Summary: A new study finds that large language models (LLMs), like GPT-J, generate words not by applying fixed grammatical rules, but by drawing analogies, mirroring how humans process unfamiliar language. When faced with made-up adjectives, the LLM chose noun forms based on similarity to words it had seen in training, just as humans do.
However, unlike humans, LLMs don’t build mental dictionaries; they treat each instance of a word as unique, relying heavily on memorized examples. This analogy-based pattern-matching explains both their impressive fluency and their need for massive data inputs.
Key Facts:
- Analogy Over Rules: LLMs generalize new word forms through analogy, not grammar.
- No Mental Dictionary: Unlike humans, LLMs do not consolidate word instances into abstract categories.
- Data-Hungry: Their lack of abstraction may explain why LLMs need much more data than humans to learn language.
Source: Oxford University
A new study led by researchers at the University of Oxford and the Allen Institute for AI (Ai2) has found that large language models (LLMs) – the AI systems behind chatbots like ChatGPT – generalize language patterns in a surprisingly human-like way: through analogy, rather than strict grammatical rules.
The findings were published on 9 May in the journal PNAS.
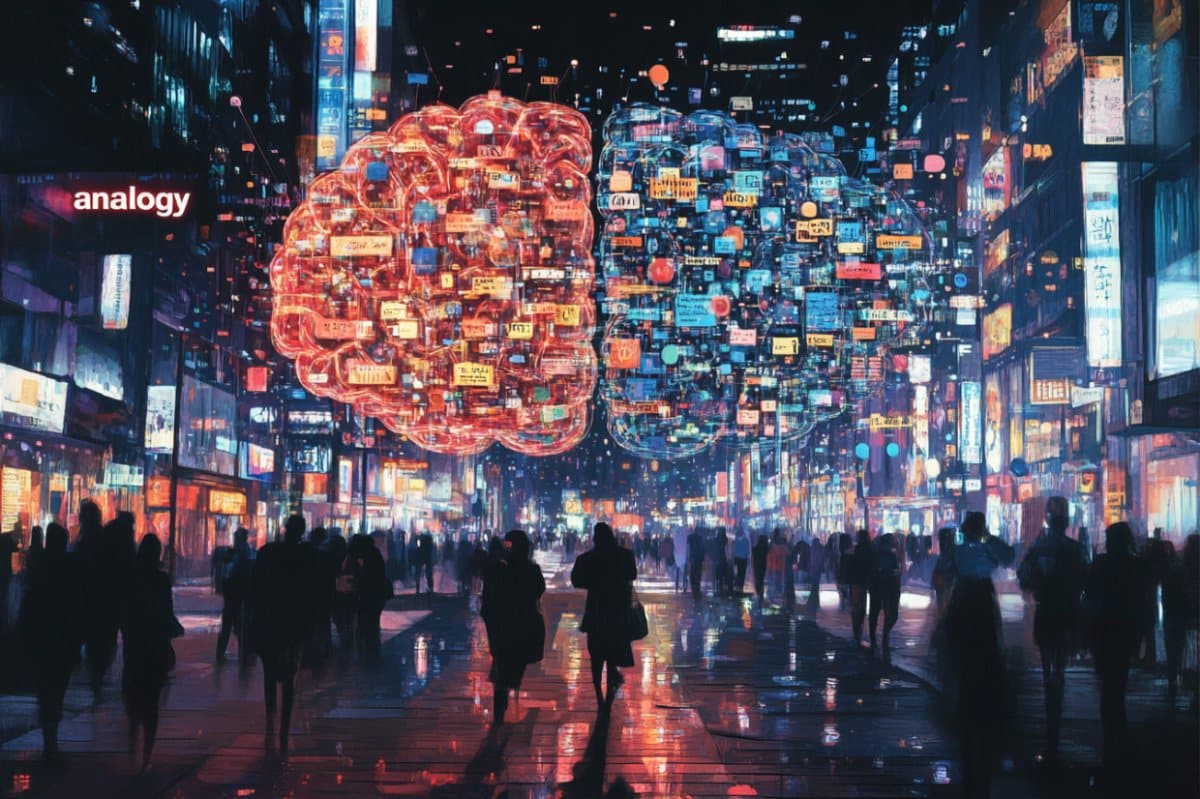
The research challenges a widespread assumption about LLMs: that these learn how to generate language primarily by inferring rules from their training data. Instead, the models rely heavily on stored examples and draw analogies when dealing with unfamiliar words, much as people do.
