Summary: As social robotics is becoming more popular, researchers explore how people react to the technology. They report people show significantly stronger liking to faulty robots than those that interact perfectly.
Source: Frontiers.
New social robotics research finds that humans prefer interacting with faulty robots significantly more than with robots that function and behave flawlessly.
It has been argued that the ability of humans to recognize social signals is crucial to mastering social intelligence – but can robots learn to read human social cues and adapt or correct their own behavior accordingly?
In a recent study, researchers examined how people react to robots that exhibit faulty behavior compared to perfectly performing robots. The results, published in Frontiers in Robotics and AI, show that the participants took a significantly stronger liking to the faulty robot than the robot that interacted flawlessly.
“Our results show that decoding a human’s social signals can help the robot understand that there is an error and subsequently react accordingly,” says corresponding author Nicole Mirnig, PhD candidate at the Center for Human-Computer Interaction, University of Salzburg, Austria.
Although social robotics is a rapidly advancing field, social robots are not yet at a technical level where they operate without making errors. Nevertheless, most studies in the field are based on the assumption of faultlessly performing robots. “Alternatives resulting from unforeseeable conditions that develop during an experiment are often not further regarded or simply excluded,” says Nicole Mirnig. “It lies within the nature of thorough scientific research to pursue a strict code of conduct. However, we suppose that faulty instances of human-robot interaction are full with knowledge that can help us further improve the interactional quality in new dimensions. We think that because most research focuses on perfect interaction, many potentially crucial aspects are overlooked.”
To examine the human interaction partners’ social signals following a robot error, the research team purposefully programmed faulty behavior into a human-like NAO robot’s routine and let the participants interact with it. They measured the robot’s likability, anthropomorphism and perceived intelligence, and analyzed the users’ reaction when the robot made a mistake. By means of video coding, the researchers could replicate their findings from earlier studies and show that humans respond to faulty robot behavior with social signals. Through interviews and user ratings, the research team found that somewhat surprisingly, erroneous robots were not perceived as significantly less intelligent or anthropomorphic compared to perfectly performing robots. Instead, although the humans recognized the faulty robot’s mistakes, they actually rated it as more likeable than its perfectly performing counterpart.
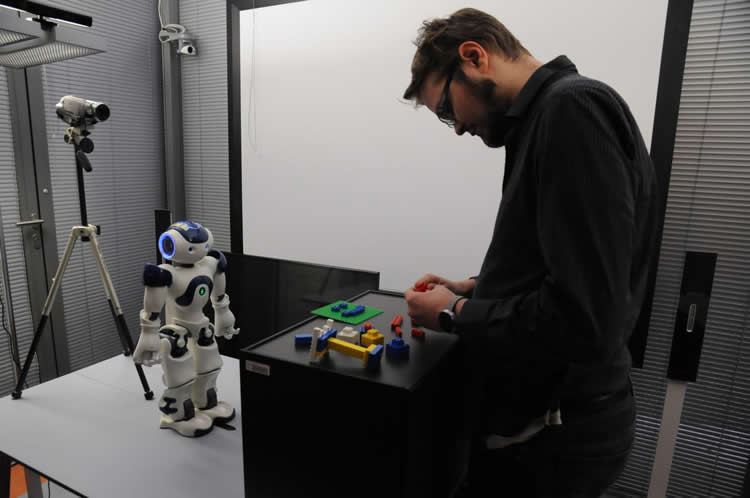
“Our results showed that the participants liked the faulty robot significantly more than the flawless one. This finding confirms the Pratfall Effect, which states that people’s attractiveness increases when they make a mistake,” says Nicole Mirnig. “Specifically exploring erroneous instances of interaction could be useful to further refine the quality of human-robotic interaction. For example, a robot that understands that there is a problem in the interaction by correctly interpreting the user’s social signals, could let the user know that it understands the problem and actively apply error recovery strategies.”
These findings have exciting implications for the field of social robotics, since they emphasize the importance for robot creators to keep potential imperfections in mind when designing robots. As opposed to assuming that a robot will behave perfectly, embracing the flaws of social robot technology might pave way for the development of robots that make mistakes – and learn from them. This would also make the robots more likeable to humans. “Studying the sources of imperfect robot behavior will lead to more believable robot characters and more natural interaction,” concludes Nicole Mirnig.
Funding: The research was funded by the Austrian Federal Ministry of Economy, Family and Youth, National Foundation for Research, Technology and Development.
Source: Melissa Cochrane – Frontiers
Image Source: NeuroscienceNews.com image is credited to Center for Human-Computer Interaction.
Original Research: Full open access research for “To Err Is Robot: How Humans Assess and Act toward an Erroneous Social Robot” by Nicole Mirnig, Gerald Stollnberger, Markus Miksch, Susanne Stadler, Manuel Giuliani and Manfred Tscheligi in Frontiers in Robotics and AI. Published online May 31 2017 doi:10.3389/frobt.2017.00021
[cbtabs][cbtab title=”MLA”]Frontiers “Why Humans Find Faulty Robots More Likable.” NeuroscienceNews. NeuroscienceNews, 4 August 2017.
<faulty-robots-likeable-7239/>.[/cbtab][cbtab title=”APA”]Frontiers (2017, August 4). Why Humans Find Faulty Robots More Likable. NeuroscienceNew. Retrieved August 4, 2017 from faulty-robots-likeable-7239/[/cbtab][cbtab title=”Chicago”]Frontiers “Why Humans Find Faulty Robots More Likable.” faulty-robots-likeable-7239/ (accessed August 4, 2017).[/cbtab][/cbtabs]
Abstract
To Err Is Robot: How Humans Assess and Act toward an Erroneous Social Robot
We conducted a user study for which we purposefully programmed faulty behavior into a robot’s routine. It was our aim to explore if participants rate the faulty robot different from an error-free robot and which reactions people show in interaction with a faulty robot. The study was based on our previous research on robot errors where we detected typical error situations and the resulting social signals of our participants during social human–robot interaction. In contrast to our previous work, where we studied video material in which robot errors occurred unintentionally, in the herein reported user study, we purposefully elicited robot errors to further explore the human interaction partners’ social signals following a robot error. Our participants interacted with a human-like NAO, and the robot either performed faulty or free from error. First, the robot asked the participants a set of predefined questions and then it asked them to complete a couple of LEGO building tasks. After the interaction, we asked the participants to rate the robot’s anthropomorphism, likability, and perceived intelligence. We also interviewed the participants on their opinion about the interaction. Additionally, we video-coded the social signals the participants showed during their interaction with the robot as well as the answers they provided the robot with. Our results show that participants liked the faulty robot significantly better than the robot that interacted flawlessly. We did not find significant differences in people’s ratings of the robot’s anthropomorphism and perceived intelligence. The qualitative data confirmed the questionnaire results in showing that although the participants recognized the robot’s mistakes, they did not necessarily reject the erroneous robot. The annotations of the video data further showed that gaze shifts (e.g., from an object to the robot or vice versa) and laughter are typical reactions to unexpected robot behavior. In contrast to existing research, we assess dimensions of user experience that have not been considered so far and we analyze the reactions users express when a robot makes a mistake. Our results show that decoding a human’s social signals can help the robot understand that there is an error and subsequently react accordingly.
“To Err Is Robot: How Humans Assess and Act toward an Erroneous Social Robot” by Nicole Mirnig, Gerald Stollnberger, Markus Miksch, Susanne Stadler, Manuel Giuliani and Manfred Tscheligi in Frontiers in Robotics and AI. Published online May 31 2017 doi:10.3389/frobt.2017.00021