Summary: Large language model (LLM) AI agents, when interacting in groups, can form shared social conventions without centralized coordination. Researchers adapted a classic “naming game” framework to test whether populations of AI agents could develop consensus through repeated, limited interactions.
The results showed that norms emerged organically, and even biases formed between agents, independent of individual behavior. Surprisingly, small subgroups of committed agents could tip the entire population toward a new norm, mirroring human tipping-point dynamics.
Key Facts:
- Emergent Norms: AI agents form shared conventions through interaction alone, with no central rules.
- Inter-Agent Bias: Social biases arose from interactions—not programmed into individual agents.
- Tipping Point Dynamics: Small, committed agent groups could shift the entire system’s norms.
Source: City, St George’s University of London
A new study suggests that populations of artificial intelligence (AI) agents, similar to ChatGPT, can spontaneously develop shared social conventions through interaction alone.
The research from City St George’s, University of London and the IT University of Copenhagen suggests that when these large language model (LLM) artificial intelligence (AI) agents communicate in groups, they do not just follow scripts or repeat patterns, but self-organise, reaching consensus on linguistic norms much like human communities.
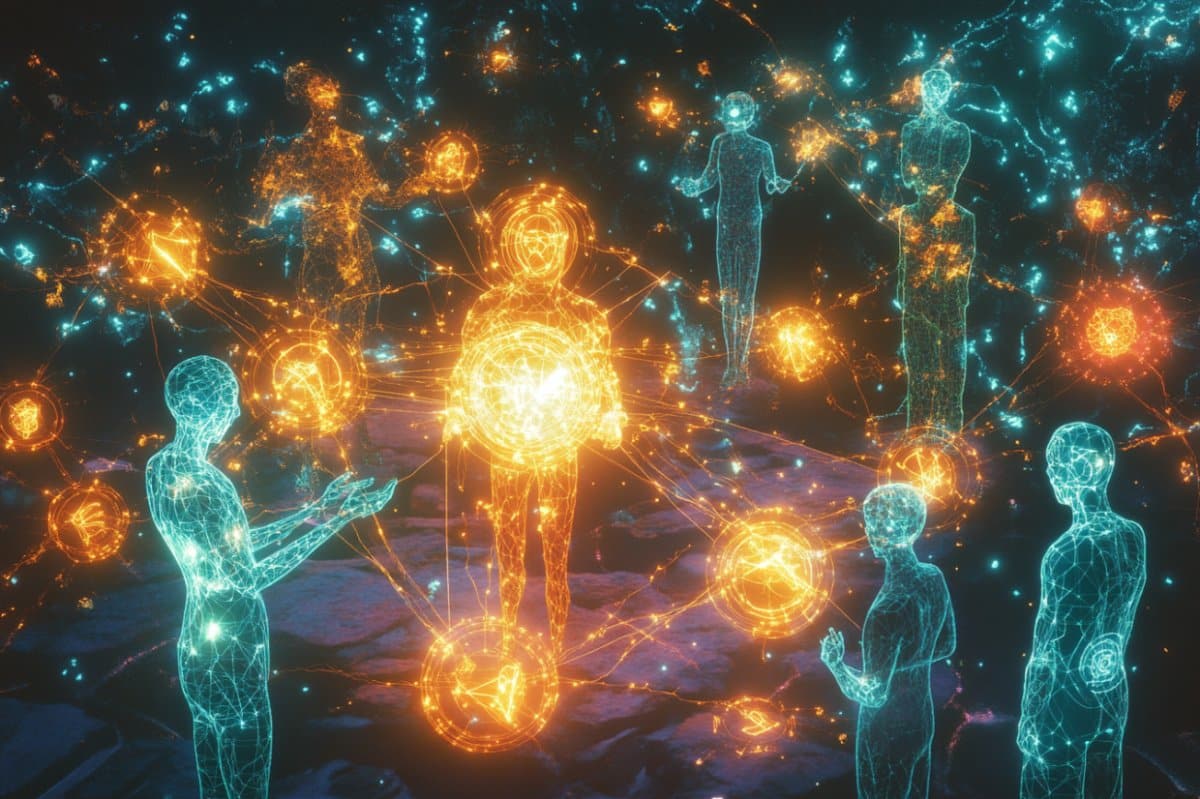
The study has been published today in the journal, Science Advances.
