Summary: Researchers unravel the mysteries of smell using machine learning. Their AI model has achieved human-level skill in describing how certain chemicals will smell, closing a critical gap in the scientific understanding of olfaction.
Beyond advancing our comprehension of smell, this technology could lead to breakthroughs in the fragrance and flavor industries, and even help create new functional scents like mosquito repellents. The study validates a first-of-its-kind data-driven map of human olfaction, which correlates chemical structure to odor perception.
Key Facts:
- The AI model was trained using an industry dataset of 5,000 known odorants, enabling it to predict smell descriptors based on a molecule’s structure.
- Human panelists trained in the study, when compared to the AI model, found that the model outperformed individual human assessments for 53% of the molecules tested.
- The AI model also displayed capabilities it was not trained for, like accurately predicting the strength of odors, revealing its potential for broader olfactory tasks.
Source: Monell Chemical Senses Center
A main crux of neuroscience is learning how our senses translate light into sight, sound into hearing, food into taste, and texture into touch. Smell is where these sensory relationships get more complex and perplexing.
To address this question, a research team co-led by the Monell Chemical Senses Center and start-up Osmo, a Cambridge, Mass.-based company spun out of machine learning research done at Google Research, are investigating how airborne chemicals connect to odor perception in the brain.
To this end they discovered that a machine-learning model has achieved human-level proficiency at describing, in words, how chemicals might smell.
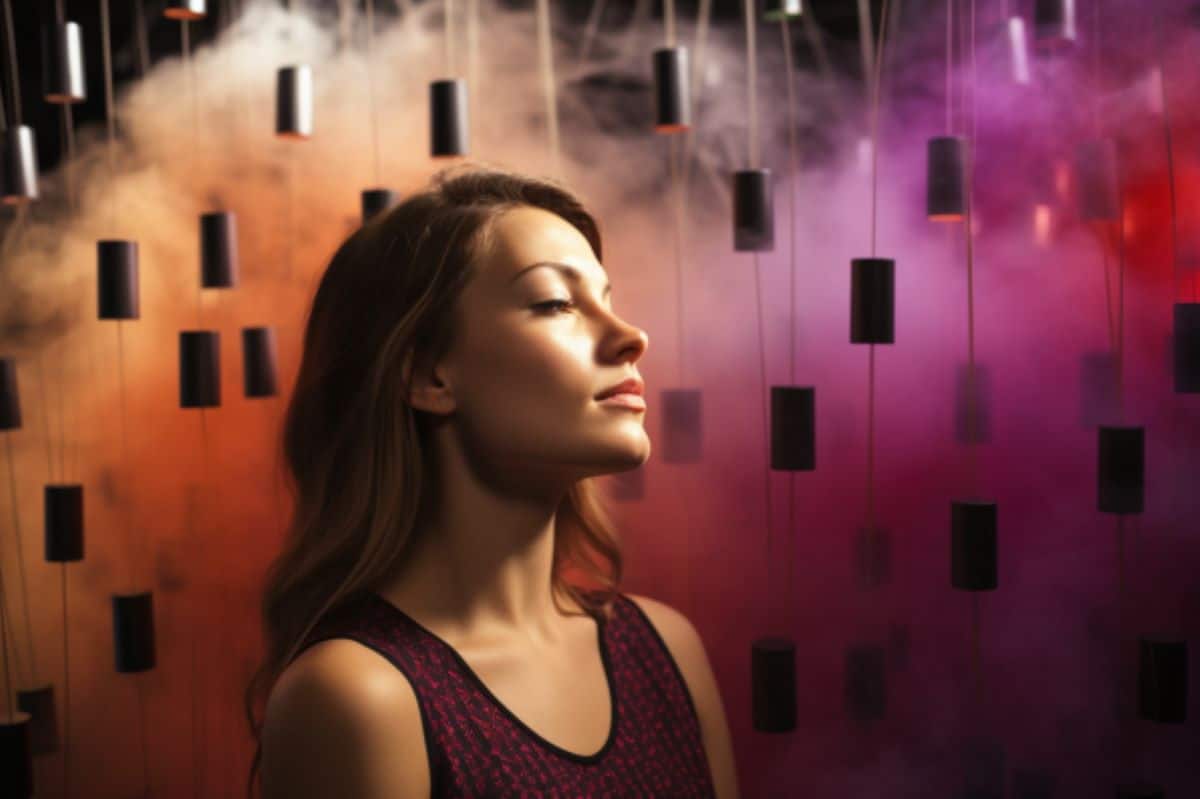
Their research appears in the September 1 issue of Science.
“The model addresses age-old gaps in the scientific understanding of the sense of smell,” said senior co-author Joel Mainland, PhD, Monell Center Member. This collaboration moves the world closer to digitizing odors to be recorded and reproduced. It also may identify new odors for the fragrance and flavor industry that could not only decrease dependence on naturally sourced endangered plants, but also identify new functional scents for such uses as mosquito repellent or malodor masking.
“How our brains and noses work together”
Humans have about 400 functional olfactory receptors. These are proteins at the end of olfactory nerves that connect with airborne molecules to transmit an electrical signal to the olfactory bulb. The number of olfactory receptors is much more than we use for color vision – four – or even taste – about 40.
