Summary: Researchers developed a brain-inspired AI technique using neural networks to model the challenging quantum states of molecules, crucial for technologies like solar panels and photocatalyst.
This new approach significantly improves accuracy, enabling better prediction of molecular behaviors during energy transitions. By enhancing our understanding of molecular excited states, this research could revolutionize material prototyping and chemical synthesis.
Key Facts:
- Neural networks modeled molecular excited states with unprecedented accuracy.
- Achieved five times greater precision than previous methods for complex molecules.
- Could lead to computer-simulated material and chemical prototyping.
Source: Imperial College London
New research using neural networks, a form of brain-inspired AI, proposes a solution to the tough challenge of modelling the states of molecules.
The research shows how the technique can help solve fundamental equations in complex molecular systems.
This could lead to practical uses in the future, helping researchers to prototype new materials and chemical syntheses using computer simulation before trying to make them in the lab.
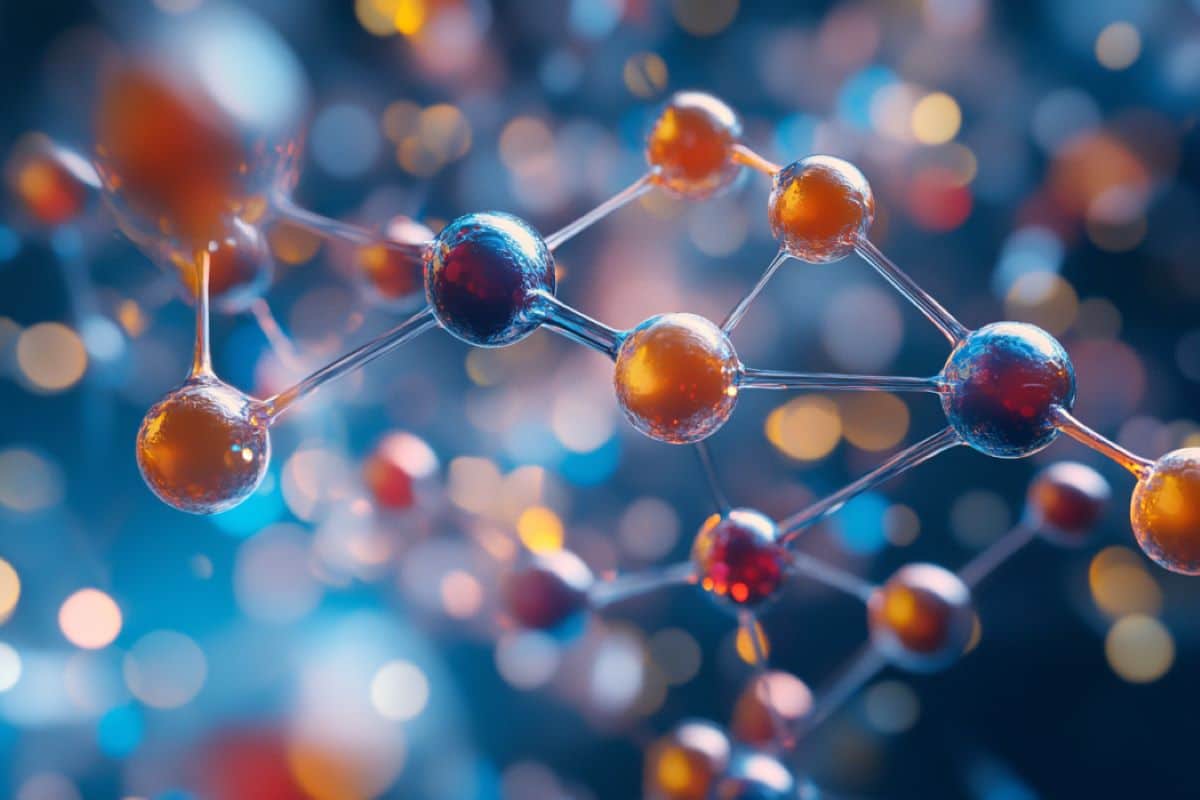
Led by Imperial College London and Google DeepMind scientists, the study is published today in Science.
Excited molecules
The team investigated the problem of understanding how molecules transition to and from ‘excited states’. When molecules and materials are stimulated by a large amount of energy, such as being exposed to light or high temperatures, their electrons can get kicked into a temporary new configuration, known as an excited state.
The exact amount of energy absorbed and released as molecules transition between states creates a unique fingerprint for different molecules and materials. This affects the performance of technologies ranging from solar panels and LEDs to semiconductors and photocatalysts. They also play a critical role in biological processes involving light, including photosynthesis and vision.
However, this fingerprint is extremely difficult to model because the excited electrons are quantum in nature, meaning their positions within the molecules are never certain, and can only be expressed as probabilities.
Lead researcher Dr David Pfau, from Google DeepMind and the Department of Physics at Imperial, said: “Representing the state of a quantum system is extremely challenging. A probability has to be assigned to every possible configuration of electron positions.
