Summary: Applying a nonlinear signal processing method to experimental data, a new study reveals a connection between motor behavior and brain activity. The findings could help with the development of new brain-computer interfaces and artificial intelligence technologies.
Source: American Institute of Physics
Motor-related brain activity, particularly its accurate detection, quantification and classification capabilities, is of great interest to researchers. They are searching for a better way to help patients with cognitive or motor impairments or to improve neurorehabilitation for patients with nervous system injuries.
There is a close relationship between motor and cognitive activity of the human brain, and suppression of the specific rhythmic activity of neurons within the sensorimotor cortex of the brain — known as the mu-rhythm (8 to 14 hertz) — is a biological marker of motor-related brain activity. Studies indicate this feature of motor-related brain activity suffers from intra- and inter-subject variability when using traditional methods to explore it, such as time-frequency analysis, spatial filtering and machine learning.
In the journal Chaos, from AIP Publishing, Nikita Frolov and colleagues at Innopolis University in Russia are approaching the problem from a different angle to search for a more robust feature of brain activity associated with accomplishing motor tasks.
“We put forward the hypothesis that suppression of mu-oscillations will cause a reduction of measured brain activity signals and, consequently, reflects the simplification of underlying neuronal dynamics,” said Frolov. “To address this issue, we applied recurrence quantification analysis, which is a powerful toolbox, to explore systems complexity through the analysis of its time series.”
The group’s work confirms, for the first time, the neuronal dynamics within the sensorimotor brain area underlying the motor functions of the human brain can be simplified.
“We demonstrated this using the RQA toolbox, which is fundamentally different from traditional methods for quantification of motor-related brain activity,” Frolov said. “We also demonstrated that RQA measures of complexity are well suited to detect and classify motor tasks.”
These results show potential for developing efficient methods for classifying brain states.
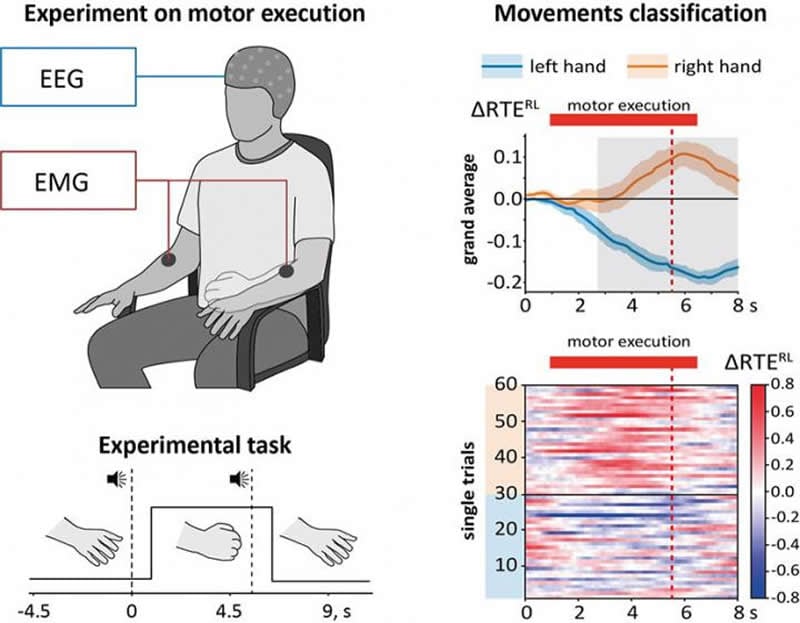
“By accurately introducing a state space, you can consider any natural system as a dynamical system. For the human brain, you can create state space by taking the measured signals of its activity as the state variables,” Frolov said.
“In our study, we consider state space formed by the set of electroencephalograms (signals of electrical activity within the brain’s cortical regions) recorded within the motor cortex. This allows us to introduce the ‘state’ of the cortical region of interest and to consider it as a dynamical system.”
One of the applications of the group’s work is “implementation of RQA-based analysis of electroencephalograms as a computational core of the brain-computer interfaces for online detection, quantification, and training of brain motor functions,” Frolov said.
“This is relevant not only for the development of closed-loop interfaces for motor skills training during neurorehabilitation but also for the diagnosis of cognitive and motor impairments as well as age-related changes.”
Source:
American Institute of Physics
Media Contacts:
Larry Frum – American Institute of Physics
Image Source:
The image is credited to Nikita Frolov/Innopolis University.
Original Research: Open access
“Motor execution reduces EEG signals complexity: recurrence quantification analysis study”. Elena N. Pitsik, Nikita S. Frolov, Kai Hauke Kraemer, Vadim V. Grubov, Vladimir A. Maksiemenko, Juergen Kurths and Alexander E. Hramov.
Chaos doi:10.1063/1.5136246.
Abstract
Motor execution reduces EEG signals complexity: recurrence quantification analysis study
The development of new approaches to detect motor-related brain activity is key in many aspects of science, especially in brain–computer interface applications. Even though some well-known features of motor-related electroencephalograms have been revealed using traditionally applied methods, they still lack a robust classification of motor-related patterns. Here, we introduce new features of motor-related brain activity and uncover hidden mechanisms of the underlying neuronal dynamics by considering event-related desynchronization (ERD) of μ-rhythm in the sensorimotor cortex, i.e., tracking the decrease of the power spectral density in the corresponding frequency band. We hypothesize that motor-related ERD is associated with the suppression of random fluctuations of μ-band neuronal activity. This is due to the lowering of the number of active neuronal populations involved in the corresponding oscillation mode. In this case, we expect more regular dynamics and a decrease in complexity of the EEG signal recorded over the sensorimotor cortex. In order to support this, we apply measures of signal complexity by means of recurrence quantification analysis (RQA). In particular, we demonstrate that certain RQA quantifiers are very useful to detect the moment of movement onset and, therefore, are able to classify the laterality of executed movements.