Summary: Artificial neural networks modeled on human brain connectivity can effectively perform complex cognitive tasks.
Source: McGill University
A new study shows that artificial intelligence networks based on human brain connectivity can perform cognitive tasks efficiently.
By examining MRI data from a large Open Science repository, researchers reconstructed a brain connectivity pattern, and applied it to an artificial neural network (ANN). An ANN is a computing system consisting of multiple input and output units, much like the biological brain.
A team of researchers from The Neuro (Montreal Neurological Institute-Hospital) and the Quebec Artificial Intelligence Institute trained the ANN to perform a cognitive memory task and observed how it worked to complete the assignment.
This is a unique approach in two ways. Previous work on brain connectivity, also known as connectomics, focused on describing brain organization, without looking at how it actually performs computations and functions. Secondly, traditional ANNs have arbitrary structures that do not reflect how real brain networks are organized.
By integrating brain connectomics into the construction of ANN architectures, researchers hoped to both learn how the wiring of the brain supports specific cognitive skills, and to derive novel design principles for artificial networks.
They found that ANNs with human brain connectivity, known as neuromorphic neural networks, performed cognitive memory tasks more flexibly and efficiently than other benchmark architectures.
The neuromorphic neural networks were able to use the same underlying architecture to support a wide range of learning capacities across multiple contexts.
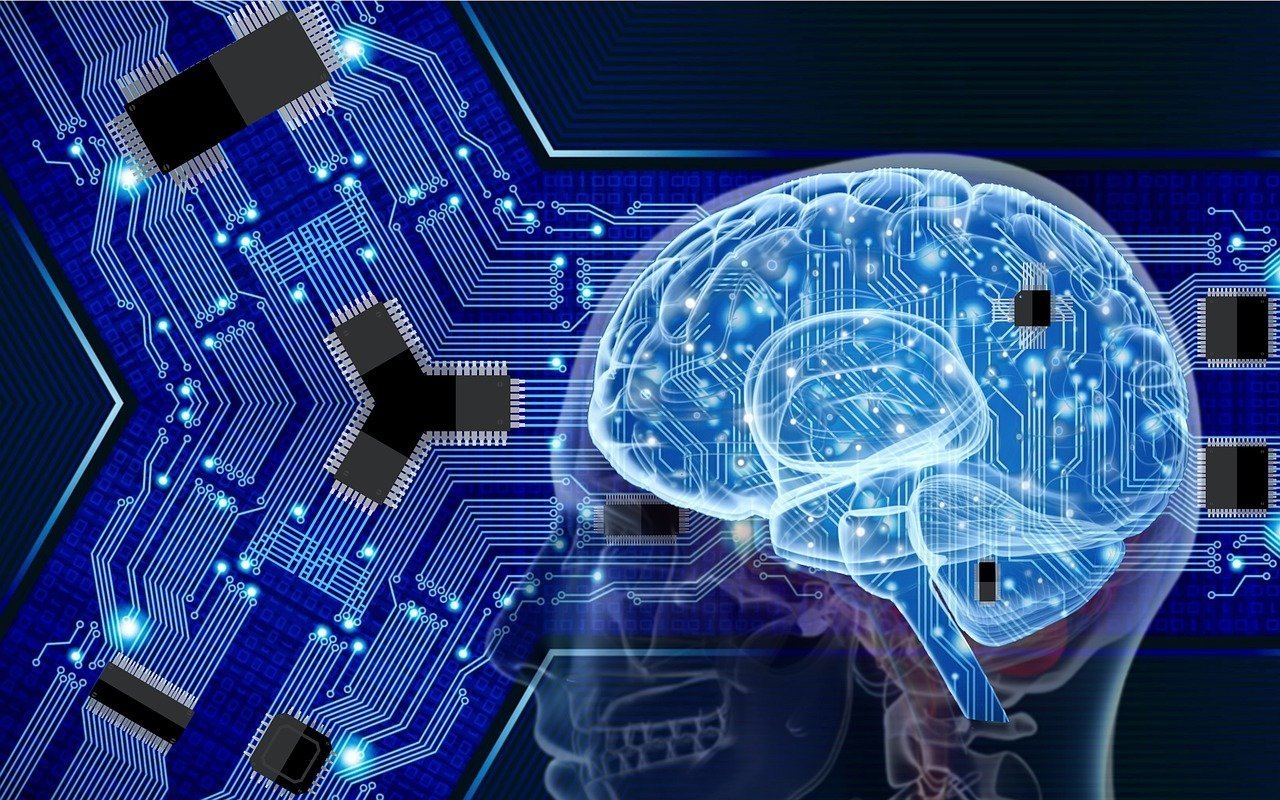
“The project unifies two vibrant and fast-paced scientific disciplines,” says Bratislav Misic, a researcher at The Neuro and the paper’s senior author. “Neuroscience and AI share common roots, but have recently diverged. Using artificial networks will help us to understand how brain structure supports brain function. In turn, using empirical data to make neural networks will reveal design principles for building better AI. So, the two will help inform each other and enrich our understanding of the brain.”
Funding: This study, published in the journal Nature Machine Intelligence on Aug. 9, 2021, was funded with the help of the Canada First Research Excellence Fund, awarded to McGill University for the Healthy Brains, Healthy Lives initiative, the Natural Sciences and Engineering Research Council of Canada, Fonds de Recherche du Quebec – Santé, Canadian Institute for Advanced Research, Canada Research Chairs, Fonds de Recherche du Quebec – Nature et Technologies, and Centre UNIQUE (Union of Neuroscience and Artificial Intelligence).
About this artificial intelligence research news
Source: McGill University
Contact: Press Office – McGill University
Image: The image is in the public domain
Original Research: Closed access.
“Learning function from structure in neuromorphic networks” by Laura E. Suárez, Blake A. Richards, Guillaume Lajoie & Bratislav Misic. Nature
Abstract
Learning function from structure in neuromorphic networks
The connection patterns of neural circuits in the brain form a complex network. Collective signalling within the network manifests as patterned neural activity and is thought to support human cognition and adaptive behaviour.
Recent technological advances permit macroscale reconstructions of biological brain networks. These maps, termed connectomes, display multiple non-random architectural features, including heavy-tailed degree distributions, segregated communities and a densely interconnected core. Yet, how computation and functional specialization emerge from network architecture remains unknown.
Here we reconstruct human brain connectomes using in vivo diffusion-weighted imaging and use reservoir computing to implement connectomes as artificial neural networks.
We then train these neuromorphic networks to learn a memory-encoding task. We show that biologically realistic neural architectures perform best when they display critical dynamics. We find that performance is driven by network topology and that the modular organization of intrinsic networks is computationally relevant.
We observe a prominent interaction between network structure and dynamics throughout, such that the same underlying architecture can support a wide range of memory capacity values as well as different functions (encoding or decoding), depending on the dynamical regime the network is in.
This work opens new opportunities to discover how the network organization of the brain optimizes cognitive capacity.