Summary: A new deep learning algorithm can distinguish different sleep stages. The technology could help with diagnosing sleep disorders, such as obstructive sleep apnea.
Source: University of Eastern Finland
A new deep learning model developed by researchers at the University of Eastern Finland can identify sleep stages as accurately as an experienced physician. This opens up new avenues for the diagnostics and treatment of sleep disorders, including obstructive sleep apnea.
Obstructive sleep apnea (OSA) is a nocturnal breathing disorder that causes a major burden on public health care systems and national economies. It is estimated that up to one billion people worldwide suffer from obstructive sleep apnea, and the number is expected to grow due to population ageing and increased prevalence of obesity. When untreated, OSA increases the risk of cardiovascular diseases and diabetes, among other severe health consequences.
The identification of sleep stages is essential in the diagnostics of sleep disorders, including obstructive sleep apnea. Traditionally, sleep is manually classified into five stages, which are wake, rapid eye movement (REM) sleep and three stages of non-REM sleep. However, manual scoring of sleep stages is time-consuming, subjective and costly.
To overcome these challenges, researchers at the University of Eastern Finland used polysomnographic recording data from healthy individuals and individuals with suspected OSA to develop an accurate deep learning model for automatic classification of sleep stages. In addition, they wanted to find out how the severity of OSA affects classification accuracy.
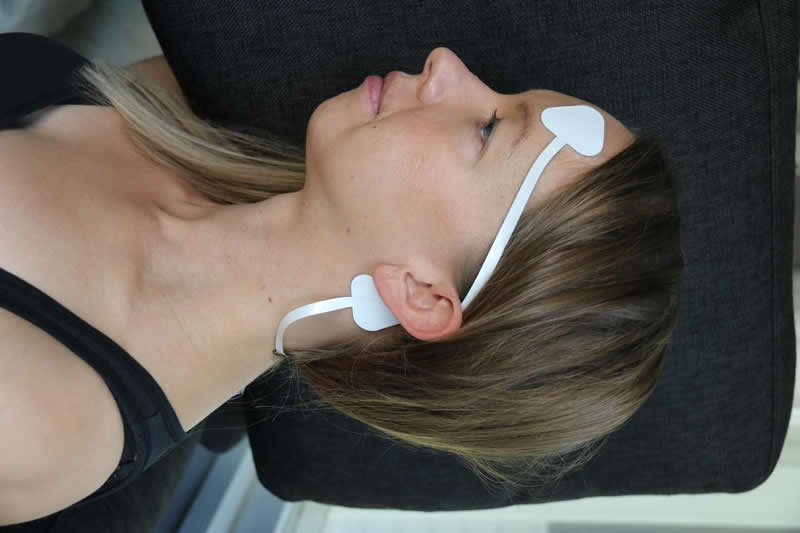
Modern sleep diagnostics is based on wearable, non-intrusive methods. Image is credited to Juha Rutanen.
In healthy individuals, the model was able to identify sleep stages with an 83.7% accuracy when using a single frontal electroencephalography channel (EEG), and with an 83.9% accuracy when supplemented with electrooculogram (EOG). In patients with suspected OSA, the model achieved accuracies of 82.9% (single EEG channel) and 83.8% (EEG and EOG channels). The single-channel accuracies ranged from 84.5% for individuals without OSA to 76.5% for severe OSA patients. The accuracies achieved by the model are equivalent to the correspondence between experienced physicians performing manual sleep scoring. However, the model has the benefit of being systematic and always following the same protocol, and conducting the scoring in a matter of seconds.
According to the researchers, deep learning enables automatic sleep staging for suspected OSA patients with a high accuracy. The study was published in IEEE Journal of Biomedical and Health Informatics.
The Sleep Technology and Analytics Group, STAG, at the University of Eastern Finland solves sleep diagnostics challenges by using a variety of different approaches. The methods developed by the group are based on wearable, non-intrusive sensors, better diagnostic parameters and modern computational solutions that are based on artificial intelligence. The new methods developed by the group are expected to significantly improve OSA severity assessment, promote individualised treatment planning and more reliable prediction of OSA-related daytime symptoms and comorbidities.
Source:
University of Eastern Finland
Media Contacts:
Sami Myllymaa – University of Eastern Finland
Image Source:
The image is credited to Juha Rutanen.
Original Research: Open access
“Accurate Deep Learning-Based Sleep Staging in a Clinical Population with Suspected Obstructive Sleep Apnea”. Henri Korkalainen et al.
IEEE Journal of Biomedical and Health Informatics doi:10.1109/JBHI.2019.2951346.
Abstract
Accurate Deep Learning-Based Sleep Staging in a Clinical Population with Suspected Obstructive Sleep Apnea
The identification of sleep stages is essential in the diagnostics of sleep disorders, among which obstructive sleep apnea (OSA) is one of the most prevalent. However, manual scoring of sleep stages is time-consuming, subjective, and costly. To overcome this shortcoming, we aimed to develop an accurate deep learning approach for automatic classification of sleep stages and to study the effect of OSA severity on the classification accuracy. Overnight polysomnographic recordings from a public dataset of healthy individuals (Sleep-EDF, n=153) and from a clinical dataset (n=891) of patients with suspected OSA were used to develop a combined convolutional and long short-term memory neural network. On the public dataset, the model achieved sleep staging accuracy of 83.7% (κ=0.77) with a single frontal EEG channel and 83.9% (κ=0.78) when supplemented with EOG. For the clinical dataset, the model achieved accuracies of 82.9% (κ=0.77) and 83.8% (κ=0.78) with a single EEG channel and two channels (EEG+EOG), respectively. The sleep staging accuracy decreased with increasing OSA severity. The single-channel accuracy ranged from 84.5% (κ=0.79) for individuals without OSA diagnosis to 76.5% (κ=0.68) for severe OSA patients. In conclusion, deep learning enables automatic sleep staging for suspected OSA patients with high accuracy and expectedly, the accuracy lowered with increasing OSA severity. Furthermore, the accuracies achieved in the public dataset were superior to previously published state-of-the-art methods. Adding an EOG channel did not significantly increase the accuracy. The automatic, single-channel-based sleep staging could enable easy, accurate, and cost-efficient integration of EEG recording into diagnostic ambulatory recordings.