Summary: New AI technology can detect a patient’s stroke depression type, and improve treatment options.
Source: Hiroshima University
An AI developed by Japanese researchers might soon help stroke survivors get the right treatment by detecting a patient’s post-stroke depression (PSD) type, a frequently seen but often overlooked neuropsychiatric manifestation after a stroke that could impair functional recovery.
The AI was developed by Hiroshima University (HU) researchers using a probabilistic artificial neural network called log-linearized Gaussian mixture network. The neural network was trained to distinguish between depression, apathy, or anxiety based on 36 evaluation indices obtained from functional, physical, and cognitive tests on 274 patients.
Details about their research that analyzed the relationship between PSD and activities of daily living independence, degree of paralysis, stress awareness, and higher brain function using machine learning are published in Scientific Reports.
Early PSD detection
The researchers said each PSD type might have different underlying neuroanatomic mechanisms which could have a distinct impact on a patient’s functional recovery. And its early detection is crucial to give the appropriate treatment needed by the patient.
“Depression is a highly comorbid neuropsychiatric symptom during the acute and subacute phase after a stroke and has been reported to negatively influence functional and cognitive recovery. Thus, early diagnosis and intervention are crucial for post-stroke depression,” study author Seiji Hama, a research associate at HU’s Graduate School of Biomedical and Health Science, said.
“However, PSD is multifactorial, and associated neurological symptoms may hinder the detection process. This study is the first step in aiming to accurately diagnose PSD using data obtained in routine practice without any special equipment.”
The researchers tested the AI’s diagnostic accuracy through the receiver operating characteristic curve which visually evaluates the performance of a machine learning algorithm by giving it an area under the curve (AUC) score. An AUC score of 1.0 means a perfect performance. The PSD detection AI scored above 0.85.
Stress threshold hypothesis
Various post-stroke physical disorders, cognitive dysfunction, and mood disorders associated with stress responses are intricately intertwined, making it difficult to understand the cause of PSD and, therefore, making its diagnosis challenging.
It is uncertain whether PSD occurs as part of the mourning process due to the physical impairments after a stroke or if it is brought about by biological factors associated with brain damage.
The study results, however, suggested that reduced stress adaptability due to stroke-induced brain lesions is behind PSD.
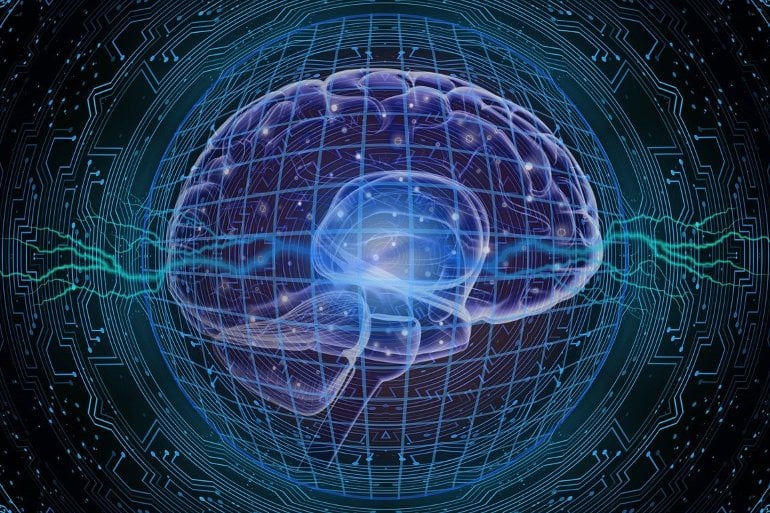
“One of the traditional hypotheses on the PSD mechanism was ‘threshold hypothesis,’ consistent with many previous reports demonstrating the association between the accumulation of lacunar infarcts within the basal ganglia, thalamus, and deep white matter and PSD,” they said in their study.
Hama said they intend to conduct detailed analysis using MRI images to further clarify the origins of PSD and improve techniques to diagnose it in hopes of applying the technology to wearable devices. The researchers expect patient rehabilitation would be improved through the early diagnosis and treatment of PSD.
“If this diagnostic technique of PSD becomes possible to test with a wearable device, it will be possible to use it in the local community. By combining it with the test for cognitive function, we would like to verify its application to the preventive effect of strokes,” he said.
About this AI research news
NeuroscienceNews would like to thank Mikas Matsuzawa for submitting this AI research news.
Source: Hiroshima University
Contact: Mikas Matsuzawa – Hiroshima University
Image: The image is in the public domain
Original Research: Open access.
“Relationships between motor and cognitive functions and subsequent post-stroke mood disorders revealed by machine learning analysis” by Seiji Hama, Kazumasa Yoshimura, Akiko Yanagawa, Koji Shimonaga, Akira Furui, Zu Soh, Shinya Nishino, Harutoyo Hirano, Shigeto Yamawaki & Toshio Tsuji. Scientific Reports
Abstract
Relationships between motor and cognitive functions and subsequent post-stroke mood disorders revealed by machine learning analysis
Mood disorders (e.g. depression, apathy, and anxiety) are often observed in stroke patients, exhibiting a negative impact on functional recovery associated with various physical disorders and cognitive dysfunction. Consequently, post-stroke symptoms are complex and difficult to understand.
In this study, we aimed to clarify the cross-sectional relationship between mood disorders and motor/cognitive functions in stroke patients. An artificial neural network architecture was devised to predict three types of mood disorders from 36 evaluation indices obtained from functional, physical, and cognitive tests on 274 patients.
The relationship between mood disorders and motor/cognitive functions were comprehensively analysed by performing input dimensionality reduction for the neural network. The receiver operating characteristic curve from the prediction exhibited a moderate to high area under the curve above 0.85. Moreover, the input dimensionality reduction retrieved the evaluation indices that are more strongly related to mood disorders.
The analysis results suggest a stress threshold hypothesis, in which stroke-induced lesions promote stress vulnerability and may trigger mood disorders.