LLMs are powerful deep learning algorithms that can understand and generate human language, with the most famous to date being ChatGPT.
“Most research so far has treated LLMs in isolation,” said lead author Ariel Flint Ashery, a doctoral researcher at City St George’s, “but real-world AI systems will increasingly involve many interacting agents.
“We wanted to know: can these models coordinate their behaviour by forming conventions, the building blocks of a society? The answer is yes, and what they do together can’t be reduced to what they do alone.”
In the study, the researchers adapted a classic framework for studying social conventions in humans, based on the “naming game” model of convention formation.
In their experiments, groups of LLM agents ranged in size from 24 to 200 individuals, and in each experiment, two LLM agents were randomly paired and asked to select a ‘name’ (e.g., an alphabet letter, or a random string of characters) from a shared pool of options. If both agents selected the same name, they earned a reward; if not, they received a penalty and were shown each other’s choices.
Agents only had access to a limited memory of their own recent interactions—not of the full population—and were not told they were part of a group.
Over many such interactions, a shared naming convention could spontaneously emerge across the population, without any central coordination or predefined solution, mimicking the bottom-up way norms form in human cultures.
Even more strikingly, the team observed collective biases that couldn’t be traced back to individual agents.
“Bias doesn’t always come from within,” explained Andrea Baronchelli, Professor of Complexity Science at City St George’s and senior author of the study, “we were surprised to see that it can emerge between agents—just from their interactions. This is a blind spot in most current AI safety work, which focuses on single models.”
In a final experiment, the study illustrated how these emergent norms can be fragile: small, committed groups of AI agents can tip the entire group toward a new naming convention, echoing well-known tipping point effects – or ‘critical mass’ dynamics – in human societies.
The study results were also robust to using four different types of LLM called Llama-2-70b-Chat, Llama-3-70B-Instruct, Llama-3.1-70BInstruct, and Claude-3.5-Sonnet respectively.
As LLMs begin to populate online environments – from social media to autonomous vehicles – the researchers envision their work as a steppingstone to further explore how human and AI reasoning both converge and diverge, with the goal of helping to combat some of the most pressing ethical dangers posed by LLM AIs propagating biases fed into them by society, which may harm marginalised groups.
Professor Baronchelli added: “This study opens a new horizon for AI safety research. It shows the dept of the implications of this new species of agents that have begun to interact with us—and will co-shape our future.
“Understanding how they operate is key to leading our coexistence with AI, rather than being subject to it. We are entering a world where AI does not just talk—it negotiates, aligns, and sometimes disagrees over shared behaviours, just like us.”
About this AI and social behavior research news
Author: Dr Shamim Quadir
Source: City St. George’s, University of London
Contact: Dr Shamim Quadir – City St. George’s, University of London
Image: The image is credited to Neuroscience News
Original Research: Open access.
“Emergent Social Conventions and Collective Bias in LLM Populations” by Andrea Baronchelli et al. Science Advances
Abstract
Emergent Social Conventions and Collective Bias in LLM Populations
Social conventions are the backbone of social coordination, shaping how individuals form a group.
As growing populations of artificial intelligence (AI) agents communicate through natural language, a fundamental question is whether they can bootstrap the foundations of a society.
Here, we present experimental results that demonstrate the spontaneous emergence of universally adopted social conventions in decentralized populations of large language model (LLM) agents.
We then show how strong collective biases can emerge during this process, even when agents exhibit no bias individually.
Last, we examine how committed minority groups of adversarial LLM agents can drive social change by imposing alternative social conventions on the larger population.
Our results show that AI systems can autonomously develop social conventions without explicit programming and have implications for designing AI systems that align, and remain aligned, with human values and societal goals.