Summary: Researchers improved the performance of natural language processing technology to increase recognitionion of names associated with characters in Game of Thrones, and other literature, from 7% to 90%.
Source: PeerJ
Researchers from the Vrije Universiteit Amsterdam and the Dutch Royal Academy’s Humanities Cluster evaluated four state-of-the-art tools for recognising names in text, to assess and improve their performance on popular fiction. They found solutions to boost the tools’ capability to recognise names in one novel from the accuracy of 7% to 90%.
Natural language processing (NLP) tools are commonly used in many day-to-day applications such as Siri and Google, but the effectiveness of these technologies is not thoroughly understood. Researchers from the Vrije Universiteit Amsterdam and the Dutch Royal Academy’s Humanities Cluster have performed a thorough evaluation of four different name recognition tools on popular 40 novels, including A Game of Thrones. Their analyses, published in PeerJ Computer Science, highlight types of names and texts that are particularly challenging for these tools to identify as well as solutions for mitigating this. In addition, they extracted social networks from the novels to explore differences in story structure. These insights can help make such technologies more robust against genre differences and can help for example make this technology more useful to journalists wanting to analyse large datasets such as the Panama Papers.
Many NLP tools are based on machine learning; that is, a computer program is trained to identify patterns in text based on previously fed examples. To recognise names in text, it is, for example, fed many newspaper articles in which humans have meticulously marked the names. The program is then tasked to ‘learn’ what a name looks like based on context (such as, it is preceded by Mr) or the shape of the word (such as that names generally start with a capital letter in English). Now, the problem when applying such a system trained on newspapers to novels is that authors of novels have much more freedom in their narrative than journalists who need to stick to facts. Fiction authors can make up their own names, such as Tywin or R’hllor, or use descriptive character names straight from the dictionary such as Grey Worm. These names do not behave like ‘normal’ names, thus NLP systems have difficulty recognising them in a text.
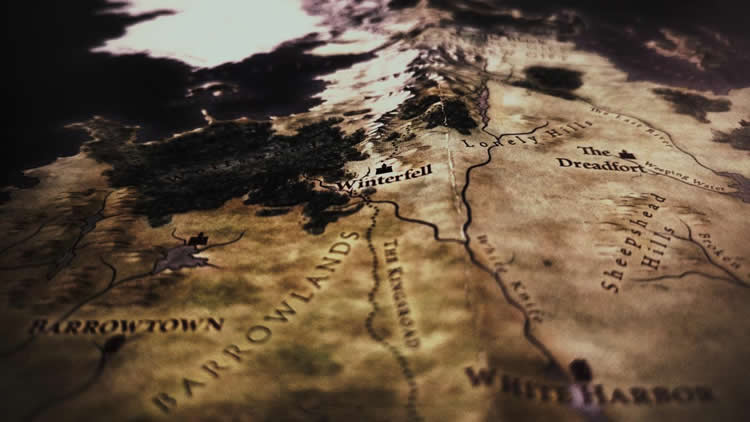
The experiments performed by Niels Dekker (Trifork B.V.), Tobias Kuhn (Vrije Universiteit Amsterdam) and Marieke van Erp (KNAW Humanities Cluster) also highlight the flexibility of language and how names are contextualised in stories. It is for example possible to refer to Daenerys Targaryen as Daenerys and she, but she is also known as Dany, Daenerys Stormborn, Mother of Dragons, Khaleesi, the Unburnt and Mhysa. The social network created for A Game of Thrones illustrates for example that Dany is used by her friends, and her full name Daenerys only by her enemies (in her absence).
The research described in this publication shows that more attention should be paid to the performance of NLP tools and that there is still work to do before ‘text’ can be fully understood by computers.
Source:
PeerJ
Media Contacts:
Thijs van der Veen – PeerJ
Image Source:
The image is credited to mauRÍCIO santos and is in the public domain.
Original Research: Open access.
“Evaluating named entity recognition tools for extracting social networks from novels”
Niels Dekker, Tobias Kuhn, Marieke van Erp. PeerJ Computer Science doi:10.7717/peerj-cs.189
Abstract
Evaluating named entity recognition tools for extracting social networks from novels
The analysis of literary works has experienced a surge in computer-assisted processing. To obtain insights into the community structures and social interactions portrayed in novels, the creation of social networks from novels has gained popularity. Many methods rely on identifying named entities and relations for the construction of these networks, but many of these tools are not specifically created for the literary domain. Furthermore, many of the studies on information extraction from literature typically focus on 19th and early 20th century source material. Because of this, it is unclear if these techniques are as suitable to modern-day literature as they are to those older novels. We present a study in which we evaluate natural language processing tools for the automatic extraction of social networks from novels as well as their network structure. We find that there are no significant differences between old and modern novels but that both are subject to a large amount of variance. Furthermore, we identify several issues that complicate named entity recognition in our set of novels and we present methods to remedy these. We see this work as a step in creating more culturally-aware AI systems.