Summary: Researchers have found a surprising similarity between the way large language models (LLMs) like ChatGPT process information and how the brains of people with Wernicke’s aphasia function. In both cases, fluent but often incoherent output is produced, suggesting rigid internal processing patterns that can distort meaning.
By applying energy landscape analysis to brain scans and AI model data, scientists observed shared dynamics in signal flow, hinting at deeper structural parallels. These findings could help improve both aphasia diagnosis and AI design, revealing how internal limitations affect language clarity.
Key Facts:
- Cognitive Parallel: AI systems and aphasia patients both generate fluent but unreliable output.
- Shared Dynamics: Brain scans and LLM data reveal similar signal patterns using energy landscape analysis.
- Dual Impact: Insights could refine both AI architecture and clinical diagnostics for language disorders.
Source: University of Tokyo
Agents, chatbots and other tools based on artificial intelligence (AI) are increasingly used in everyday life by many.
So-called large language model (LLM)-based agents, such as ChatGPT and Llama, have become impressively fluent in the responses they form, but quite often provide convincing yet incorrect information.
Researchers at the University of Tokyo draw parallels between this issue and a human language disorder known as aphasia, where sufferers may speak fluently but make meaningless or hard-to-understand statements.
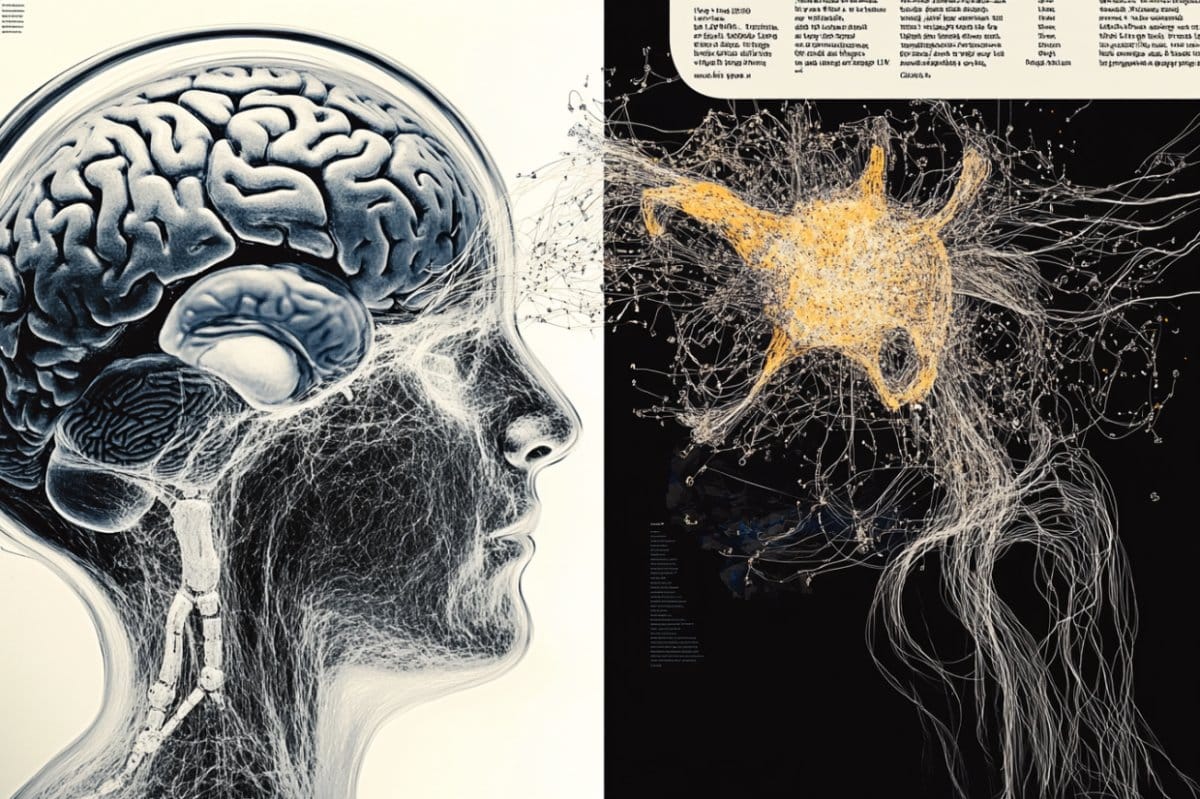
This similarity could point toward better forms of diagnosis for aphasia, and even provide insight to AI engineers seeking to improve LLM-based agents.
