Summary: Humans have an exceptional ability to learn socially, but most studies have focused on overly simplified tasks. In a new study using Minecraft, researchers found that the most successful participants dynamically balanced individual exploration and social learning. Adaptability, rather than relying solely on one strategy, predicted better performance across various environments.
By using visual field tracking and computational modeling, scientists could precisely predict players’ decisions. The findings close a key gap in understanding how humans integrate social and asocial learning in realistic settings. This research also opens new pathways for designing better educational and social information systems.
Key Facts:
- Adaptability Matters: Flexible switching between social and individual learning strategies was the best predictor of success.
- Realistic Testing: Researchers used Minecraft and detailed visual tracking to simulate and study real-world learning dynamics.
- Bridging a Gap: The study closes a decades-long gap between research on individual and social learning mechanisms.
Source: TUB
The ability to learn socially from one another is a defining feature of the human species. Social learning enables humans to gradually accumulate information across generations.
Although we are able to build cities full of skyscrapers, send people into space, and collectively develop cures for diseases, most studies investigating social learning mechanisms focus on relatively simple, abstract tasks that bear little resemblance to real-world social learning environments.
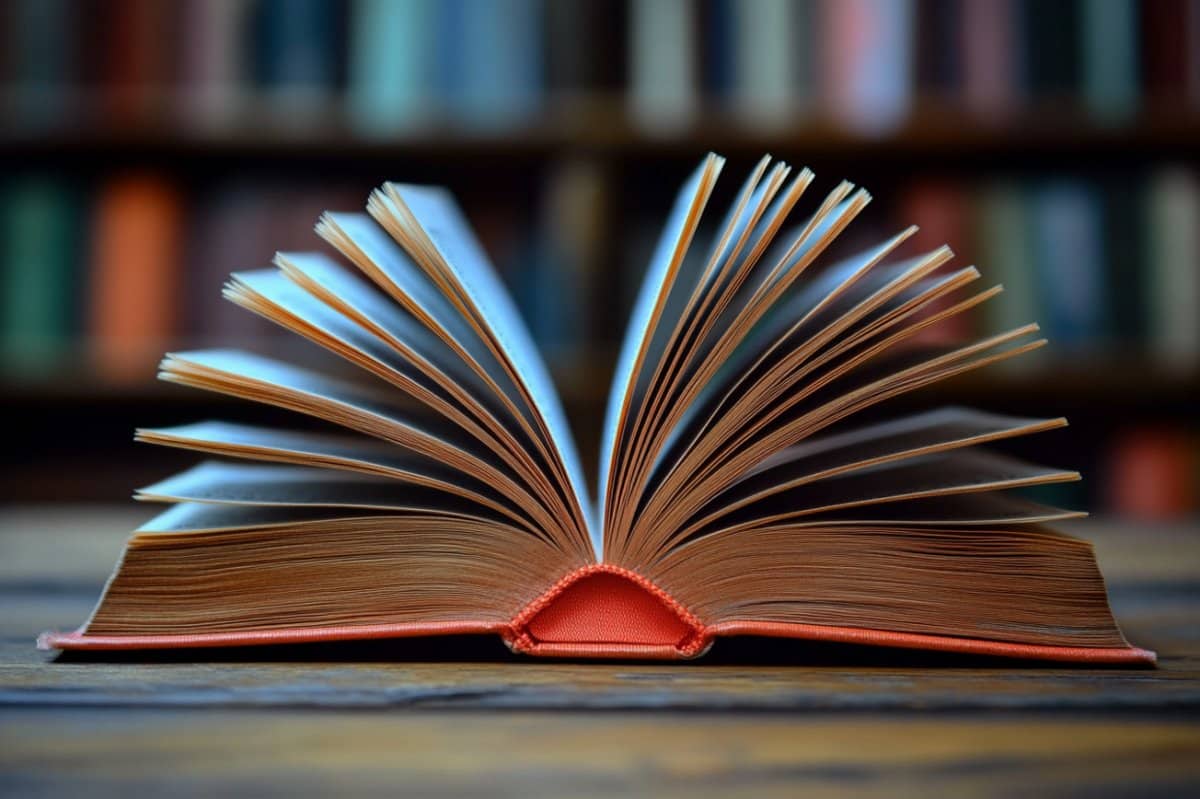
As a result, little is known about how humans dynamically integrate asocial and social information in realistic, real-world contexts.
To investigate this, an international team of scientists from the Cluster of Excellence Science of Intelligence (SCIoI), the Max Planck Institute for Human Development (MPIB), the University of Tübingen, and NYU developed a virtual foraging task programmed in the popular video game Minecraft.
