Summary: Researchers developed AI software that rapidly analyzes bone density scans to predict a person’s future risk of health conditions, such as heart attacks, stroke, falls, fractures, and dementia.
The software looks for signs of abdominal aortic calcification (AAC), a predictor of these conditions. While human experts typically take 5-15 minutes to analyze each scan, the AI can process 60,000 images in one day.
The algorithm accurately matches expert conclusions 80% of the time, representing a significant advancement in early detection and disease monitoring.
Key Facts:
- The AI software is capable of analyzing bone density scans to detect signs of abdominal aortic calcification (AAC), a predictor of future health conditions.
- The AI can process 60,000 images in one day, compared to a human expert who typically takes 5-15 minutes per image.
- The algorithm matches the conclusions of human experts 80% of the time, and only 3% of high AAC levels were incorrectly diagnosed as low by the software.
Source: Edith Cowan University
Thanks to artificial intelligence, we will soon be able to predict our risk of developing serious health conditions later in life, at the press of a button.
Abdominal aortic calcification, or AAC, is a calcification which can build up within the walls of the abdominal aorta and predicts your risk of developing cardiovascular disease events such as heart attacks and stroke.
It also predicts your risk of falls, fractures and late-life dementia.
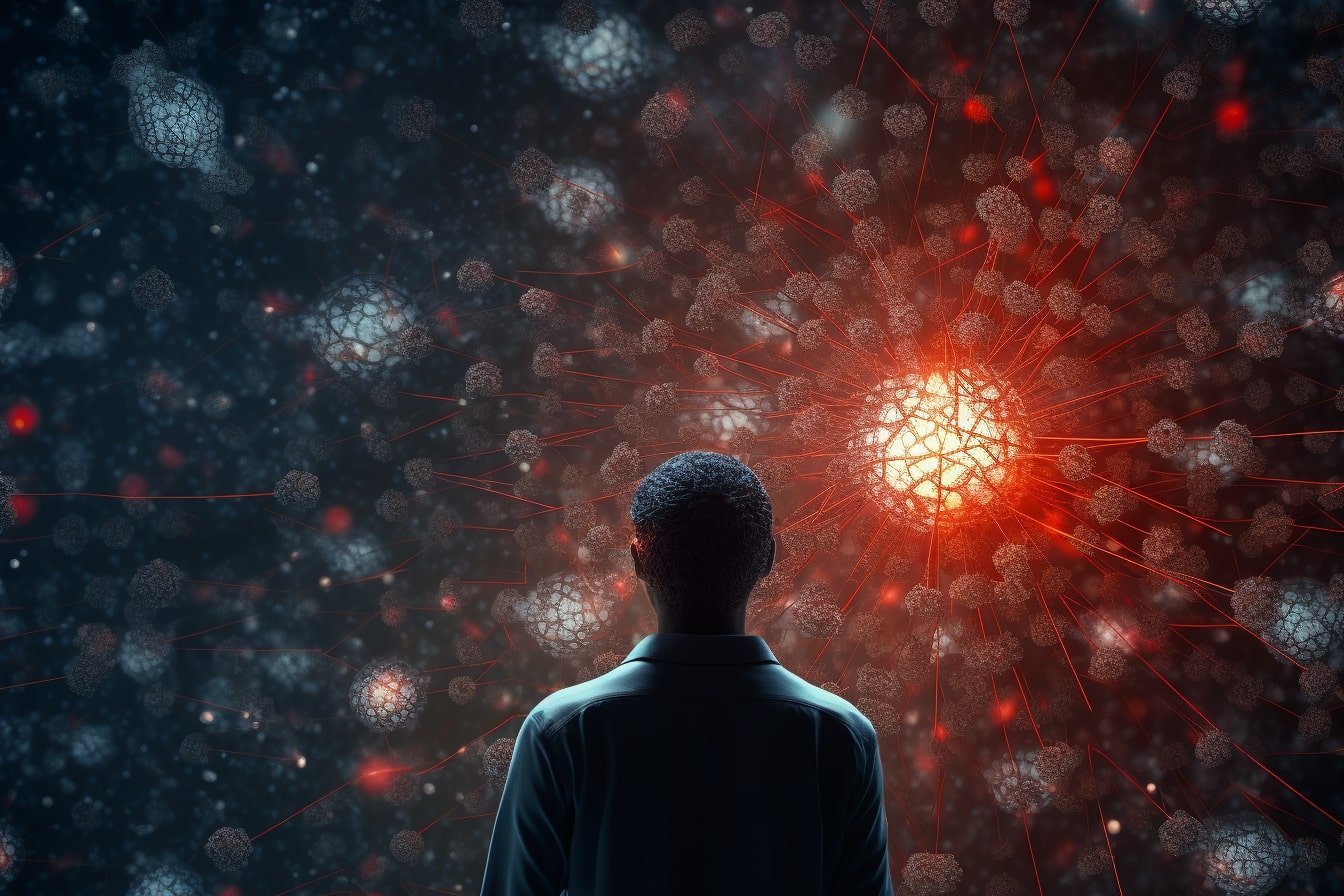
Conveniently, common bone density machine scans used to detect osteoporosis can also detect AAC.