To explore how LLMs generate language, the study compared judgments made by humans with those made by GPT-J (an open-source large language model developed by EleutherAI in 2021) on a very common word formation pattern in English, which turns adjectives into nouns by adding the suffix “-ness” or “-ity”.
For instance, happy becomes happiness, and available becomes availability.
The research team generated 200 made-up English adjectives that the LLM had never encountered before – words such as cormasive and friquish. GPT-J was asked to turn each one into a noun by choosing between -ness and -ity (for example, deciding between cormasivity and cormasiveness).
The LLM’s responses were compared to the choices made by people, and to predictions made by two well-established cognitive models.
One model generalises using rules, and another uses analogical reasoning based on similarity to stored examples.
The results revealed that the LLM’s behaviour resembled human analogical reasoning. Rather than using rules, it based its answers on similarities to real words it had “seen” during training – much as people do when thinking about new words.
For instance, friquish is turned into friquishness on the basis of its similarity to words like selfish, whereas the outcome for cormasive is influenced by word pairs such as sensitive, sensitivity.
The study also found pervasive and subtle influences of how often word forms had appeared in the training data. The LLM’s responses on nearly 50,000 real English adjectives were probed, and its predictions matched the statistical patterns in its training data with striking precision.
The LLM behaved as if it had formed a memory trace from every individual example of every word it has encountered during training.
Drawing on these stored ‘memories’ to make linguistic decisions, it appeared to handle anything new by asking itself: “What does this remind me of?”
The study also revealed a key difference between how human beings and LLMs form analogies over examples.
Humans acquire a mental dictionary – a mental store of all the word forms that they consider to be meaningful words in their language, regardless of how often they occur. They easily recognize that forms like friquish and cormasive are not words of English at this time.
To deal with these potential neologisms, they make analogical generalizations based on the variety of known words in their mental dictionaries.
The LLMs, in contrast, generalize directly over all the specific instances of words in the training set, without unifying instances of the same word into a single dictionary entry.
Senior author Janet Pierrehumbert, Professor of Language Modelling at Oxford University, said: “Although LLMs can generate language in a very impressive manner, it turns out that they do not think as abstractly as humans do.
“This probably contributes to the fact that their training requires so much more language data than humans need to learn a language.”
Co-lead author Dr. Valentin Hofman (Ai2 and University of Washington) said: “This study is a great example of synergy between Linguistics and AI as research areas.
“The findings give us a clearer picture of what’s going on inside LLMs when they generate language, and will support future advances in robust, efficient, and explainable AI.”
The study also involved researchers from LMU Munich and Carnegie Mellon University.
About this AI and learning research news
Author: Philippa Sims
Source: Oxford University
Contact: Philippa Sims – Oxford University
Image: The image is credited to Neuroscience News
Original Research: Open access.
“Derivational Morphology Reveals Analogical Generalization in Large Language Models” by Janet Pierrehumbert et al. PNAS
Abstract
Derivational Morphology Reveals Analogical Generalization in Large Language Models
What mechanisms underlie linguistic generalization in large language models (LLMs)?
This question has attracted considerable attention, with most studies analyzing the extent to which the language skills of LLMs resemble rules.
As of yet, it is not known whether linguistic generalization in LLMs could equally well be explained as the result of analogy.
A key shortcoming of prior research is its focus on regular linguistic phenomena, for which rule-based and analogical approaches make the same predictions.
Here, we instead examine derivational morphology, specifically English adjective nominalization, which displays notable variability.
We introduce a method for investigating linguistic generalization in LLMs: Focusing on GPT-J, we fit cognitive models that instantiate rule-based and analogical learning to the LLM training data and compare their predictions on a set of nonce adjectives with those of the LLM, allowing us to draw direct conclusions regarding underlying mechanisms.
As expected, rule-based and analogical models explain the predictions of GPT-J equally well for adjectives with regular nominalization patterns.
However, for adjectives with variable nominalization patterns, the analogical model provides a much better match.
Furthermore, GPT-J’s behavior is sensitive to the individual word frequencies, even for regular forms, a behavior that is consistent with an analogical account but not a rule-based one.
These findings refute the hypothesis that GPT-J’s linguistic generalization on adjective nominalization involves rules, suggesting analogy as the underlying mechanism.
Overall, our study suggests that analogical processes play a bigger role in the linguistic generalization of LLMs than previously thought.