harbor Laboratory
Image Source:
The image is credited to Mitra lab/CSHL, 2020.
Original Research: Closed access
“Semantic segmentation of microscopic neuroanatomical data by combining topological priors with encoder–decoder deep networks” by Samik Banerjee, Lucas Magee, Dingkang Wang, Xu Li, Bing-Xing Huo, Jaikishan Jayakumar, Katherine Matho, Meng-Kuan Lin, Keerthi Ram, Mohanasankar Sivaprakasam, Josh Huang, Yusu Wang & Partha P. Mitra. Nature Machine Intelligence.
Abstract
Semantic segmentation of microscopic neuroanatomical data by combining topological priors with encoder–decoder deep networks
Understanding of neuronal circuitry at cellular resolution within the brain has relied on neuron tracing methods that involve careful observation and interpretation by experienced neuroscientists. With recent developments in imaging and digitization, this approach is no longer feasible with the large-scale (terabyte to petabyte range) images. Machine-learning-based techniques, using deep networks, provide an efficient alternative to the problem. However, these methods rely on very large volumes of annotated images for training and have error rates that are too high for scientific data analysis, and thus requires a substantial volume of human-in-the-loop proofreading. Here we introduce a hybrid architecture combining prior structure in the form of topological data analysis methods, based on discrete Morse theory, with the best-in-class deep-net architectures for the neuronal connectivity analysis. We show significant performance gains using our hybrid architecture on detection of topological structure (for example, connectivity of neuronal processes and local intensity maxima on axons corresponding to synaptic swellings) with precision and recall close to 90% compared with human observers. We have adapted our architecture to a high-performance pipeline capable of semantic segmentation of light-microscopic whole-brain image data into a hierarchy of neuronal compartments. We expect that the hybrid architecture incorporating discrete Morse techniques into deep nets will generalize to other data domains.