However, highly trained expert readers are needed to analyze the images, a process which can take 5-15 minutes per image.
But researchers from Edith Cowan University’s (ECU) School of Science and School of Medical and Health Sciences have collaborated to develop software which can analyze scans much, much faster: roughly 60,000 images in a single day.
Researcher and Heart Foundation Future Leader Fellow Associate Professor Joshua Lewis said this significant boost in efficiency will be crucial for the widespread use of AAC in research and helping people avoid developing health problems later in life.
“Since these images and automated scores can be rapidly and easily acquired at the time of bone density testing, this may lead to new approaches in the future for early cardiovascular disease detection and disease monitoring during routine clinical practice,” he said.
Saving a LOT of time
The results were from an international collaboration between ECU, the University of WA, University of Minnesota, Southampton, University of Manitoba, Marcus Institute for Aging Research, and Hebrew SeniorLife Harvard Medical School. Truly a multidisciplinary global effort.
Though it’s not the first algorithm developed to assess AAC from these images, the study is the biggest of its kind, was based on the most commonly used bone density machine models, and is the first to be tested in a real-world setting using images taken as part of routine bone density testing.
It saw more than 5000 images analyzed by experts and the team’s software.
After comparing the results, the expert and software arrived at the same conclusion for the extent of AAC (low, moderate or high) 80 percent of the time – an impressive figure given it was the first version of the software.
ARTICLE IMAGE HERE PLS
Importantly, only 3 percent of people deemed to have high AAC levels were incorrectly diagnosed to have low levels by the software.
“This is notable as these are the individuals with the greatest extent of disease and highest risk of fatal and nonfatal cardiovascular events and all-cause mortality,” Professor Lewis said.
“Whilst there is still to work to do to improve the software’s accuracy compared to human readings, these results are from our version 1.0 algorithm, and we already have improved the results substantially with our more recent versions.
“Automated assessment of the presence and extent of AAC with similar accuracies to imaging specialists provides the possibility of large-scale screening for cardiovascular disease and other conditions – even before someone has any symptoms.”
“This will allow people at risk to make the necessary lifestyle changes far earlier and put them in a better place to be healthier in their later years.”
Funding: The Heart Foundation provided funding for the project, thanks to Professor Lewis’ 2019 Future Leadership Fellowship providing support for research over a three-year period.
About this artificial intelligence and health research news
Author: Sam Jeremic
Source: Edith Cowan University
Contact: Sam Jeremic – Edith Cowan University
Image: The image is credited to Neuroscience News
Original Research: Open access.
“Machine learning for abdominal aortic calcification assessment from bone density machine-derived lateral spine images” by Joshua Lewis et al. eBioMedicine
Abstract
Machine learning for abdominal aortic calcification assessment from bone density machine-derived lateral spine images
Background
Lateral spine images for vertebral fracture assessment can be easily obtained on modern bone density machines. Abdominal aortic calcification (AAC) can be scored on these images by trained imaging specialists to assess cardiovascular disease risk. However, this process is laborious and requires careful training.
Methods
Training and testing of model performance of the convolutional neural network (CNN) algorithm for automated AAC-24 scoring utilised 5012 lateral spine images (2 manufacturers, 4 models of bone density machines), with trained imaging specialist AAC scores. Validation occurred in a registry-based cohort study of 8565 older men and women with images captured as part of routine clinical practice for fracture risk assessment. Cox proportional hazards models were used to estimate the association between machine-learning AAC (ML-AAC-24) scores with future incident Major Adverse Cardiovascular Events (MACE) that including death, hospitalised acute myocardial infarction or ischemic cerebrovascular disease ascertained from linked healthcare data.
Findings
The average intraclass correlation coefficient between imaging specialist and ML-AAC-24 scores for 5012 images was 0.84 (95% CI 0.83, 0.84) with classification accuracy of 80% for established AAC groups. During a mean follow-up 4 years in the registry-based cohort, MACE outcomes were reported in 1177 people (13.7%). With increasing ML-AAC-24 scores there was an increasing proportion of people with MACE (low 7.9%, moderate 14.5%, high 21.2%), as well as individual MACE components (all p-trend <0.001). After multivariable adjustment, moderate and high ML-AAC-24 groups remained significantly associated with MACE (HR 1.54, 95% CI 1.31–1.80 & HR 2.06, 95% CI 1.75–2.42, respectively), compared to those with low ML-AAC-24.
Interpretation
The ML-AAC-24 scores had substantial levels of agreement with trained imaging specialists, and was associated with a substantial gradient of risk for cardiovascular events in a real-world setting. This approach could be readily implemented into these clinical settings to improve identification of people at high CVD risk.
Funding
The study was supported by a National Health and Medical Research Council of Australia Ideas grant and the Rady Innovation Fund, Rady Faculty of Health Sciences, University of Manitoba.