Summary: Researchers developed methods to predict emotions in spontaneous thoughts using fMRI and machine learning. They crafted personalized narratives that participants read while their brain activity was monitored, aiming to decode the emotional dimensions of thoughts.
By analyzing fMRI data, the team identified key brain regions involved in processing personal relevance and emotional valence. Their breakthrough offers insights into the emotional undertones of daydreams and could revolutionize mental health diagnostics.
Key Facts:
- The study utilized fMRI and machine learning to predict subjective feelings during story reading and spontaneous thinking.
- Key brain areas like the anterior insula and midcingulate cortex were crucial in predicting personal relevance and emotional tone.
- The research suggests potential applications in understanding individual emotional experiences and improving mental health assessments.
Source: Institute for Basic Science
A team of researchers led by KIM Hong Ji and WOO Choong-Wan at the Center for Neuroscience Imaging Research (CNIR) within the Institute for Basic Science (IBS), in collaboration with Emily FINN at Dartmouth College, has unlocked a new realm of understanding within the human brain.
The team demonstrated the possibility of using functional Magnetic Resonance Imaging (fMRI) and machine learning algorithms to predict subjective feelings in people’s thoughts while reading stories or in a freely thinking state.
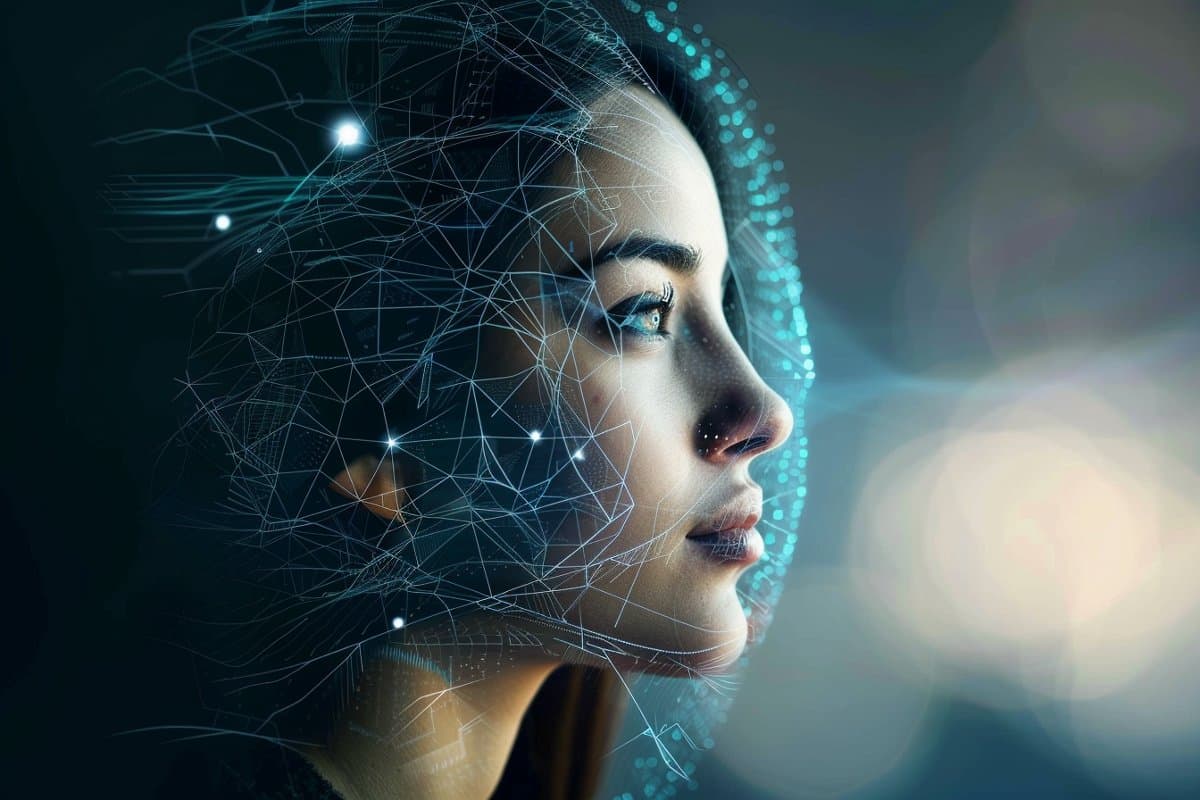
The brain is constantly active, and spontaneous thoughts occur even during rest or sleep. These thoughts can be anything ranging from memories of the past to aspirations for the future, and they are often intertwined with emotions and personal concerns.
