Summary: A new study reveals that artificial intelligence systems are more susceptible to adversarial attacks than previously believed, making them vulnerable to manipulation that can lead to incorrect decisions.
Researchers found that adversarial vulnerabilities are widespread in AI deep neural networks, raising concerns about their use in critical applications. To assess these vulnerabilities, the team developed QuadAttacK, a software that can test neural networks for susceptibility to adversarial attacks.
The findings highlight the need to enhance AI robustness against such attacks, particularly in applications with potential human life implications.
Key Facts:
- Adversarial attacks involve manipulating data to confuse AI systems, potentially leading to erroneous outcomes.
- QuadAttacK, developed by the researchers, can test deep neural networks for susceptibility to adversarial vulnerabilities.
- Widespread vulnerabilities were found in various widely-used deep neural networks, emphasizing the need for increased AI robustness.
Source: North Carolina State University
Artificial intelligence tools hold promise for applications ranging from autonomous vehicles to the interpretation of medical images. However, a new study finds these AI tools are more vulnerable than previously thought to targeted attacks that effectively force AI systems to make bad decisions.
At issue are so-called “adversarial attacks,” in which someone manipulates the data being fed into an AI system in order to confuse it. For example, someone might know that putting a specific type of sticker at a specific spot on a stop sign could effectively make the stop sign invisible to an AI system. Or a hacker could install code on an X-ray machine that alters the image data in a way that causes an AI system to make inaccurate diagnoses.
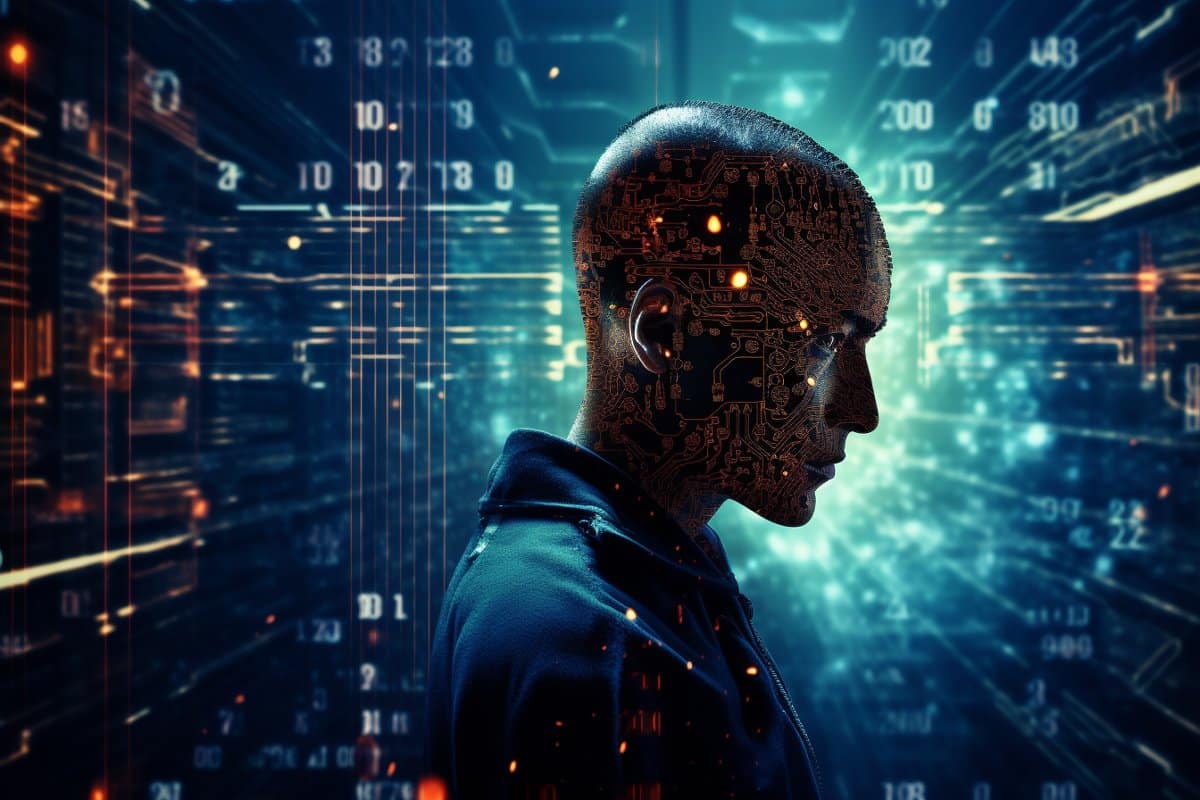
“For the most part, you can make all sorts of changes to a stop sign, and an AI that has been trained to identify stop signs will still know it’s a stop sign,” says Tianfu Wu, co-author of a paper on the new work and an associate professor of electrical and computer engineering at North Carolina State University.
