Summary: When convolutional neural networks are trained under experimental conditions, they are deceived by the brightness and color of a visual image in similar ways to the human visual system.
Source: UPF Barcelona
A convolutional neural network is a type of artificial neural network in which the neurons are organized into receptive fields in a very similar way to neurons in the visual cortex of a biological brain.
Today, convolutional neural networks (CNNs) are found in a variety of autonomous systems (for example, face detection and recognition, autonomous vehicles, etc.). This type of network is highly effective in many artificial vision tasks, such as in image segmentation and classification, along with many other applications.
Convolutional networks were inspired by the behaviour of the human visual system, particularly its basic structure formed by the concatenation of compound modules comprising a linear operation followed by a non-linear operation. A study published in the advanced online edition of the journal Vision Research examines the phenomenon of visual illusions in convolutional networks compared to their effect on human vision.
“Because of this connection of CNNs with our visual system, in this paper we wanted to see if convolutional networks suffer from similar problems to our visual system. Hence, we focused on visual illusions. Visual illusions are images that our brain perceives differently from how they actually are”, explains Gómez Vila, first author of the study.
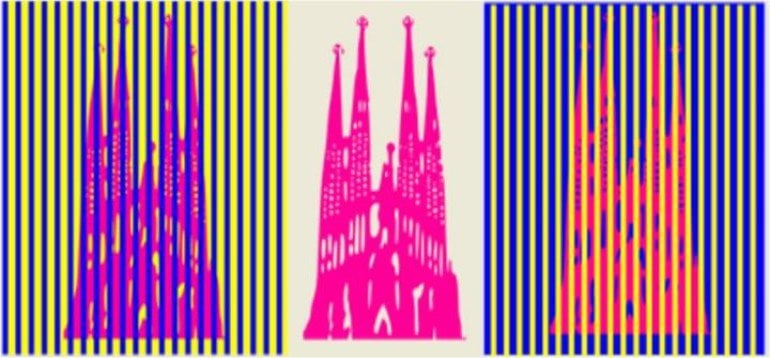
In their study, the authors trained CNNs for simple tasks also performed by human vision, such as denoising and deblurring. What they observed is that these CNNs trained under these experimental conditions are also “deceived” by brightness and colour visual illusions in the same way that visual illusions deceive humans.
Furthermore, as Gómez Villa explains, “for our work we also analyse when such illusions cause responses in the network that are not as physically expected, but neither do they match with human perception”, that is to say, cases in which CNNs obtain a different optical illusion than the illusion that humans would perceive.
The results of this study are consistent with the long-standing hypothesis that considers low-level visual illusions as a by-product of the optimization to natural environments (that a human sees in their everyday). Meanwhile, these results highlight the limitations and differences between the human visual system and CNNs artificial neural networks.
About this artificial intelligence research news
Source: UPF Barcelona
Contact: Núria Perez – UPF Barcelona
Image: The image is credited to the researchers
Original Research: Closed access.
“Color illusions also deceive CNNs for low-level vision tasks: Analysis and implications” by Alexander Gómez Vila, Adrian Martín, Javier Vázquez-Corral, Marcelo Bertalmío, Jesús Malo . Vision Research
Abstract
Color illusions also deceive CNNs for low-level vision tasks: Analysis and implications
The study of visual illusions has proven to be a very useful approach in vision science. In this work we start by showing that, while convolutional neural networks (CNNs) trained for low-level visual tasks in natural images may be deceived by brightness and color illusions, some network illusions can be inconsistent with the perception of humans. Next, we analyze where these similarities and differences may come from. On one hand, the proposed linear eigenanalysis explains the overall similarities: in simple CNNs trained for tasks like denoising or deblurring, the linear version of the network has center-surround receptive fields, and global transfer functions are very similar to the human achromatic and chromatic contrast sensitivity functions in human-like opponent color spaces. These similarities are consistent with the long-standing hypothesis that considers low-level visual illusions as a by-product of the optimization to natural environments. Specifically, here human-like features emerge from error minimization. On the other hand, the observed differences must be due to the behavior of the human visual system not explained by the linear approximation. However, our study also shows that more ‘flexible’ network architectures, with more layers and a higher degree of nonlinearity, may actually have a worse capability of reproducing visual illusions. This implies, in line with other works in the vision science literature, a word of caution on using CNNs to study human vision: on top of the intrinsic limitations of the L + NL formulation of artificial networks to model vision, the nonlinear behavior of flexible architectures may easily be markedly different from that of the visual system.