This article was written by a human being, but the use of text-generating AI is on the rise in many areas. As more and more people come to use and rely on such things, there’s an ever-increasing need to make sure that these tools deliver correct and coherent responses and information to their users.
Many familiar tools, including ChatGPT and others, appear very fluent in whatever they deliver. But their responses cannot always be relied upon due to the amount of essentially made-up content they produce.
If the user is not sufficiently knowledgeable about the subject area in question, they can easily fall foul of assuming this information is right, especially given the high degree of confidence ChatGPT and others show.
“You can’t fail to notice how some AI systems can appear articulate while still producing often significant errors,” said Professor Takamitsu Watanabe from the International Research Center for Neurointelligence (WPI-IRCN) at the University of Tokyo.
“But what struck my team and I was a similarity between this behavior and that of people with Wernicke’s aphasia, where such people speak fluently but don’t always make much sense.
“That prompted us to wonder if the internal mechanisms of these AI systems could be similar to those of the human brain affected by aphasia, and if so, what the implications might be.”
To explore this idea, the team used a method called energy landscape analysis, a technique originally developed by physicists seeking to visualize energy states in magnetic metal, but which was recently adapted for neuroscience.
They examined patterns in resting brain activity from people with different types of aphasia and compared them to internal data from several publicly available LLMs. And in their analysis, the team did discover some striking similarities.
The way digital information or signals are moved around and manipulated within these AI models closely matched the way some brain signals behaved in the brains of people with certain types of aphasia, including Wernicke’s aphasia.
“You can imagine the energy landscape as a surface with a ball on it. When there’s a curve, the ball may roll down and come to rest, but when the curves are shallow, the ball may roll around chaotically,” said Watanabe.
“In aphasia, the ball represents the person’s brain state. In LLMs, it represents the continuing signal pattern in the model based on its instructions and internal dataset.”
The research has several implications. For neuroscience, it offers a possible new way to classify and monitor conditions like aphasia based on internal brain activity rather than just external symptoms.
For AI, it could lead to better diagnostic tools that help engineers improve the architecture of AI systems from the inside out. Though, despite the similarities the researchers discovered, they urge caution not to make too many assumptions.
“We’re not saying chatbots have brain damage,” said Watanabe.
“But they may be locked into a kind of rigid internal pattern that limits how flexibly they can draw on stored knowledge, just like in receptive aphasia.
“Whether future models can overcome this limitation remains to be seen, but understanding these internal parallels may be the first step toward smarter, more trustworthy AI too.”
Funding: This work was supported by Grant-in-aid for Research Activity from Japan Society for Promotion of Sciences (19H03535, 21H05679, 23H04217, JP20H05921), The University of Tokyo Excellent Young Researcher Project, Showa University Medical Institute of Developmental Disabilities Research, JST Moonshot R&D Program (JPMJMS2021), JST FOREST Program (24012854), Institute of AI and Beyond of UTokyo, Cross-ministerial Strategic Innovation Promotion Program (SIP) on “Integrated Health Care System” (JPJ012425).
About this AI and aphasia research news
Author: Rohan Mehra
Source: University of Tokyo
Contact: Rohan Mehra – University of Tokyo
Image: The image is credited to Neuroscience News
Original Research: Open access.
“Comparison of large language model with aphasia” by Takamitsu Watanabe et al. Advanced Science
Abstract
Comparison of large language model with aphasia
Large language models (LLMs) respond fluently but often inaccurately, which resembles aphasia in humans. Does this behavioral similarity indicate any resemblance in internal information processing between LLMs and aphasic humans?
Here, we address this question by comparing the network dynamics between LLMs—ALBERT, GPT-2, Llama-3.1 and one Japanese variant of Llama—and various aphasic brains.
Using energy landscape analysis, we quantify how frequently the network activity pattern is likely to move from one state to another (transition frequency) and how long it tends to dwell in each state (dwelling time).
First, by investigating the frequency spectrums of these two indices for brain dynamics, we find that the degrees of the polarization of the transition frequency and dwelling time enable accurate classification of receptive aphasia, expressive aphasia and controls: receptive aphasia shows the bimodal distributions for both indices, whereas expressive aphasia exhibits the most uniform distributions.
In parallel, we identify highly polarized distributions in both transition frequency and dwelling time in the network dynamics in the four LLMs.
These findings indicate the similarity in internal information processing between LLMs and receptive aphasia, and the current approach can provide a novel diagnosis and classification tool for LLMs and help their performance improve.