Summary: Researchers have devised an innovative machine learning model that uses retinal images to distinguish normal cognitive function from mild cognitive impairment. This model represents a new, non-invasive, and cost-effective method to detect early signs of cognitive decline, which could lead to Alzheimer’s disease.
The model identifies unique features in retinal images obtained via optical coherence tomography and angiography (OCT/OCTA), successfully discerning individuals with mild cognitive impairment. The method signifies an essential stride toward early detection of cognitive impairment before it escalates to Alzheimer’s dementia.
Key Facts:
- The machine learning model shows a sensitivity of 79% and specificity of 83% in distinguishing individuals with mild cognitive impairment from those with normal cognition, using retinal images and patient data.
- This study represents the first application of retinal OCT/OCTA images to differentiate individuals with mild cognitive impairment from those with normal cognition.
- The Duke Health team previously developed a similar model that used retinal scans and associated data to identify patients with an existing Alzheimer’s diagnosis.
Source: Duke University
A machine learning model developed by Duke Health researchers can differentiate normal cognition from mild cognitive impairment using retinal images from the eye.
The model analyzes retinal images and associated data and recognizes specific features to identify individuals with mild cognitive impairment.
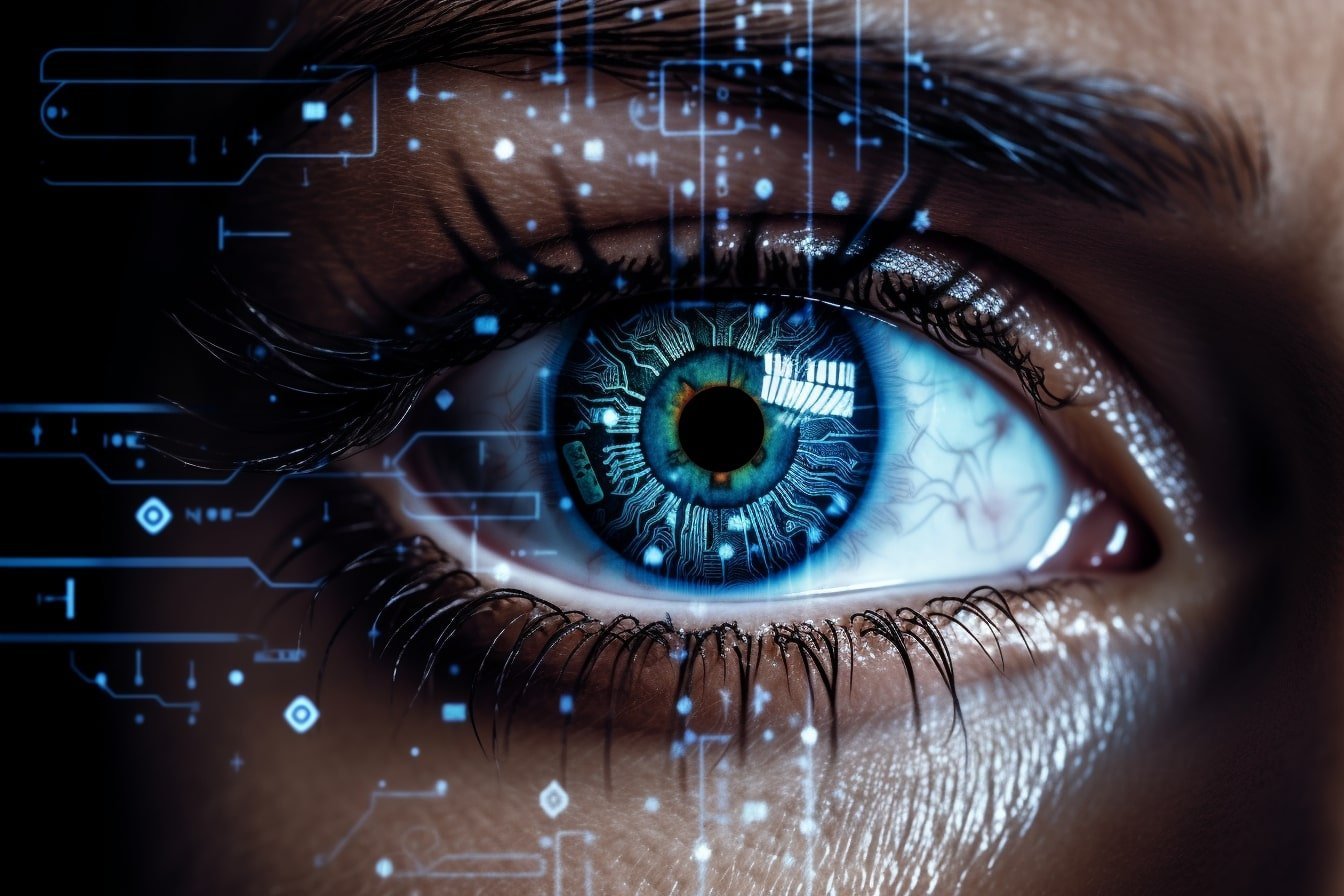
Publishing in the journal Ophthalmology Science, the model demonstrates the potential for a non-invasive and inexpensive method of identifying the early signs of cognitive impairment that could progress to Alzheimer’s disease.