“In olfaction research, however, the question of what physical properties make an airborne molecule smell the way it does to the brain has remained an enigma,” said Mainland. “But if a computer can discern the relationship between how molecules are shaped and how we ultimately perceive their odors, scientists could use that knowledge to advance the understanding of how our brains and noses work together.”
To address this, Osmo CEO Alex Wiltschko, PhD and his team created a model that learned how to match the prose descriptions of a molecule’s odor with the odor’s molecular structure. The resulting map of these interactions is essentially groupings of similarly smelling odors, like floral sweet and candy sweet.
“Computers have been able to digitize vision and hearing, but not smell – our deepest and oldest sense,” said Wiltschko. “This study proposes and validates a novel data-driven map of human olfaction, matching chemical structure to odor perception.”
What is the smell of garlic or of ozone?
The model was trained using an industry dataset that included the molecular structures and odor qualities of 5,000 known odorants. Data input is the shape of a molecule, and the output is a prediction of which odor words best describe its smell.
To ascertain the efficacy of the model, researchers at Monell conducted a blind validation procedure in which a panel of trained research participants described new molecules, and then compared their answers with the model’s description. The 15 panelists were each given 400 odorants as well as trained to use a set of 55 words – from mint to musty – to describe each molecule.
“Our confidence in this model can only be as good as our confidence in the data we used to test it,” said co-first author Emily Mayhew, PhD, who conducted this research while a Monell postdoctoral fellow. She is now an assistant professor at Michigan State University. Brian K. Lee, PhD, Google Research, Brain Team, Cambridge, Mass., is also a co-first author.
The Monell team supplied panelists with lab-designed odor reference kits to teach them how to recognize the smells and select the most appropriate words to describe their perception. To avoid pitfalls from past studies like panelist conflation of “musty,” like a wet basement, and “musky,” like a perfume, training sessions and lab-designed odor reference kits taught each panelist the odor quality associated with each descriptive term.
The panelists were asked to select which of the 55 descriptors applied and to rate the extent to which the term best applied to the odor on a 1-to-5 scale for each of the 400 odors. For example, one panelist rated the smell of the previously uncharacterized odorant 2,3-dihydrobenzofuran-5-carboxaldehyde as very powdery (5) and somewhat sweet (3).
Quality control is also important in the final comparison of the human sniffers to the computer model. That’s where co-author Jane Parker, PhD, Professor of Flavour Chemistry, University of Reading, UK comes in. “I’ve worked on smell for many years, relying mainly on my own nose to describe aromas.”
Her team verified the purity of samples used to test the model’s prediction. First, gas chromatography enabled them to separate out each compound in a sample, including any impurities. Next, Parker and her team smelled each separated compound to determine whether any impurity is overwhelming the target molecule’s known odor.
“We did find a few samples with significant impurities, among the 50 tested,” Parker said. In one case, the impurity was from traces of a reagent used in the synthesis of the target molecule and gave the sample a distinctive buttery smell that overpowered the odorant of interest. “In this case we were able to explain why the panel had described the smell differently to the AI prediction.”
Better Than a Human?
In comparing the model’s performance to that of individual panelists, the model achieved better predictions of the average of the group’s odor ratings than any single panelist in the study, impurities aside. Specifically, the model performed better than the average panelist for 53% of the molecules tested.
“The most surprising result, however, is that the model succeeded at olfactory tasks it was not trained to do,” said Mainland. “The eye-opener was that we never trained it to learn odor strength, but it could nonetheless make accurate predictions.”
The model was able to identify dozens of pairs of structurally dissimilar molecules that had counter-intuitively similar smells, and characterize a wide variety of odor properties, such as odor strength, for 500,000 potential scent molecules. “We hope this map will be useful to researchers in chemistry, olfactory neuroscience, and psychophysics as a new tool for investigating the nature of olfactory sensation,” said Mainland.
What’s next? The team surmises that the model map may be organized based on metabolism, which would be a fundamental shift in how scientists think about odors. In other words, odors that are close to each other on the map, or perceptually similar, are also more likely to be metabolically related. Sensory scientists currently organize molecules the way a chemist would, for example, asking does it have an ester or an aromatic ring?
“Our brains don’t organize odors in this way,” said Mainland. “Instead, this map suggests that our brains may organize odors according to the nutrients from which they derive.”
Funding: This research was funded in part by Google Research and National Institutes of Health grants F32 DC019030 and T32 DC000014.
About this AI and olfaction research news
Author: Karen Kreeger
Source: Monell Chemical Senses Center
Contact: Karen Kreeger – Monell Chemical Senses Center
Image: The image is credited to Neuroscience News
Original Research: Closed access.
“A principal odor map unifies diverse tasks in olfactory perception” by Joel Mainland et al. Science
Abstract
A principal odor map unifies diverse tasks in olfactory perception
Mapping molecular structure to odor perception is a key challenge in olfaction. We used graph neural networks to generate a principal odor map (POM) that preserves perceptual relationships and enables odor quality prediction for previously uncharacterized odorants.
The model was as reliable as a human in describing odor quality: On a prospective validation set of 400 out-of-sample odorants, the model-generated odor profile more closely matched the trained panel mean than did the median panelist.
By applying simple, interpretable, theoretically rooted transformations, the POM outperformed chemoinformatic models on several other odor prediction tasks, indicating that the POM successfully encoded a generalized map of structure-odor relationships.
This approach broadly enables odor prediction and paves the way toward digitizing odors.