“The space of all possible configurations is enormous — if you tried to represent it as a grid with 100 points along each dimension, then the number of possible electron configurations for the silicon atom would be larger than the number of atoms in the universe. This is exactly where we thought deep neural networks could help.”
Neural networks
The researchers developed a new mathematical approach and used it with a neural network called FermiNet (Fermionic Neural Network), which was the first example where deep learning was used to compute the energy of atoms and molecules from fundamental principles that was accurate enough to be useful.
The team tested their approach with a range of examples, with promising results. On a small but complex molecule called the carbon dimer, they achieved a mean absolute error (MAE) of 4 meV (millielectronvolt – a tiny measure of energy), which is five times closer to experimental results than prior gold standard methods reaching 20 meV.
Dr Pfau said: “We tested our method on some of the most challenging systems in computational chemistry, where two electrons are excited simultaneously, and found we were within around 0.1 eV of the most demanding, complex calculations done to date.
“Today, we’re making our latest work open source, and hope the research community will build upon our methods to explore the unexpected ways matter interacts with light.”
About this artificial intelligence (AI) research news
Author: Hayley Dunning
Source: Imperial College London
Contact: Hayley Dunning – Imperial College London
Image: The image is credited to Neuroscience News
Original Research: Closed access.
“Accurate Computation of Quantum Excited States with Neural Networks” by David Pfau et al. Science
Abstract
Accurate Computation of Quantum Excited States with Neural Networks
INTRODUCTION
Understanding the physics of how matter interacts with light requires accurate modeling of electronic excited states of quantum systems. This underpins the behavior of photocatalysts, fluorescent dyes, quantum dots, light-emitting diodes (LEDs), lasers, solar cells, and more.
Existing quantum chemistry methods for excited states can be much more inaccurate than those for ground states, sometimes qualitatively so, or can require prior knowledge targeted to specific states. Neural networks combined with variational Monte Carlo (VMC) have achieved remarkable accuracy for ground state wave functions for a range of systems, including spin models, molecules, and condensed matter systems.
Although VMC has been used to study excited states, prior approaches have limitations that make it difficult or impossible to use them with neural networks and often have many free parameters that require tuning to achieve good results.
RATIONALE
We combine the flexibility of neural network ansätze with a mathematical insight that allows us to convert the problem of finding excited states of a system to one of finding the ground state of an expanded system, which can then be tackled with standard VMC. We call this approach natural excited states VMC (NES-VMC).
Linear independence of the excited states is automatically imposed through the functional form of the ansatz. The energy and other observables of each excited state are obtained from diagonalizing the matrix of Hamiltonian expectation values taken over the single-state ansätze, which can be accumulated with no additional cost.
Crucially, this approach has no free parameters to tune and needs no penalty terms to enforce orthogonalization. We examined the accuracy of this approach with two different neural network architectures—the FermiNet and Psiformer.
RESULTS
We demonstrated our approach on benchmark systems ranging from individual atoms up to molecules the size of benzene. We validated the accuracy of NES-VMC on first-row atoms, closely matching experimental results, and on a range of small molecules, obtaining highly accurate energies and oscillator strengths comparable to existing best theoretical estimates.
We computed the potential energy curves of the lowest excited states of the carbon dimer and identified the states across bond lengths by analyzing their symmetries and spins. The NES-VMC vertical excitation energies matched those obtained using the highly accurate semistochastic heat-bath configuration interaction (SHCI) method to within chemical accuracy for all bond lengths, whereas the adiabatic excitations were within 4 meV of experimental values on average—a fourfold improvement over SHCI.
In the case of ethylene, NES-VMC correctly described the conical intersection of the twisted molecule and was in excellent agreement with highly accurate multireference configuration interaction (MR-CI) results. We also considered five challenging systems with low-lying double excitations, including multiple benzene-scale molecules.
On all systems where there is good agreement between methods on the vertical excitation energies, the Psiformer was within chemical accuracy across states, including butadiene, where even the ordering of certain states has been disputed for many decades. On tetrazine and cyclopentadienone, where state-of-the-art calculations from just a few years ago were known to be inaccurate, NES-VMC results closely matched recent sophisticated diffusion Monte Carlo (DMC) and complete-active-space third-order perturbation theory (CASPT3) calculations.
Finally, we considered the benzene molecule, where NES-VMC combined with the Psiformer ansatz is in substantially better agreement with theoretical best estimates compared with other methods, including neural network ansätze using penalty methods. This both validates the mathematical correctness of our approach and shows that neural networks can accurately represent excited states of molecules right at the current limit of computational approaches.
CONCLUSION
NES-VMC is a parameter-free and mathematically sound variational principle for excited states. Combining it with neural network ansätze enables marked accuracy across a wide range of benchmark problems. The development of an accurate VMC approach to excited states of quantum systems opens many possibilities and substantially expands the scope of applications of neural network wave functions.
Although we considered only electronic excitations of molecular systems and neural network ansätze, NES-VMC is applicable to any quantum Hamiltonian and any ansatz, enabling accurate computational studies that could improve our understanding of vibronic couplings, optical bandgaps, nuclear physics, and other challenging problems.