In their study, published in Nature Communications, they found that adaptability (i.e. flexibly using asocial and social learning strategies, rather than fixed strategies) is the most important driver of success.
“Should I explore on my own or work with the group?”
In the experiment, each participant controls an avatar that destroys Minecraft blocks in order to find resources. Whenever a resource is discovered, a blue splash appears, which is visible to other players, and could potentially provide useful social information about the location of further resources.
At the beginning of each round, the players are informed of whether they will be working alone or in a group of four people who can interact with each other in real-time. Additionally, they are tested across two types of environments.
In “patchy” environments, resources are clustered together, which means that participants can find numerous blocks containing resources close to each other, while in “random” environments resources are spread out.
Thus, social information is particularly valuable in “patchy” environments, since it may reveal other rewards nearby. However, social information has no value in “random” environments, since there is no learnable pattern of resource locations.
Each player tries to maximize their own rewards, rather than working towards a collective goal, and thus needs to effectively find rewards using the right balance of individual and social learning strategies.
“Using a game like Minecraft is useful because it simulates real-life challenges. For instance, since you can only see a small part of the game world at a time, you must choose whether to focus on searching on your own or pay attention to what the other players are doing to learn from them,” said Ralf Kurvers, the senior author of the study.
“This means that I am constantly faced with a choice: do I follow my own instinct and go search alone, or do I utilize social information (in this case, the blue “splashes”) by following the players who’ve already found something, as they are likely to have found a resource patch?”
New tools for studying the interaction between individual and social learning
Through a newly developed computational method for automating the transcription of visual field data, the scientists measured which objects, events, and other players were observed by each participant, recorded at a rate of 20 times per second.
They created a model that brings together where people look, how they move, and the choices they make when foraging.
“In simpler terms, we can now predict which block a participant will choose next by combining individual and social learning strategies, all in one computational framework,” explained Charley Wu from the University of Tübingen.
“This new approach allows us to connect the learning algorithms that power modern AI with flexible social learning mechanisms that adaptively learn from the successful behaviors of others.”
Why this matters
Altogether, the study bridges a decades-long gap between research on individual and social learning. The results show that humans are not just passive imitators or stubborn individual learners.
Rather, they dynamically balance these strategies; adaptive mechanisms of individual and social learning amplify one another, and are driven by a common currency of individual performance.
Furthermore, the extent to which each individual was able to adapt their individual and social learning strategies was the best predictor of their performance. This emphasizes that adaptability, rather than fixed strategies, is what drives human intelligence.
Future implications
This work advances our understanding of the cognitive mechanisms underlying adaptive learning and decision-making in social contexts, opening new pathways for understanding how information spreads in groups, how new innovations emerge, and gives clues on how to design systems that better foster adaptive learning in social settings.
In brief:
• The study shows that adaptability, i.e. the flexible switching between individual and social learning, is crucial for success.
- The researchers used the video game Minecraft to study social learning processes in a dynamic, realistic environment.
- Using a new computer-based method for capturing visual field data, individual and social learning strategies could be precisely modelled and predicted.
- The results close a research gap and show that people dynamically adapt learning strategies – an important factor for the design of learning environments and information dissemination in social groups.
About this adaptive learning and cognitive flexibility research news
Author: Solveig Steinhardt
Source: TUB
Contact: Solveig Steinhardt – TUB
Image: The image is credited to Neuroscience News
Original Research: Open access.
“Adaptive mechanisms of social and asocial learning in immersive collective foraging” by Ralf Kurvers et al. Nature Communications
Abstract
Adaptive mechanisms of social and asocial learning in immersive collective foraging
Human cognition is distinguished by our ability to adapt to different environments and circumstances. Yet the mechanisms driving adaptive behavior have predominantly been studied in separate asocial and social contexts, with an integrated framework remaining elusive.
Here, we use a collective foraging task in a virtual Minecraft environment to integrate these two fields, by leveraging automated transcriptions of visual field data combined with high-resolution spatial trajectories.
Our behavioral analyses capture both the structure and temporal dynamics of social interactions, which are then directly tested using computational models sequentially predicting each foraging decision.
These results reveal that adaptation mechanisms of both asocial foraging and selective social learning are driven by individual foraging success (rather than social factors).
Furthermore, it is the degree of adaptivity—of both asocial and social learning—that best predicts individual performance.
These findings not only integrate theories across asocial and social domains, but also provide key insights into the adaptability of human decision-making in complex and dynamic social landscapes.