“However, if the AI has a vulnerability, and an attacker knows the vulnerability, the attacker could take advantage of the vulnerability and cause an accident.”
The new study from Wu and his collaborators focused on determining how common these sorts of adversarial vulnerabilities are in AI deep neural networks. They found that the vulnerabilities are much more common than previously thought.
“What’s more, we found that attackers can take advantage of these vulnerabilities to force the AI to interpret the data to be whatever they want,” Wu says.
“Using the stop sign example, you could make the AI system think the stop sign is a mailbox, or a speed limit sign, or a green light, and so on, simply by using slightly different stickers – or whatever the vulnerability is.
“This is incredibly important, because if an AI system is not robust against these sorts of attacks, you don’t want to put the system into practical use – particularly for applications that can affect human lives.”
To test the vulnerability of deep neural networks to these adversarial attacks, the researchers developed a piece of software called QuadAttacK. The software can be used to test any deep neural network for adversarial vulnerabilities.
“Basically, if you have a trained AI system, and you test it with clean data, the AI system will behave as predicted. QuadAttacK watches these operations and learns how the AI is making decisions related to the data. This allows QuadAttacK to determine how the data could be manipulated to fool the AI.
QuadAttacK then begins sending manipulated data to the AI system to see how the AI responds. If QuadAttacK has identified a vulnerability it can quickly make the AI see whatever QuadAttacK wants it to see.”
In proof-of-concept testing, the researchers used QuadAttacK to test four deep neural networks: two convolutional neural networks (ResNet-50 and DenseNet-121) and two vision transformers (ViT-B and DEiT-S). These four networks were chosen because they are in widespread use in AI systems around the world.
“We were surprised to find that all four of these networks were very vulnerable to adversarial attacks,” Wu says. “We were particularly surprised at the extent to which we could fine-tune the attacks to make the networks see what we wanted them to see.”
The research team has made QuadAttacK publicly available, so that the research community can use it themselves to test neural networks for vulnerabilities. The program can be found here: https://thomaspaniagua.github.io/quadattack_web/.
“Now that we can better identify these vulnerabilities, the next step is to find ways to minimize those vulnerabilities,” Wu says. “We already have some potential solutions – but the results of that work are still forthcoming.”
The paper, “QuadAttacK: A Quadratic Programming Approach to Learning Ordered Top-K Adversarial Attacks,” will be presented Dec. 16 at the Thirty-seventh Conference on Neural Information Processing Systems (NeurIPS 2023), which is being held in New Orleans, La. First author of the paper is Thomas Paniagua, a Ph.D. student at NC State. The paper was co-authored by Ryan Grainger, a Ph.D. student at NC State.
Funding: The work was done with support from the U.S. Army Research Office, under grants W911NF1810295 and W911NF2210010; and from the National Science Foundation, under grants 1909644, 2024688 and 2013451.
About this artificial intelligence research news
Author: Matt Shipman
Source: North Carolina State University
Contact: Matt Shipman – North Carolina State University
Image: The image is credited to Neuroscience News
Original Research: The findings will be presented at the Thirty-seventh Conference on Neural Information Processing Systems (NeurIPS)