However, because spontaneous thought typically occurs without any constraint of consciousness, researching them poses challenges – even simply asking individuals what they are currently thinking can change the nature of their thoughts.
New research suggests that it may be possible to develop predictive models of affective contents during spontaneous thought by combining personal narratives with fMRI. Narratives and spontaneous thoughts share similar characteristics, including rich semantic information and temporally unfolding nature.
To capture a diverse range of thought patterns, participants engaged in one-on-one interviews to craft personalized narrative stimuli, reflecting their past experiences and emotions. While participants read their stories inside the MRI scanner, their brain activity was recorded.
After the fMRI scan, the participants were asked to read the stories again and report perceived self-relevance (i.e., how much this content is related to themselves) and valence (i.e., how much this content is positive or negative) at each moment.
Using a quintile (five levels) from each participant’s self-relevance and valence ratings, 25 (5 levels of self-relevance rating × 5 levels of valence rating) possible segments of fMRI and rating data were created.
The team then harnessed machine learning techniques to train predictive models, combining these data with the fMRI brain scans from 49 individuals to decode the “emotional dimensions” of thoughts in real time.
To interpret the brain representations of the predictive models, the research team employed multiple approaches, such as virtual lesion and virtual isolation analyses at both region and network levels.
Through these analyses, they discovered the significance of the default mode, ventral attention, and frontoparietal networks in both self-relevance and valence predictions.
Specifically, they identified the involvement of the anterior insula and midcingulate cortex in self-relevance prediction, while the left temporoparietal junction and dorsomedial prefrontal cortex played important roles in valence prediction.
Moreover, the predictive models showed their capacity to predict both self-relevance and valence not only during story reading but also when applied to data from 199 individuals engaging in spontaneous, task-free thinking or even during resting. These findings show the promise of daydream decoding.
“Several tech companies and research teams are currently endeavoring to decode words or images directly from brain activity, but there are limited initiatives aimed at decoding intimate emotions underlying these thoughts,” stated Dr. WOO Choong-Wan, associate director of IBS, who led the study.
“Our research is centered on human emotions, with the aim of decoding emotions within the natural flow of thoughts to obtain information that can benefit people’s mental health.”
KIM Hongji, a doctoral candidate and the first author of this study, emphasized, “This study holds significance as we decoded the emotional state associated with general thoughts, rather than targeting emotions limited to specific tasks,” adding,
“These findings advance our understanding of the internal states and contexts influencing subjective experiences, potentially shedding light on individual differences in thoughts and emotions, and aiding in the evaluation of mental well-being.”
About this AI and emotion research news
Author: William Suh
Source: Institute for Basic Science
Contact: William Suh – Institute for Basic Science
Image: The image is credited to Neuroscience News
Original Research: Closed access.
“Brain decoding of spontaneous thought: predictive modeling of self-relevance and valence using personal narratives” by KIM Hong Ji et al. PNAS
Abstract
Brain decoding of spontaneous thought: predictive modeling of self-relevance and valence using personal narratives
The contents and dynamics of spontaneous thought are important factors for personality traits and mental health. However, assessing spontaneous thoughts is challenging due to their unconstrained nature, and directing participants’ attention to report their thoughts may fundamentally alter them.
Here, we aimed to decode two key content dimensions of spontaneous thought—self-relevance and valence—directly from brain activity.
To train functional MRI-based predictive models, we used individually generated personal stories as stimuli in a story-reading task to mimic narrative-like spontaneous thoughts (n = 49). We then tested these models on multiple test datasets (total n = 199).
The default mode, ventral attention, and frontoparietal networks played key roles in the predictions, with the anterior insula and midcingulate cortex contributing to self-relevance prediction and the left temporoparietal junction and dorsomedial prefrontal cortex contributing to valence prediction.
Overall, this study presents brain models of internal thoughts and emotions, highlighting the potential for the brain decoding of spontaneous thought.