“This is particularly exciting work because we have previously been unable to differentiate mild cognitive impairment from normal cognition in previous models,” said senior author Sharon Fekrat, M.D., professor in Duke’s departments of Ophthalmology and Neurology, and associate professor in the Department of Surgery.
“This work brings us one step closer to detecting cognitive impairment earlier before it progresses to Alzheimer’s dementia.”
Fekrat and colleagues previously developed a model that used retinal scans and other data to successfully identify patients with a known Alzheimer’s diagnosis. The scans – based on optical coherence tomography (OCT) and OCT angiography (OCTA) — detected structural changes in the neurosensory retina and its microvasculature among Alzheimer’s patients.
The current study expands on that work, using machine learning techniques to detect mild cognitive impairment, which is often a precursor to Alzheimer’s.
The new model identifies specific features in the OCT and OCTA images that signal the presence of cognitive impairment, along with patient data such as age, sex, visual acuity, and years of education and quantitative data from the images themselves.
The researchers reported that the model analyzed retinal pictures and images along with quantitative data to differentiate people with normal cognition from those with a diagnosis of mild cognitive impairment with a sensitivity of 79% and specificity of 83%.
“This is the first study to use retinal OCT and OCTA images to distinguish people with mild cognitive impairment from individuals with normal cognition,” said co-first author C. Ellis Wisely, M.D., assistant professor in the Department of Ophthalmology.
“Having a non-invasive and less expensive means to reliably identify these patients is increasingly important, particularly as new therapies for Alzheimer’s disease may become available,” Wisely said.
“The retina is a window to the brain, and machine learning algorithms that leverage non-invasive and cost-effective retinal imaging to assess neurological health can be a potent tool to screen patients at scale,” said co-lead author Alexander Richardson, a student in the Eye Multimodal Imaging in Neurodegenerative Disease lab at Duke.
In addition to Fekrat, Wisely and Richardson, study authors include Ricardo Henao, Cason B. Robbins, Justin P. Ma, Dong Wang, Kim G. Johnson, Andy J. Liu, and Dilraj S. Grewal.
Funding: The study received support in part from the Alzheimer’s Drug Discovery Foundation.
About this machine learning and dementia research news
Author: Sarah Avery
Source: Duke University
Contact: Sarah Avery – Duke University
Image: The image is credited to Neuroscience News
Original Research: Open access.
“A convolutional neural network using multimodal retinal imaging for differentiation of mild cognitive impairment from normal cognition” by Sharon Fekrat et al. Ophthalmology Science
Abstract
A convolutional neural network using multimodal retinal imaging for differentiation of mild cognitive impairment from normal cognition
Mild cognitive impairment (MCI), often considered the clinical precursor to Alzheimer’s disease (AD), is an intermediate state where cognitive impairment is present, but the ability to perform activities of daily living is preserved.
MCI can be further classified into amnestic and non-amnestic types, with amnestic MCI referring to a state where memory is affected more significantly than other domains of cognitive function (e.g. executive, language, or visuospatial).
Most individuals diagnosed with MCI progress to AD; however, patients with amnestic MCI are more likely to progress to AD than individuals with non-amnestic disease.
Some estimates indicate amnestic MCI patients may progress to AD at rates of approximately 20% per year. Thus, early identification of MCI is important for effective intervention, particularly as new therapies may become available.
Given the existing unmet need of accurately establishing an MCI diagnosis, especially in those who will progress to AD, in the setting of an aging worldwide population, reliable biomarkers that are also non-invasive and widely available are needed to support the clinical diagnosis of MCI.