Summary: A new AI algorithm inspired by the genome’s ability to compress vast information offers insights into brain function and potential tech applications. Researchers found that this algorithm performs tasks like image recognition and video games almost as effectively as fully trained AI networks.
By mimicking how genomes encode complex behaviors with limited data, the model highlights the evolutionary advantage of efficient information compression. The findings suggest new pathways for developing advanced, lightweight AI systems capable of running on smaller devices like smartphones.
Key Facts:
- The AI algorithm compresses information like genomes, enabling high efficiency.
- It performs tasks nearly as effectively as fully trained state-of-the-art AI.
- Potential applications include running large AI models on devices like smartphones.
Source: CSHL
In a sense, each of us begins life ready for action. Many animals perform amazing feats soon after they’re born. Spiders spin webs. Whales swim. But where do these innate abilities come from?
Obviously, the brain plays a key role as it contains the trillions of neural connections needed to control complex behaviors. However, the genome has space for only a small fraction of that information.
This paradox has stumped scientists for decades. Now, Cold Spring Harbor Laboratory (CSHL) Professors Anthony Zador and Alexei Koulakov have devised a potential solution using artificial intelligence.
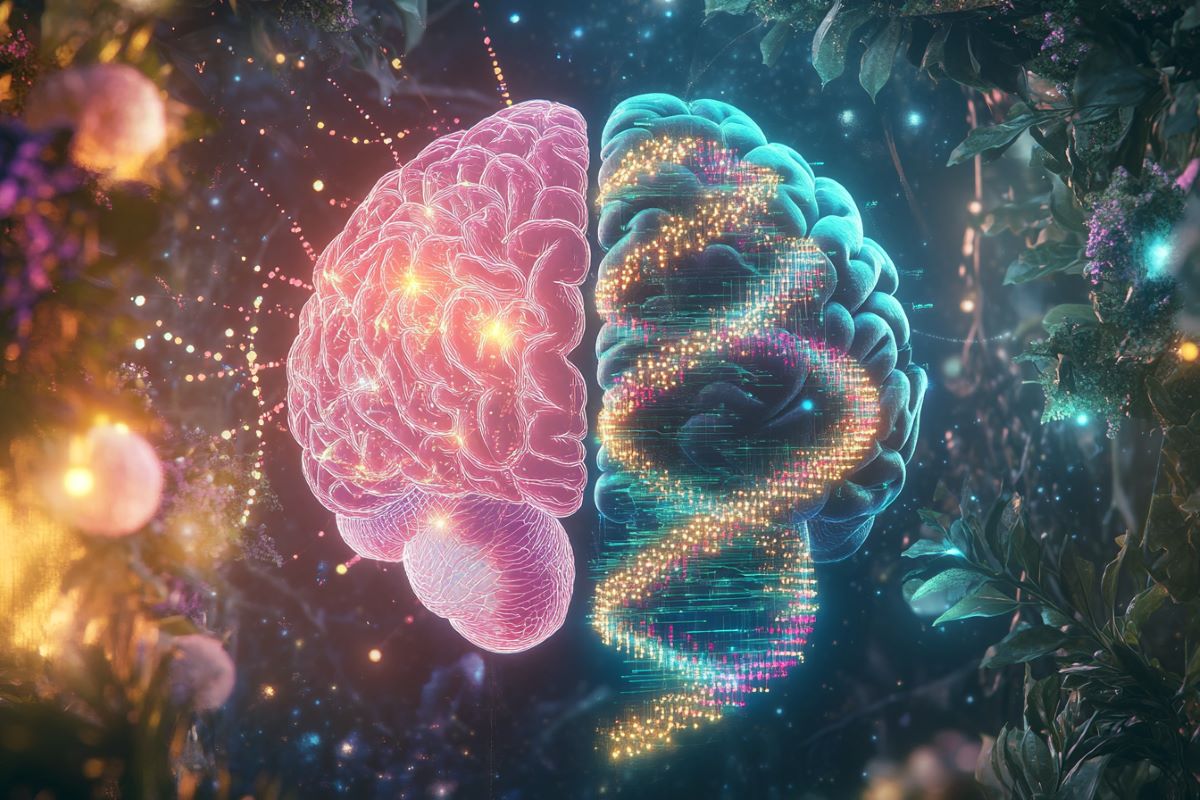
When Zador first encounters this problem, he puts a new spin on it. “What if the genome’s limited capacity is the very thing that makes us so smart?” he wonders. “What if it’s a feature, not a bug?”
In other words, maybe we can act intelligently and learn quickly because the genome’s limits force us to adapt. This is a big, bold idea—tough to demonstrate. After all, we can’t stretch lab experiments across billions of years of evolution. That’s where the idea of the genomic bottleneck algorithm emerges.
In AI, generations don’t span decades. New models are born with the push of a button. Zador, Koulakov, and CSHL postdocs Divyansha Lachi and Sergey Shuvaev set out to develop a computer algorithm that folds heaps of data into a neat package—much like our genome might compress the information needed to form functional brain circuits.
They then test this algorithm against AI networks that undergo multiple training rounds. Amazingly, they find the new, untrained algorithm performs tasks like image recognition almost as effectively as state-of-the-art AI. Their algorithm even holds its own in video games like Space Invaders. It’s as if it innately understands how to play.
Does this mean AI will soon replicate our natural abilities?
“We haven’t reached that level,” says Koulakov. “The brain’s cortical architecture can fit about 280 terabytes of information—32 years of high-definition video. Our genomes accommodate about one hour. This implies a 400,000-fold compression technology cannot yet match.”
Nevertheless, the algorithm allows for compression levels thus far unseen in AI. That feature could have impressive uses in tech. Shuvaev, the study’s lead author, explains: “For example, if you wanted to run a large language model on a cell phone, one way [the algorithm] could be used is to unfold your model layer by layer on the hardware.”
Such applications could mean more evolved AI with faster runtimes. And to think, it only took 3.5 billion years of evolution to get here.
About this AI, genetics, and evolution research news
Author: Samuel Diamond
Source: CSHL
Contact: Samuel Diamond – CSHL
Image: The image is credited to Neuroscience News
Original Research: Open access.
“Encoding innate ability through a genomic bottleneck” by Anthony Zador et al. PNAS
Abstract
Encoding innate ability through a genomic bottleneck
Animals are born with extensive innate behavioral capabilities, which arise from neural circuits encoded in the genome.
However, the information capacity of the genome is orders of magnitude smaller than that needed to specify the connectivity of an arbitrary brain circuit, indicating that the rules encoding circuit formation must fit through a “genomic bottleneck” as they pass from one generation to the next.
Here, we formulate the problem of innate behavioral capacity in the context of artificial neural networks in terms of lossy compression of the weight matrix.
We find that several standard network architectures can be compressed by several orders of magnitude, yielding pretraining performance that can approach that of the fully trained network.
Interestingly, for complex but not for simple test problems, the genomic bottleneck algorithm also captures essential features of the circuit, leading to enhanced transfer learning to novel tasks and datasets.
Our results suggest that compressing a neural circuit through the genomic bottleneck serves as a regularizer, enabling evolution to select simple circuits that can be readily adapted to important real-world tasks.
The genomic bottleneck also suggests how innate priors can complement conventional approaches to learning in designing algorithms for AI.