Summary: Findings allow for the development of an autonomously updating brain-machine interface, which is able to improve on its own by learning about its subject without additional programming. The system could help develop new robotic prosthetics, which can perform more naturally.
Source: University of Houston
A University of Houston engineer is reporting in eNeuro that a brain-computer interface, a form of artificial intelligence, can sense when its user is expecting a reward by examining the interactions between single-neuron activities and the information flowing to these neurons, called the local field potential.
Professor of biomedical engineering Joe Francis reports his team’s findings allow for the development of an autonomously updating brain-computer interface (BCI) that improves on its own, learning about its subject without having to be programed.
The findings potentially have applications for robotic prosthetics, which would sense what a user wants to do (pick up a glass, for example) and do it. The work represents a significant step forward for prosthetics that perform more naturally.
“This will help prosthetics work the way the user wants them to,” said Francis.
“The BCI quickly interprets what you’re going to do and what you expect as far as whether the outcome will be good or bad.” Francis said that information drives scientists’ abilities to predict reward outcome to 97%, up from the mid-70s.
To understand the effects of reward on the brain’s primary motor cortex activity, Francis used implanted electrodes to investigate brainwaves and spikes in brain activity while tasks were performed to see how interactions are modulated by conditioned reward expectations.
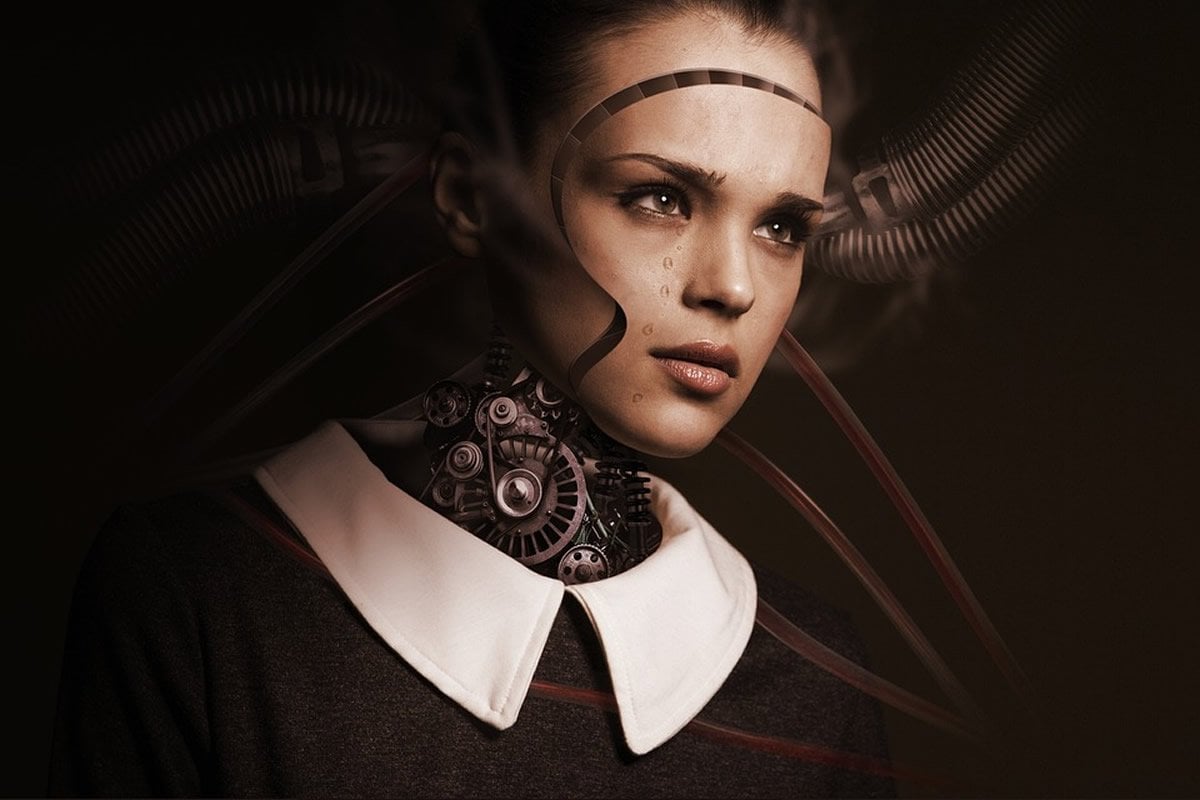
“We assume intention is in there, and we decode that information by an algorithm and have it control either a computer cursor, for example, or a robotic arm,” said Francis. Interestingly even when the task called for no movement, just passively observing an activity, the BCI was able to determine intention because the pattern of neural activity resembled that during movement.
“This is important because we are going to have to extract this information and brain activity out of people who cannot actually move, so this is our way of showing we can still get the information even if there is no movement,” said Francis. This process utilizes mirror neurons, which fire when action is taken and action is observed.
“This examination of reward motivation in the primary motor cortex could be useful in developing an autonomously updating brain machine interface,” said Francis.
Source:
University of Houston
Media Contacts:
Laurie Fickman – University of Houston
Image Source:
The image is in the public domain.
Original Research: Closed access
“Reward Expectation Modulates Local Field Potentials, Spiking Activity and Spike-Field Coherence in the Primary Motor Cortex”. Junmo An, Taruna Yadav, John P. Hessburg and Joseph T. Francis.
eNeuro. doi:10.1523/ENEURO.0178-19.2019
Abstract
Reward Expectation Modulates Local Field Potentials, Spiking Activity and Spike-Field Coherence in the Primary Motor Cortex
Reward modulation of the primary motor cortex (M1) could be exploited in developing an autonomously updating brain-computer interface (BCI) based on a reinforcement learning (RL) architecture. For an autonomously updating RL based BCI system, we would need a reward prediction error, or a state-value representation from the user’s neural activity, which the RL-BCI agent could use to update its BCI-decoder. In order to understand the multifaceted effects of reward on M1 activity, we investigated how neural spiking, oscillatory activities and their functional interactions are modulated by conditioned stimuli related reward expectation. To do so, local field potentials (LFPs) and single-unit/multiunit activities were recorded simultaneously and bilaterally from M1 cortices while four non-human primates performed cued center-out reaching or grip force tasks either manually using their right arm/hand or observed passively. We found that reward expectation influenced the strength of alpha (8-14 Hz) power, alpha-gamma comodulation, alpha spike-field coherence, and firing rates in general in M1. Furthermore, we found that an increase in alpha-band power was correlated with a decrease in neural spiking activity, that firing rates were highest at the trough of the alpha-band cycle and lowest at the peak of its cycle. These findings imply that alpha oscillations modulated by reward expectation have an influence on spike firing rate and spike timing during both reaching and grasping tasks in M1. These LFP, spike, and spike-field interactions could be used to follow the M1 neural state in order to enhance BCI decoding (An et al., 2018; Zhao et al., 2018).
Significance Statement
Knowing the subjective value of performed or observed actions is valuable feedback that could be used to improve the performance of an autonomously updating brain-computer interface (BCI). Reward-related information in the primary motor cortex (M1) may be crucial for more stable and robust BCI decoding (Zhao et al., 2018). Here, we present how expectation of reward during motor tasks, or simple observation, is represented by increased spike firing rates in conjunction with decreased alpha (8-14 Hz) oscillatory power, alpha-gamma comodulation, and alpha spike-field coherence, as compared to nonrewarding trials. Moreover, a phasic relation between alpha oscillations and firing rates was observed where firing rates were found to be lowest and highest at the peak and trough of alpha oscillations, respectively.