Summary: Artificial intelligence can detect signs of mild cognitive decline and Alzheimer’s disease, even when no symptoms are apparent, by analyzing a person’s speech. The technology could be used as a simple screening method to identify early signs of cognitive impairment.
Source: UT Southwestern
New technologies that can capture subtle changes in a patient’s voice may help physicians diagnose cognitive impairment and Alzheimer’s disease before symptoms begin to show, according to a UT Southwestern Medical Center researcher who led a study published in the Alzheimer’s Association publication Diagnosis, Assessment & Disease Monitoring.
“Our focus was on identifying subtle language and audio changes that are present in the very early stages of Alzheimer’s disease but not easily recognizable by family members or an individual’s primary care physician,” said Ihab Hajjar, M.D., Professor of Neurology at UT Southwestern’s Peter O’Donnell Jr. Brain Institute.
Researchers used advanced machine learning and natural language processing (NLP) tools to assess speech patterns in 206 people – 114 who met the criteria for mild cognitive decline and 92 who were unimpaired. The team then mapped those findings to commonly used biomarkers to determine their efficacy in measuring impairment.
Study participants, who were enrolled in a research program at Emory University in Atlanta, were given several standard cognitive assessments before being asked to record a spontaneous 1- to 2-minute description of artwork.
“The recorded descriptions of the picture provided us with an approximation of conversational abilities that we could study via artificial intelligence to determine speech motor control, idea density, grammatical complexity, and other speech features,” Dr. Hajjar said.
The research team compared the participants’ speech analytics to their cerebral spinal fluid samples and MRI scans to determine how accurately the digital voice biomarkers detected both mild cognitive impairment and Alzheimer’s disease status and progression.
“Prior to the development of machine learning and NLP, the detailed study of speech patterns in patients was extremely labor intensive and often not successful because the changes in the early stages are frequently undetectable to the human ear,” Dr. Hajjar said.
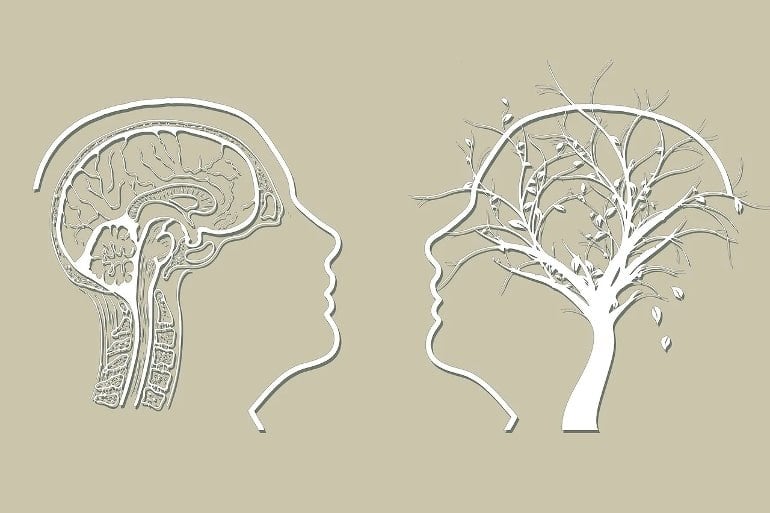
“This novel method of testing performed well in detecting those with mild cognitive impairment and more specifically in identifying patients with evidence of Alzheimer’s disease – even when it cannot be easily detected using standard cognitive assessments.”
During the study, researchers spent fewer than 10 minutes capturing a patient’s voice recording. Traditional neuropsychological tests typically take several hours to administer.
“If confirmed with larger studies, the use of artificial intelligence and machine learning to study vocal recordings could provide primary care providers with an easy-to-perform screening tool for at-risk individuals,” Dr. Hajjar said. “Earlier diagnoses would give patients and families more time to plan for the future and give clinicians greater flexibility in recommending promising lifestyle interventions.”
Dr. Hajjar collaborated on this study with a team of researchers at Emory, where he previously served as Director of the Clinical Trial Unit of the Goizueta Alzheimer’s Disease Research Center before joining UTSW in 2022. He is continuing to collect voice recordings in Dallas as part of a follow-up study at UTSW being funded with a National Institutes of Health grant.
Funding: This study’s research was supported by grants from the National Institutes of Health/National Institute on Aging (AG051633, AG057470-01, AG042127) and the Alzheimer’s Drug Discovery Foundation (20150603).
Dr. Hajjar holds the Pogue Family Distinguished University Chair in Alzheimer’s Disease Clinical Research and Care, in Memory of Maurine and David Weigers McMullan.
About this AI and Alzheimer’s disease research news
Author: Press Office
Source: UT Southwestern
Contact: Press Office – UT Southwestern
Image: The image is in the public domain
Original Research: Closed access.
“Development of digital voice biomarkers and associations with cognition, cerebrospinal biomarkers, and neural representation in early Alzheimer’s disease” by Ihab Hajjar et al. Alzheimer’s & Dementia: Diagnosis, Assessment, and Disease Monitoring
Abstract
Development of digital voice biomarkers and associations with cognition, cerebrospinal biomarkers, and neural representation in early Alzheimer’s disease
Introduction
Advances in natural language processing (NLP), speech recognition, and machine learning (ML) allow the exploration of linguistic and acoustic changes previously difficult to measure. We developed processes for deriving lexical-semantic and acoustic measures as Alzheimer’s disease (AD) digital voice biomarkers.
Methods
We collected connected speech, neuropsychological, neuroimaging, and cerebrospinal fluid (CSF) AD biomarker data from 92 cognitively unimpaired (40 Aβ+) and 114 impaired (63 Aβ+) participants. Acoustic and lexical-semantic features were derived from audio recordings using ML approaches.
Results
Lexical-semantic (area under the curve [AUC] = 0.80) and acoustic (AUC = 0.77) scores demonstrated higher diagnostic performance for detecting MCI compared to Boston Naming Test (AUC = 0.66). Only lexical-semantic scores detected amyloid-β status (p = 0.0003). Acoustic scores associated with hippocampal volume (p = 0.017) while lexical-semantic scores associated with CSF amyloid-β (p = 0.007). Both measures were significantly associated with 2-year disease progression.
Discussion
These preliminary findings suggest that derived digital biomarkers may identify cognitive impairment in preclinical and prodromal AD, and may predict disease progression.